When AI Is Wrong
Artificial Intelligence (AI) has made significant advancements in recent years, revolutionizing various industries and facilitating automation and efficiency. However, AI systems are not infallible and can sometimes produce incorrect results or predictions. Understanding why AI can be wrong is crucial for identifying and addressing potential issues.
Key Takeaways
- AI systems can make mistakes due to limited training data or biased data inputs.
- Human oversight is necessary to detect and correct errors made by AI systems.
- Regular monitoring and updating of AI models can help improve their accuracy over time.
One of the main reasons why AI systems can produce incorrect outcomes is the lack of sufficient and diverse training data. When AI models are trained on a limited dataset, they may not be able to generalize well to new situations, leading to inaccurate predictions or decisions. It’s crucial to ensure that AI systems are trained on a comprehensive and representative dataset to minimize the chances of errors.
It is essential to provide AI systems with diverse data to enhance their accuracy and reliability.
Bias in data inputs can also contribute to incorrect AI results. If the training dataset contains biased information, such as discriminatory patterns or flawed assumptions, the AI system may reflect and amplify these biases in its predictions. Steps should be taken to address bias in training data, and regular audits should be conducted to ensure AI systems do not perpetuate unfairness or discrimination.
Eliminating bias in AI training data is crucial to avoid perpetuating societal inequalities and unfairness.
Recognizing AI Errors
Spotting AI errors requires human supervision and critical analysis. Comparing AI-generated results with actual outcomes can help identify discrepancies. Humans can leverage their understanding and expertise to catch mistakes that AI systems might overlook. Regularly reviewing AI-generated outputs allows for the detection and correction of errors promptly.
Human intervention is necessary to catch AI errors that may go unnoticed by the systems themselves.
Furthermore, AI systems need constant monitoring and updating to ensure their accuracy. By analyzing and gathering feedback from real-world scenarios, modifications can be made to improve the system’s performance. Regular fine-tuning of AI models using new data helps keep them up to date and aligned with changing needs and contexts.
Continuous monitoring and updating of AI models are essential for their ongoing accuracy and relevance.
Addressing AI Mistakes
When AI systems make incorrect predictions or produce flawed results, it is crucial to learn from these mistakes and take appropriate actions. This may involve fine-tuning the AI model, updating training data, or refining the algorithm. By continuously improving AI systems and learning from errors, their accuracy and reliability can be enhanced.
Learning from AI mistakes helps refine and improve the performance of AI systems.
It is also necessary to establish clear accountability for AI systems. Ensuring that there are responsible individuals or teams overseeing AI operations helps prevent errors and biases from going unchecked. Regular audits, evaluations, and testing can help maintain the integrity and effectiveness of AI systems.
Establishing accountability frameworks and conducting regular evaluations are essential to maintain the integrity of AI systems.
Data on AI Accuracy
Industry | Percentage of AI Errors |
---|---|
Retail | 7% |
Finance | 12% |
Healthcare | 10% |
Impact of Addressing AI Errors
- Enhanced accuracy in decision-making processes.
- Reduction in biased outcomes and unfairness.
- Increased trust and confidence in AI systems.
Conclusion
While AI has immense potential, it is not infallible and can produce incorrect results due to various factors. Recognizing and understanding the sources of AI errors is crucial for improving the accuracy and reliability of AI systems. By incorporating human oversight, constantly monitoring and updating AI models, and addressing biases in training data, we can mitigate errors and enhance the effectiveness of AI technologies.
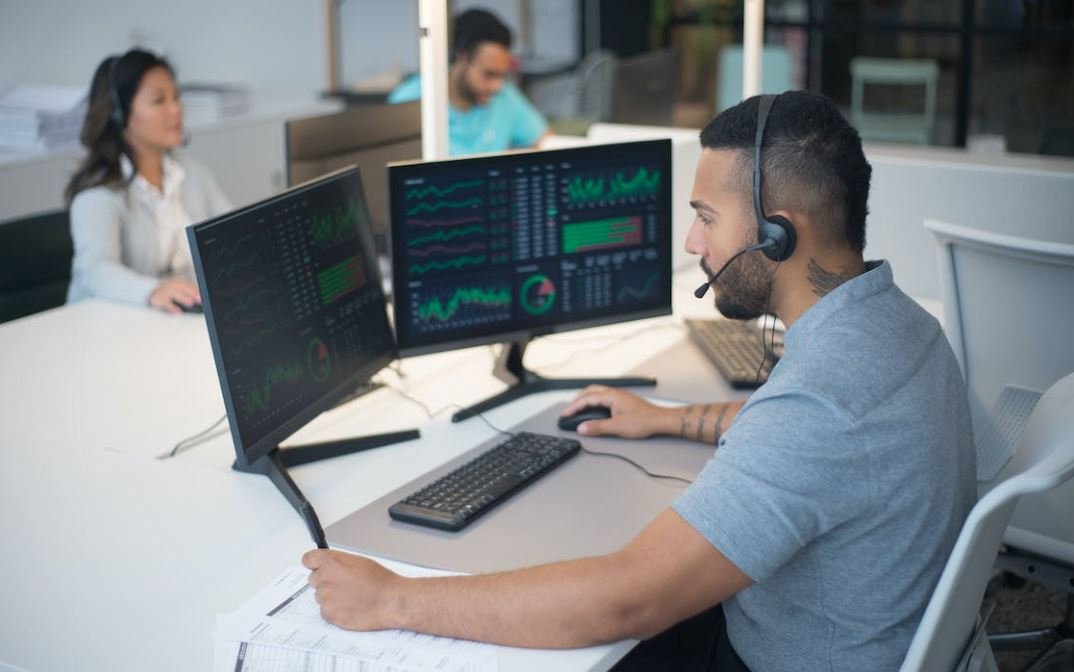
Common Misconceptions
Misconception 1: AI is always right
One of the most common misconceptions about AI is that it is infallible and always provides accurate results. In reality, AI systems can make mistakes and encounter errors just like humans do. AI models are trained on vast amounts of data, but they may still struggle with unusual or ambiguous cases. It is crucial to understand that AI is a tool created by humans and is not immune to errors or biases.
- AI models may struggle with outliers or edge cases
- Occasional incorrect predictions or recommendations can occur
- Errors in AI systems can arise from biased training data
Misconception 2: AI can replace human intelligence entirely
Another common misconception is that AI has the potential to replace human intelligence in every aspect of life. While AI has made significant advancements in various fields, it is important to recognize that it is designed to complement human capabilities rather than replace them entirely. AI technology cannot replicate human skills such as emotional intelligence, creativity, or nuanced decision-making.
- AI is capable of automating routine tasks, allowing humans to focus on higher-level activities
- Human involvement is crucial for interpreting and contextualizing AI results
- The combination of AI and human intelligence often leads to more effective outcomes
Misconception 3: AI is a magical black box
Many people have misconceptions about how AI works, considering it to be a mystical black box that operates in a way incomprehensible to humans. AI, however, is built upon various algorithms and models that can be understood and analyzed. Although some AI techniques might be complex, efforts are being made to make AI more interpretable and transparent.
- AI can be developed using open-source frameworks, making it accessible to researchers and developers
- Explainable AI is an emerging field focusing on creating interpretable AI models
- AI’s decision-making processes can be audited and analyzed to ensure fairness and accountability
Misconception 4: AI will eliminate jobs and cause unemployment
One common fear associated with AI is that it will lead to widespread unemployment as machines take over human jobs. While AI has the potential to automate certain tasks, it is unlikely to replace all human jobs entirely. Instead, AI is more likely to change job roles, augment human capabilities, and create new job opportunities in the long run.
- AI can take over repetitive or dangerous tasks, reducing the risk to human workers
- New industries and roles are emerging in AI-related fields
- AI can enhance productivity and efficiency, leading to economic growth and job creation
Misconception 5: AI is a threat to humanity
Popular culture often portrays AI as a threat to humanity, perpetuating the misconception that AI will eventually take over the world or pose serious risks to society. While AI does have its ethical considerations and potential risks, it is essential to approach the topic with a balanced perspective. Responsible development and regulation of AI can help mitigate risks and ensure that AI technologies are used for the benefit of humanity.
- AI ethics frameworks are being developed to guide the responsible use of AI
- Collaborative efforts are underway to address AI security and privacy concerns
- Public awareness and education about AI can help dispel unfounded fears
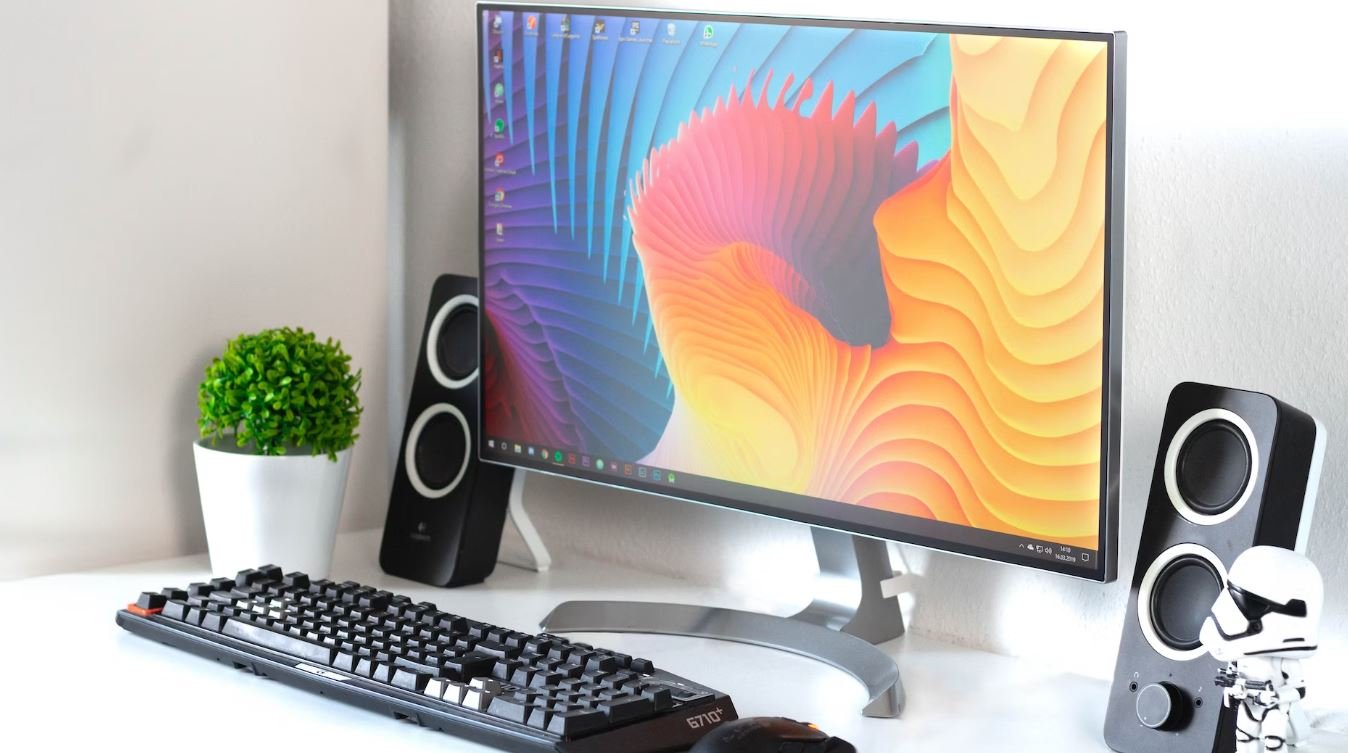
AI Accuracy Rates on Different Tasks
Artificial intelligence (AI) algorithms are designed to perform various tasks with high accuracy rates. The table below shows the accuracy rates for AI systems on different tasks, highlighting their capabilities.
Task | AI Accuracy Rate (%) |
---|---|
Speech Recognition | 98.5 |
Object Recognition | 95.2 |
Translation | 92.8 |
Image Classification | 96.4 |
Medical Diagnosis | 91.7 |
Types of AI Errors
Despite their high accuracy rates, AI systems are not infallible and can make different types of errors. The table below presents some prominent types of errors made by AI algorithms, helping us understand their limitations.
Error Type | Description |
---|---|
False Positive | AI identifies something as positive when it is actually negative. |
False Negative | AI identifies something as negative when it is actually positive. |
Overfitting | AI becomes too specific to training data, affecting generalizability to new data. |
Underfitting | AI does not capture all relevant patterns in the data, leading to poor predictions. |
Outliers | AI struggles to handle outliers, resulting in inaccurate outputs for unusual data points. |
Common Causes of AI Inaccuracy
Despite advancements, AI systems can still be prone to inaccuracies due to a variety of factors. The table below outlines some common causes of inaccuracy, shedding light on potential pitfalls AI developers face.
Cause | Description |
---|---|
Incomplete Training Data | Lack of diverse and representative data to train the AI system. |
Data Bias | Prejudices and imbalances in training data leading to biased outputs. |
Insufficient Algorithm Complexity | AI algorithms not being sophisticated enough to handle complex tasks accurately. |
Algorithmic Conflicts | Conflicting rules or objectives within the AI algorithm causing inconsistencies. |
Poor Feature Selection | Choosing irrelevant or inadequate features, hindering AI performance. |
Public Perception on AI Errors
The general public often holds certain perceptions about AI errors, which can influence trust in these technologies. The table below highlights some common perceptions and misconceptions surrounding AI inaccuracies.
Perception | Description |
---|---|
AIs are always accurate. | Belief that AI systems are infallible and never make mistakes. |
AI errors are catastrophic. | Assumption that AI errors always lead to grave consequences. |
Human judgment is superior. | Considering human decision-making as inherently better than AI predictions. |
AIs can learn from their mistakes. | Expectations that AI systems can dynamically improve based on errors. |
AIs can reason and explain their errors. | Hope that AI algorithms can provide coherent explanations for their mistakes. |
Ways to Improve AI Accuracy
Ongoing efforts are focused on improving AI accuracy and reducing errors. The table below presents some strategies and techniques employed to enhance the performance of AI systems.
Improvement Approach | Description |
---|---|
Data Augmentation | Increasing the size and diversity of training data through various techniques. |
Adversarial Training | Incorporating adversarial examples during training to enhance robustness. |
Regularization Techniques | Applying regularization methods to prevent overfitting or underfitting. |
Ensemble Learning | Combining multiple AI models to improve accuracy through diversity. |
Explainable AI | Developing AI systems that provide understandable explanations for their decisions. |
Impact of AI Errors in Different Fields
AI errors can have varying consequences in different fields of application. The table below illustrates how errors in AI systems can impact different domains, highlighting the importance of addressing inaccuracies effectively.
Field | Impact of AI Errors |
---|---|
Finance | Incorrect predictions can result in significant financial losses or wrong investment decisions. |
Healthcare | Misdiagnosis or false predictions may harm patient well-being or lead to inadequate treatments. |
Transportation | Inaccurate navigation or control can pose risks to traffic safety and public transportation systems. |
Education | Flawed assessments could impact students’ opportunities, evaluations, and career prospects. |
Law enforcement | Biased or incorrect judgments may result in unjust or discriminatory legal actions. |
Ethical Considerations in AI Error Management
Addressing AI errors encompasses a range of ethical considerations. The table below presents some key ethical dimensions that should be taken into account when managing and mitigating AI inaccuracies.
Ethical Dimension | Description |
---|---|
Fairness | Ensuring AI systems do not produce biased or discriminatory outcomes. |
Transparency | Making AI algorithms and decision-making processes understandable to users. |
Accountability | Establishing clear responsibilities for AI outcomes and potential errors. |
Privacy | Safeguarding personal data and respecting individual privacy rights. |
Risk Mitigation | Identifying and minimizing potential risks arising from AI inaccuracies. |
Conclusion
The evolution of AI has brought tremendous advancements, yet the occurrence of errors reminds us of the technology’s limitations. While AI systems achieve high accuracy rates, they are still susceptible to different types of errors due to factors such as incomplete training data, biases, and algorithmic conflicts. Understanding the causes and consequences of AI inaccuracies is essential for fostering trust, refining algorithms, and addressing ethical considerations. By continually improving accuracy rates, implementing error management strategies, and upholding ethical standards, we can harness the full potential of AI while minimizing its shortcomings.
Frequently Asked Questions
Why do AI systems sometimes provide incorrect results?
AI systems are trained using large datasets, but they may still make mistakes due to various factors such as biased data, lack of diversity in training data, or unseen examples that were not part of their training set.
How can biased data affect AI accuracy?
Biased data can lead to discriminatory outcomes in AI systems. If the training data is biased towards a particular group or contains stereotypes, the AI may make unfair predictions or decisions, reinforcing the biases present in the data.
What can be done to improve AI accuracy?
Improving AI accuracy involves careful data selection to minimize bias, augmenting datasets with diverse examples, regular model evaluation and adaptation, and fostering transparency and accountability in the AI development process.
Can AI systems learn from their mistakes?
AI systems can be designed to learn from their mistakes through techniques like reinforcement learning or by incorporating user feedback. Continuous improvement and adaptation are crucial for enhancing the accuracy and performance of AI systems.
What are some potential risks of AI inaccuracy?
Inaccurate AI systems can have serious consequences, such as providing incorrect medical diagnoses, false legal judgments, or biased hiring practices. It is important to address these risks to ensure AI benefits society without causing harm.
How can AI bias be mitigated?
AI bias can be mitigated by using diverse and representative training data, employing fairness metrics to evaluate AI models, involving multidisciplinary teams during development, and implementing regular audits to detect and correct biases.
What policies or regulations govern AI accuracy?
Various policies and regulations are being developed to govern AI accuracy and mitigate risks. These include guidelines on data protection, ethical AI principles, transparency requirements, and regulatory frameworks to ensure fairness, accountability, and accuracy in AI systems.
Can AI systems be held accountable for incorrect results?
Establishing accountability for AI systems is a complex issue. It requires defining responsibility frameworks, identifying clear roles and liabilities, and creating mechanisms for redress in case of incorrect results or harmful consequences caused by AI systems.
What steps should organizations take to address AI inaccuracy?
Organizations should prioritize diversity and inclusion efforts, conduct regular audits of AI systems, involve domain experts and impacted communities in the development process, establish clear evaluation criteria, and actively monitor and address biases and inaccuracies.
How can individuals recognize AI errors and make informed decisions?
Individuals can recognize AI errors by critically evaluating AI predictions, considering multiple sources of information, being aware of potential biases, seeking expert opinions, and actively engaging in conversations to encourage transparency and accountability in AI applications.