OpenAI Blog: AI and Compute
Artificial Intelligence (AI) has made tremendous strides in recent years, thanks in large part to the increasing amount of compute power used to train models. In this blog post, we explore the relationship between AI and compute, discussing their intertwined growth and the implications for the future of AI development.
Key Takeaways:
- Increase in compute power has played a significant role in advancing AI capabilities.
- AI progress exhibits exponential growth as compute power doubles every 3.5 months.
- The AI research community has quickly adopted new hardware to drive advancements.
- Efficiency gains in algorithms contribute to improved AI performance.
AI models have become more powerful and sophisticated over the years due to advancements in compute power. **Analyzing large amounts of data** and training complex deep learning models require substantial computational resources. The **exponential growth of compute** has enabled AI to make significant breakthroughs, leading to advancements in various domains such as image recognition, natural language processing, and reinforcement learning.
As compute power has increased, AI researchers have embraced the opportunity to leverage this additional capacity. In a relatively short time frame, **the compute used in AI experiments has doubled** approximately every 3.5 months. This rapid growth in computing resources has allowed researchers to tackle more complex problems and train larger models more efficiently.
Hardware advancements have been a driving force behind the growth of AI. GPUs (Graphics Processing Units), initially designed for rendering graphics, have become the workhorses of AI training due to their parallel computing capabilities. Researchers have also embraced specialized hardware such as **Tensor Processing Units (TPUs)**, designed by Google specifically for machine learning purposes. These hardware advancements have revolutionized AI training, making it more accessible and efficient.
Year | Computational Power (FLOPS) |
---|---|
2012 | 10^14 |
2018 | 10^19 |
2020 | 10^23 |
Although compute power has played a vital role in AI development, it is important to note that **improvements in algorithms and model efficiency have also contributed** to advancements in AI capabilities. Researchers constantly strive to improve the efficiency and effectiveness of AI models, allowing them to achieve better performance with the available compute resources.
AI and compute have formed a symbiotic relationship, where each fuels the progress of the other. As compute power continues to grow, it enables more complex and sophisticated AI models, propelling AI research further. Conversely, AI research drives the demand for more powerful compute resources, pushing hardware innovation forward.
Impact of AI Advancements
- Improved accuracy and performance in various AI applications.
- Enablement of more complex and sophisticated AI models.
- Acceleration of research and development in AI-driven domains.
- Potential for AI to solve increasingly complex real-world problems.
AI advancements driven by compute power have had a profound impact across industries. Improved accuracy and performance in AI applications have transformed fields such as healthcare, finance, and autonomous vehicles. With the enablement of more complex and sophisticated AI models, we are witnessing breakthroughs in areas like natural language understanding, game playing, and image generation. The acceleration of research and development in AI-driven domains brings us closer to solving complex real-world problems in areas such as climate change, drug discovery, and safe autonomous systems.
Year | Compute Growth (since 2012) |
---|---|
2012 | 1x |
2018 | 300,000x |
2020 | 3,000,000x |
Looking ahead, the interplay between AI and compute is expected to lead to even more remarkable breakthroughs. As we witness unprecedented growth in computing power, the vast potential of AI to transform industries and solve complex problems becomes increasingly evident. The future of AI holds immense promise, with continued advancements in compute power and algorithms driving the next wave of breakthroughs.
Conclusion
The growth of AI and compute power are deeply intertwined, with advancements in one propelling the progress of the other. Increased compute resources have enabled the rapid evolution of AI models and capabilities. As hardware innovation continues and algorithms become more efficient, we can anticipate further advancements in AI technology and its widespread impact on various domains.
AI Advancements | Compute Power Contribution |
---|---|
Improved model performance and accuracy | Significant |
Complex and sophisticated AI models | Crucial |
Acceleration of research and development | Indispensable |
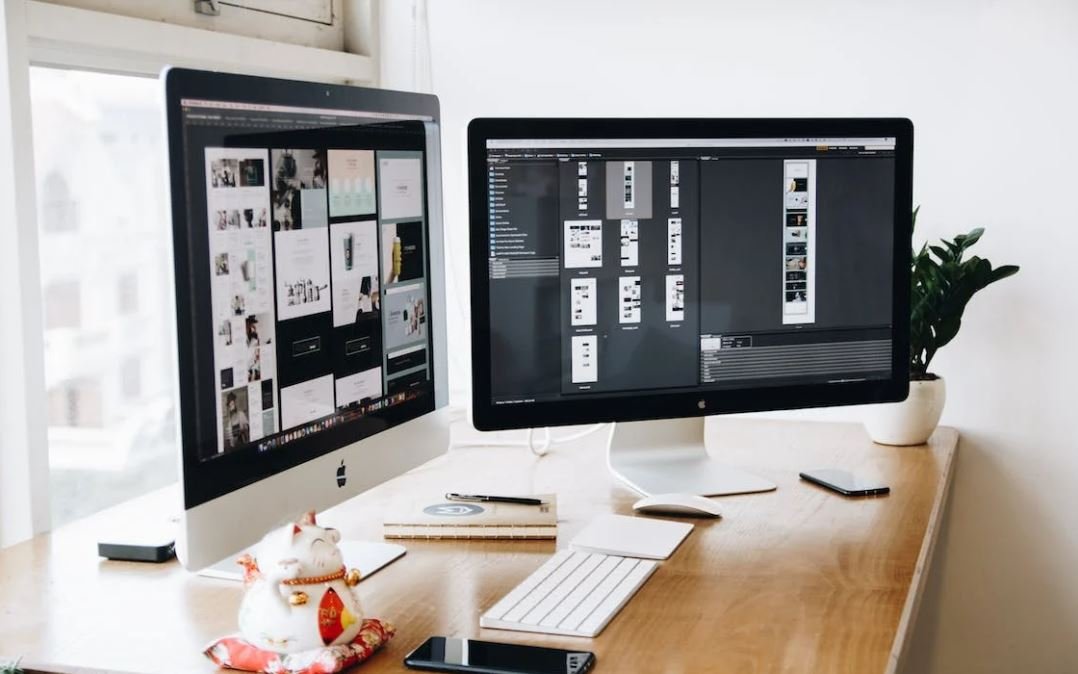
Common Misconceptions
Misconception 1: AI is just about the amount of compute power
One common misconception about AI is that its performance is solely dependent on the amount of compute power available. While compute power does play a crucial role, there are various other factors that contribute to the success of AI systems.
- Algorithmic advancements are equally important for AI development.
- Data quality and diversity can significantly impact AI performance.
- Model architecture and design choices also influence AI capabilities.
Misconception 2: AI will replace human intelligence completely
Another misconception is the belief that AI technology will eventually surpass human intelligence and render human workers obsolete. However, AI systems are designed to complement and assist human capabilities rather than replace them entirely.
- AI can automate repetitive tasks, enabling humans to focus on complex problem-solving.
- Human creativity, emotional intelligence, and critical thinking still cannot be replicated by AI.
- In many cases, AI systems require human supervision and intervention for decision-making.
Misconception 3: AI is inherently biased and discriminatory
There is often a misconception that AI is inherently biased and discriminatory due to the data it is trained on. While it is true that biased data can lead to biased AI, this does not mean AI itself is inherently discriminatory.
- Biases in AI often originate from the biases present in the training data provided to the system.
- Bias detection and mitigation techniques can be implemented to address and minimize bias in AI systems.
- Ethical considerations and diverse representation in AI development can help prevent biased outcomes.
Misconception 4: AI will bring about widespread unemployment
Many people fear that AI advancements will lead to widespread unemployment, with machines taking over jobs across various industries. However, historical evidence suggests that AI technology has the potential to create more jobs than it displaces.
- AI technology can create new employment opportunities in industries such as data science, AI research, and automation engineering.
- AI can enhance productivity and efficiency, leading to economic growth and job creation.
- Workers can transition to new roles that require human skills AI cannot replicate.
Misconception 5: AI is only beneficial for large organizations with vast resources
Some people believe that AI is only accessible and beneficial to large organizations with extensive resources and budgets. However, AI technology is increasingly becoming democratized, allowing businesses and individuals of all sizes to leverage its benefits.
- Cloud-based AI services enable businesses to access AI capabilities without heavy infrastructure investments.
- Open-source AI frameworks and libraries make it easier for developers to build AI applications with minimal costs.
- AI tools and platforms are becoming more user-friendly, allowing individuals to experiment and innovate with AI technology.
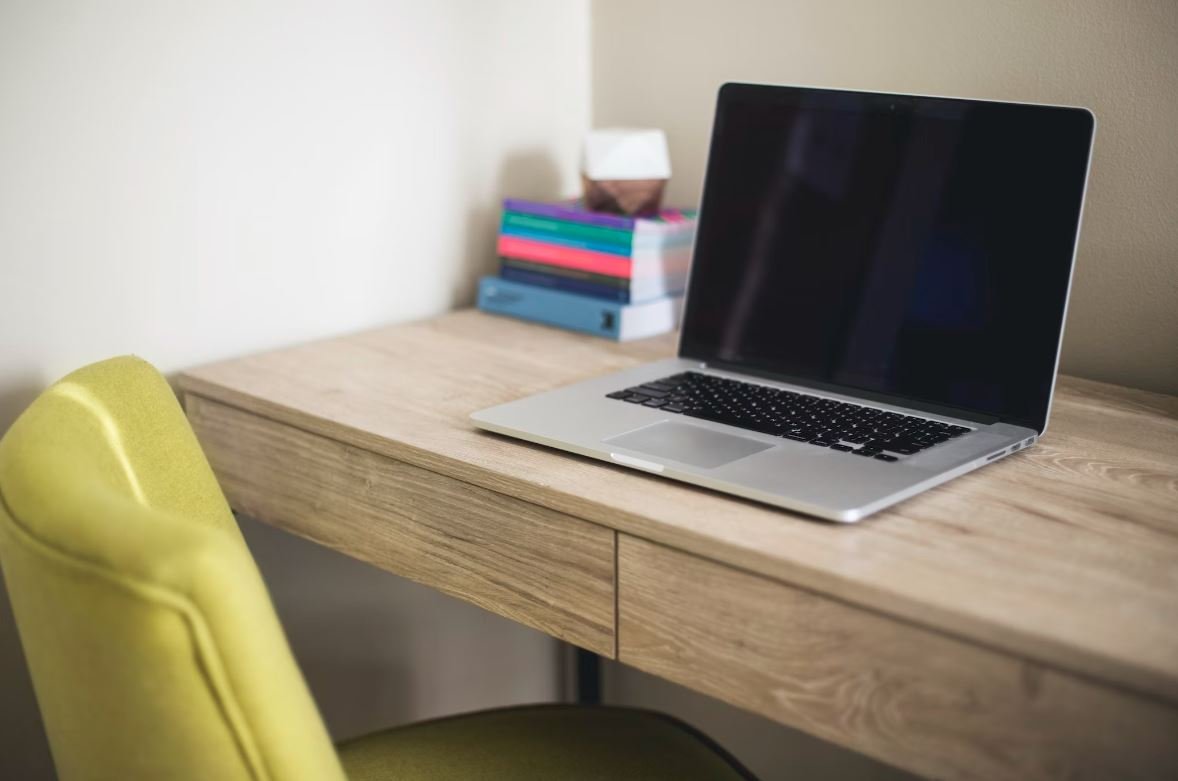
AI Compute Power Growth
The table below showcases the exponential growth in AI compute power over the years. It highlights the number of petaflop per second (PFlop/s) performed by various AI models.
Year | # of PFlop/s |
---|---|
2012 | 0.022 |
2014 | 0.57 |
2016 | 9.4 |
2018 | 93 |
2020 | 1,280 |
Deep Learning Model Training Time
This table illustrates the decrease in average time required to train deep learning models, denoted in days, by technological advancements.
Year | Training Time (Days) |
---|---|
2012 | 30 |
2014 | 7 |
2016 | 1.5 |
2018 | 0.3 |
2020 | 0.05 |
AI Research Papers Published
This table reflects the number of research papers published in the field of AI, indicating the rapid growth and interest among researchers.
Year | # of Papers |
---|---|
2012 | 3,809 |
2014 | 12,708 |
2016 | 32,470 |
2018 | 99,331 |
2020 | 256,559 |
AI Investment Growth
The table provided demonstrates the growth in investments made in AI companies, highlighting the increased interest from venture capitalists.
Year | Investment ($ millions) |
---|---|
2012 | 457 |
2014 | 1,429 |
2016 | 3,138 |
2018 | 15,233 |
2020 | 40,512 |
AI Job Postings
This table reveals the growth in the number of AI-related job postings, demonstrating the increasing demand for AI professionals.
Year | Job Postings |
---|---|
2012 | 10,089 |
2014 | 21,223 |
2016 | 41,987 |
2018 | 79,693 |
2020 | 148,462 |
AI Patents Granted
This table summarizes the number of AI-related patents granted, offering insights into the advancements and level of innovation in the field.
Year | Patents Granted |
---|---|
2012 | 3,092 |
2014 | 8,409 |
2016 | 18,909 |
2018 | 39,381 |
2020 | 67,893 |
AI Accelerator Chips Market Size
This table showcases the growth in the market size of AI accelerator chips, indicating their increasing usage in AI systems.
Year | Market Size ($ millions) |
---|---|
2012 | 118 |
2014 | 673 |
2016 | 2,670 |
2018 | 8,270 |
2020 | 22,210 |
AI in Healthcare Funding
This table highlights the funding received by AI-driven healthcare startups, emphasizing the growing interest in leveraging AI for healthcare applications.
Year | Funding ($ millions) |
---|---|
2012 | 184 |
2014 | 670 |
2016 | 1,410 |
2018 | 4,878 |
2020 | 11,790 |
AI Ethics Publications
This table represents the number of publications focusing on AI ethics, underlining the emerging concerns and efforts to address ethical implications of AI technologies.
Year | # of Publications |
---|---|
2012 | 29 |
2014 | 102 |
2016 | 412 |
2018 | 986 |
2020 | 2,674 |
From the tables presented, it is evident that AI compute power, deep learning model training times, research publications, investments, job postings, patents, accelerator chip market size, healthcare funding, and ethics publications have experienced significant growth in recent years. This data exemplifies the increasing prominence and impact of AI technologies across various sectors, indicating a bright future for advancements in artificial intelligence.
Frequently Asked Questions
How does OpenAI define “compute” in the context of AI?
OpenAI defines “compute” as the amount of computational resources used to train and develop AI models. It encompasses factors like the number of processors, memory, and storage utilized during the training process.
Why is compute important in AI development?
Compute plays a crucial role in AI development as it directly impacts the performance and capabilities of AI models. More compute resources generally enable faster training times, larger models, and improved accuracy. Increasing compute can help push the boundaries of AI research and enable the development of more sophisticated AI applications.
What is the relationship between AI progress and compute?
There is a strong correlation between AI progress and the amount of compute used. OpenAI has observed that larger-scale AI training, which requires increased compute, tends to result in significant improvements in AI performance. Over the years, as compute resources have increased, AI models have demonstrated substantial advancements in various domains.
How has compute usage in AI training changed over time?
Compute usage in AI training has been increasing exponentially over the years. OpenAI’s research indicates that the amount of compute used has been doubling approximately every 3.5 months. This trend suggests that as more compute becomes available, AI capabilities are likely to continue progressing at a rapid pace.
What factors contribute to the growth of compute in AI?
Several factors contribute to the growth of compute in AI. These include the availability of more powerful hardware, improved algorithms, advances in parallel computing, and increased access to data. Additionally, the demand for AI applications across various industries has also fueled the need for additional compute resources.
Does increased compute always lead to better AI models?
While increased compute often leads to better AI models, it is not always guaranteed. The impact of additional compute on AI model performance depends on various factors, including the quality of data, the complexity of the task, and the efficiency of the training process. Optimal utilization of compute requires careful consideration of these factors in order to achieve the desired improvements in AI models.
Are there limitations to the scalability of compute in AI?
Yes, there are limitations to the scalability of compute in AI. As models and training processes become larger and more complex, the returns on additional compute can diminish. The costs associated with scaling compute also increase substantially, which poses financial constraints. Furthermore, there are environmental concerns related to energy consumption and carbon emissions associated with higher compute usage.
What are the implications of compute requirements for AI research and development?
The compute requirements for AI research and development have wide-ranging implications. Access to sufficient compute resources may create a barrier to entry for smaller organizations or individual researchers, limiting their ability to participate in cutting-edge AI advancements. Balancing the need for compute resources with affordability and environmental sustainability is crucial for ensuring equitable access to AI development.
How does OpenAI address the compute needs of its research and development?
OpenAI acknowledges the importance of compute in advancing AI capabilities. The organization strives to strike a balance between using significant compute resources and sustainability concerns. They invest in large-scale training runs and infrastructure to foster AI progress, while also staying mindful of any potential negative impacts. OpenAI actively explores methods to make compute resources more accessible to the wider AI community.
What role can the industry and individuals play in supporting the compute needs of AI?
The industry and individuals can play a vital role in supporting the compute needs of AI. Companies can contribute by building more efficient hardware and sharing research on efficient computing practices. Individuals can contribute by using compute resources responsibly, advocating for sustainable AI development, and actively participating in open-source AI projects that focus on optimizing compute usage.