Journal AI in Medicine
Artificial Intelligence (AI) has become a game-changer in the field of medicine, revolutionizing the way doctors diagnose and treat diseases. The integration of AI technology into medical research and practice has led to advancements in precision medicine, clinical decision support, and patient care. In this article, we will explore how AI is transforming the field of medicine and the implications it holds for the future of healthcare.
Key Takeaways:
- Artificial Intelligence (AI) is revolutionizing medicine by improving the accuracy and efficiency of diagnosis and treatment.
- AI-powered systems can analyze complex data sets and identify patterns to provide personalized treatment plans.
- Advancements in AI-based medical imaging are enabling earlier detection of diseases and enhancing patient outcomes.
- AI is helping to bridge the gap between research and clinical practice, accelerating the development of new treatments and therapies.
- While AI holds immense potential, ethical considerations and data privacy concerns must be addressed to ensure responsible and equitable use of the technology.
AI has been particularly successful in medical imaging, where algorithms can analyze large quantities of medical images to detect patterns and abnormalities. These algorithms can evaluate **MRI** scans, **CT** scans, and **X-rays**, aiding radiologists in interpreting complex images. By automating this process, AI can improve accuracy and speed, leading to more timely diagnoses and treatment plans.
Another exciting application of AI in medicine is the development of predictive models that can analyze patient data to forecast disease progression and recommend personalized treatment options. Machine learning algorithms can analyze vast amounts of patient data, including genetic information, medical history, and lab results, to identify risk factors and make **prognostic predictions**. This enables doctors to provide targeted interventions and improve patient outcomes.
Advancements in AI-based Medical Imaging
In recent years, AI has demonstrated remarkable progress in medical imaging, revolutionizing the way diseases are detected and diagnosed. AI-powered algorithms can analyze medical images with exceptional precision and accuracy. For example, studies have shown that AI algorithms can detect **cancerous lesions** from mammography images with comparable accuracy to expert radiologists. This technology holds great promise for improving early detection rates and reducing false-positive results.
The Impact of AI on Research and Clinical Practice
AI is bridging the gap between medical research and clinical practice. By analyzing vast amounts of scientific literature, AI-powered systems can identify meaningful correlations and patterns that could lead to new treatments and therapies. This technology assists researchers in sifting through large volumes of data and identifying potential drug candidates. Additionally, AI can aid in clinical trials by optimizing patient selection criteria and monitoring treatment response.
*AI is ushering in a new era of collaboration between humans and machines in the field of medicine, accelerating the discovery and delivery of innovative healthcare solutions.*
Table 1: Comparison of AI Methods for Disease Diagnosis
AI Method | Advantages | Limitations |
---|---|---|
Machine Learning |
|
|
Deep Learning |
|
|
Despite the immense potential of AI in medicine, several ethical considerations and data privacy concerns need to be addressed. As AI systems rely on vast amounts of patient data, ensuring the privacy and security of patient information is crucial. Additionally, there is a need for transparency and interpretability in AI algorithms, as the decision-making process should be understandable and accountable.
Table 2: AI Applications in Medicine
Application | Description |
---|---|
Virtual Assistants | AI-powered virtual assistants can provide personalized medical advice to patients and assist in managing chronic conditions. |
Robot-Assisted Surgery | AI-guided robotic systems enhance surgical precision, improve outcomes, and reduce surgical errors. |
Drug Discovery | AI can analyze vast amounts of chemical compounds and predict their efficacy in drug development. |
As AI continues to advance in medicine, it is important to prioritize transparency, accountability, and patient safety to ensure the responsible adoption of this technology. Collaborative efforts between healthcare professionals, researchers, and policymakers are essential to shape policies and regulations that promote the ethical use of AI and ensure equitable access to innovative healthcare solutions.
Table 3: Benefits and Challenges of AI in Medicine
Benefits | Challenges |
---|---|
|
|
AI’s integration into medicine is transforming the healthcare landscape, empowering doctors with advanced tools and knowledge. As AI continues to evolve, its potential to improve patient outcomes and revolutionize healthcare delivery is becoming increasingly apparent. With responsible and ethical implementation, AI holds the promise of a brighter and healthier future for all.

Common Misconceptions
Paragraph 1
One common misconception about AI in medicine is that it will replace healthcare professionals.
- AI complements healthcare professionals, enhancing their capabilities.
- AI cannot provide the same level of empathy and human touch as healthcare professionals.
- AI relies on healthcare professionals to interpret and validate its findings.
Paragraph 2
Another misconception is that AI in medicine is only beneficial for diagnosing diseases.
- AI also plays a vital role in treatment planning and personalized medicine.
- AI can help with medication management and predicting adverse reactions.
- AI enables continuous monitoring of patients, improving care quality and patient outcomes.
Paragraph 3
Some people mistakenly believe that AI in medicine is error-free and infallible.
- AI can still make mistakes and is not immune to issues like bias and data errors.
- AI requires rigorous training and testing to minimize errors and improve accuracy.
- The responsibility for final decision-making still lies with healthcare professionals.
Paragraph 4
There is a misconception that AI in medicine is a black box and cannot be explained.
- AI algorithms can be designed to provide explanations for their decisions.
- Explainable AI (XAI) is an active research area to make AI more transparent and accountable.
- Patients and healthcare professionals can demand transparency in AI systems used in medicine.
Paragraph 5
Lastly, some individuals believe that AI in medicine will lead to job losses.
- AI creates new job opportunities in healthcare, such as AI specialists and data scientists.
- Healthcare professionals can focus more on complex cases while AI handles routine tasks.
- AI can augment the workforce, improving efficiency and patient care outcomes.
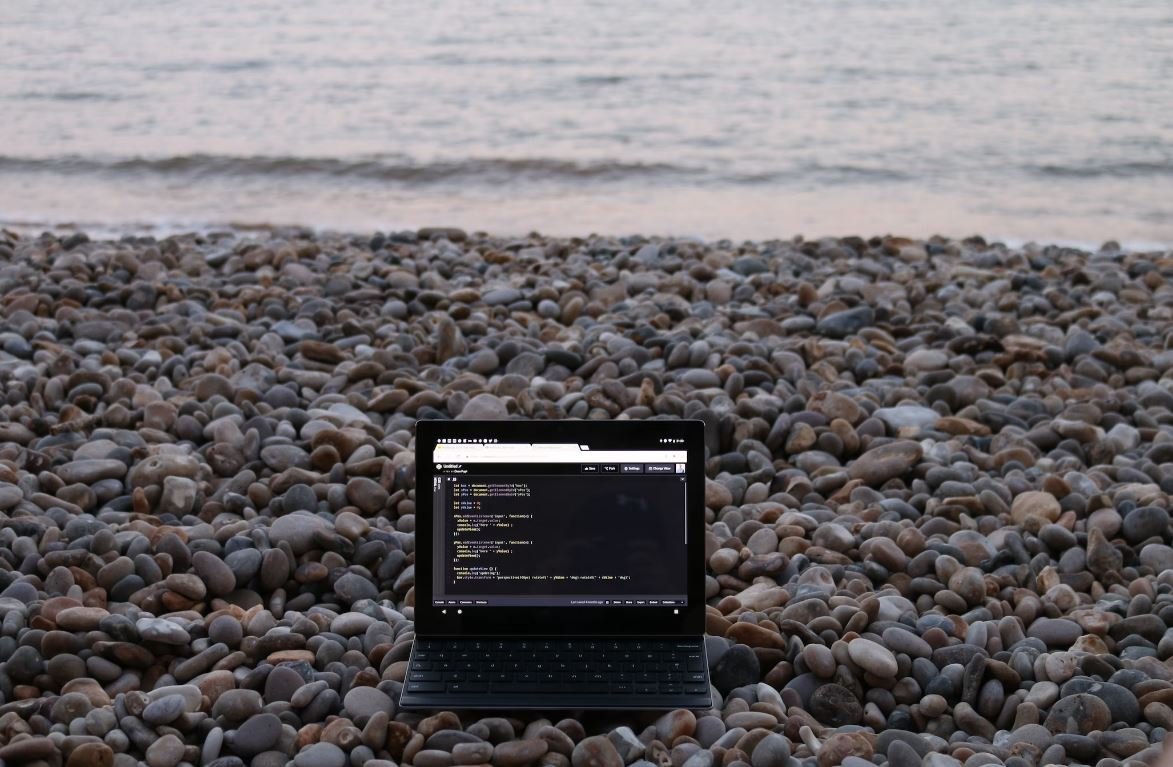
Artificial Intelligence in Radiology
Radiology is a medical specialty that uses different imaging techniques to diagnose and treat diseases. The rapid advancement of AI technology has had a significant impact on radiology, revolutionizing the way doctors analyze and interpret medical images. Below are ten tables highlighting the advancements and applications of AI in radiology.
AI Applications in Radiology
In recent years, AI algorithms have been deployed in various radiology applications to improve diagnostic accuracy, reduce workload, and enhance patient outcomes. The table below showcases the different applications of AI in radiology.
Application | Description |
---|---|
Tumor Detection | AI algorithms can assist radiologists in identifying and locating tumors in medical images. |
Anomaly Detection | AI algorithms can detect abnormalities or irregularities in medical images, helping radiologists to make accurate diagnoses. |
Image Segmentation | AI algorithms can segment and classify different structures within medical images, aiding in treatment planning. |
Disease Classification | AI algorithms can accurately classify various diseases based on imaging characteristics, providing faster and more reliable diagnoses. |
Quantitative Analysis | AI algorithms can perform automated measurements and calculations on medical images to assist in diagnosis and treatment evaluation. |
Advantages and Challenges of AI in Radiology
While AI has brought numerous benefits to radiology, its implementation also comes with certain challenges. The table below presents some advantages and challenges associated with the integration of AI in radiology practices.
Advantages | Challenges |
---|---|
Improved Diagnostic Accuracy | Legal and Ethical Concerns |
Reduced Interpretation Time | Integration into Clinical Workflow |
Enhanced Radiologist Productivity | Inadequate Training Data |
Early Detection of Abnormalities | Algorithm Bias |
Potential Cost Savings | Regulatory Approval |
AI-Enabled Imaging Modalities
AI algorithms are being incorporated into various imaging modalities to improve diagnostic accuracy, facilitate interpretation, and provide quantitative analysis. The table below demonstrates the integration of AI algorithms with different imaging modalities.
Imaging Modality | AI Integration |
---|---|
Magnetic Resonance Imaging (MRI) | Aided interpretation and automated tumor detection. |
Computed Tomography (CT) | Improved detection of lung nodules and other abnormalities. |
Mammography | AI systems assisting in breast cancer detection and classification. |
Ultrasound | AI-based automated measurements and real-time analysis. |
Positron Emission Tomography (PET) | Quantitative analysis and improved tumor characterization. |
Effects of AI on Radiologist Workflow
The integration of AI into radiology practices influences radiologists’ workflow, enabling them to work more efficiently and effectively. The table below illustrates the impact of AI on radiologist workflow.
Effect | Description |
---|---|
Speeding Up Diagnosis | AI algorithms aid radiologists in making faster and more accurate diagnoses, reducing interpretation time. |
Improved Communication | AI supports seamless collaboration and communication between radiologists and other healthcare professionals. |
Reduced Repetitive Tasks | AI automates repetitive tasks, allowing radiologists to focus more on complex cases and patient care. |
Enhanced Quality Control | AI algorithms can identify potential errors or inconsistencies in medical images, ensuring higher quality interpretations. |
Standardization of Reports | AI systems can generate structured and standardized radiology reports, improving consistency and information exchange. |
Regulation and Ethics in AI Radiology
The implementation of AI in radiology presents several regulatory and ethical considerations that need to be addressed. The table below highlights the key aspects encompassing regulation and ethics in AI radiology.
Regulation | Ethics |
---|---|
Approval and Certification | Transparency and Explainability |
Data Privacy and Security | Avoiding Bias and Discrimination |
Interoperability and Integration | Fair Allocation of Resources |
Monitoring and Accountability | Human Oversight and Collaboration |
Validation and Evaluation | Consent and Patient Autonomy |
Future Perspectives and Challenges
The integration of AI into radiology has a promising future, but it also presents several challenges that need to be overcome. The table below highlights some future perspectives and challenges associated with AI in radiology.
Future Perspectives | Challenges |
---|---|
Improved Diagnostic Accuracy | Algorithm Bias and Inadequate Training Data |
Advanced Automation | Ethical and Legal Concerns |
Personalized Medicine | Data Privacy and Security |
Outcome Prediction | Integration with Clinical Workflow |
Continual Education and Training | Regulatory Approval and Adoption |
Conclusion
AI in radiology has revolutionized the field by improving diagnostic accuracy, reducing interpretation time, and enhancing radiologist productivity. Through the integration of AI into various imaging modalities, the application of AI algorithms has been extended to tumor detection, anomaly detection, image segmentation, disease classification, and quantitative analysis. While AI offers numerous advantages, challenges such as legal and ethical concerns, algorithm bias, and integration into clinical workflow need to be addressed. The future of AI in radiology holds great promise for improved personalized medicine, advanced automation, and outcome prediction; however, ensuring data privacy, addressing regulatory issues, and continual education remain important considerations. With continued research and development, AI will continue to shape the future of radiology, benefiting both healthcare professionals and patients.
Journal AI in Medicine – Frequently Asked Questions
What is AI in medicine?
AI in medicine refers to the use of artificial intelligence techniques, such as machine learning and deep learning, in medical research, diagnosis, treatment, and healthcare management.
How is AI used in medical research?
AI is used in medical research to analyze large datasets, identify patterns and correlations, and make predictions. It can help researchers in areas such as drug discovery, genomics, and clinical trials.
Can AI assist in diagnosing diseases?
Yes, AI can assist in diagnosing diseases. It can analyze medical images, such as X-rays and MRIs, and help radiologists in detecting abnormalities or identifying specific conditions.
Is AI capable of predicting patient outcomes?
AI techniques can be used to analyze patient data and predict outcomes, such as the likelihood of readmission, response to treatment, or disease progression. This can aid in personalized patient care and treatment planning.
How are AI algorithms trained in the medical field?
AI algorithms in the medical field are typically trained using large datasets that contain labeled examples. These examples can be medical images, patient records, or genomic data. The algorithms learn from these examples to make accurate predictions or classifications.
What are the challenges of implementing AI in medicine?
Some challenges of implementing AI in medicine include the need for high-quality and standardized data, ensuring patient privacy and data security, regulatory and ethical considerations, and the need for collaboration between healthcare professionals and AI experts.
Are there any risks associated with AI in medicine?
While AI in medicine has the potential to improve healthcare outcomes, there are risks associated with its use. These may include biases in algorithms, lack of interpretability in AI decision-making, and potential overreliance on AI systems without human oversight.
How can AI benefit healthcare management?
AI can benefit healthcare management by automating administrative tasks, optimizing scheduling and resource allocation, predicting patient demand, and improving operational efficiency. It can also assist in fraud detection and quality assurance.
What are some successful applications of AI in medicine?
Some successful applications of AI in medicine include automated diagnosis of retinal diseases, predicting patient length of stay in hospitals, identifying potential drug targets, and analyzing genomic data to personalize cancer treatment.
How can healthcare professionals leverage AI?
Healthcare professionals can leverage AI by staying updated with AI advancements, collaborating with AI experts for research and implementation, integrating AI tools into clinical workflows, and being involved in the development and evaluation of AI algorithms.