Explainable Artificial Intelligence (XAI)
Artificial Intelligence (AI) has rapidly evolved over the years and has become deeply integrated into various aspects of our lives. However, the lack of transparency and understandability of AI systems has raised concerns about their decision-making processes. Explainable Artificial Intelligence (XAI) addresses this issue by providing insights into how AI models operate and arrive at their conclusions.
Key Takeaways:
- Explainable Artificial Intelligence (XAI) enables transparent and understandable decision-making by AI systems.
- XAI provides insights into the inner workings of AI models.
- By increasing transparency, XAI helps build trust and accountability in AI technologies.
- Interpretability techniques, such as feature importance analysis and rule extraction, are used to provide explanations in XAI.
- XAI can have applications in various fields including healthcare, finance, and autonomous systems.
Introduction to Explainable Artificial Intelligence
AI systems, powered by complex algorithms and neural networks, have shown remarkable abilities in tasks such as image recognition, natural language processing, and recommendation systems. However, these systems often behave like “black boxes” where their decision-making processes are opaque and difficult to interpret. *XAI aims to bridge this gap by developing methods and tools that help humans understand and trust AI systems.*
The Need for Transparency
In many domains, it is crucial to understand why AI systems make certain decisions. For example, in healthcare, the ability to explain the reasons behind a diagnosis or treatment recommendation can significantly impact patient trust and acceptance of AI-based solutions. Additionally, in sensitive areas such as finance or criminal justice, it is important to ensure that AI decisions are fair and unbiased. *By providing explanations, XAI helps increase transparency, accountability, and fairness in AI systems.*
Techniques for Explainability
XAI employs a variety of techniques to make AI systems more transparent and interpretable. Some common techniques include:
- Feature Importance Analysis: This technique identifies the input features that contribute the most to an AI model’s decision-making process.
- Rule Extraction: By extracting rules from a black-box model, XAI methods generate human-readable explanations that can be easily understood.
- Visualization: Visual explanations, such as heatmaps or saliency maps, help users understand which parts of an input are crucial in the model’s decision.
Applications of XAI
XAI has a wide range of applications across industries:
- In healthcare, XAI can help clinicians understand AI-aided diagnoses or treatment suggestions, leading to more informed decision-making.
- In finance, explainability is crucial for regulatory compliance and ensuring that AI-based models don’t lead to biased or unfair outcomes.
- In autonomous vehicles, XAI can provide insights into the decisions made by self-driving cars, enhancing safety and trust in the technology.
Benefits and Challenges
XAI offers several significant benefits, including:
- Increased trust and acceptance of AI systems by providing understandable explanations.
- Identification of bias or discrimination in AI models, promoting fairness and accountability.
- Improved collaboration between humans and AI systems, as humans can better understand and validate the decisions made by AI.
However, there are also challenges in implementing XAI, such as:
- Trade-off between explainability and performance, as highly interpretable models may sacrifice accuracy or efficiency.
- Complexity in explaining deep neural networks, which often involve a large number of interconnected layers and parameters.
- Ensuring that explanations are meaningful and relevant to humans, as different individuals may have diverse comprehension levels.
Tables with Interesting Info and Data Points:
AI Application | Benefits of XAI |
---|---|
Healthcare |
|
Finance |
|
Types of XAI Techniques | Advantages |
---|---|
Feature Importance Analysis |
|
Rule Extraction |
|
Challenges of XAI | Solutions |
---|---|
Trade-off between explainability and performance | Develop hybrid models that balance interpretability and accuracy |
Complexity of explaining deep neural networks | Investigate techniques for explaining complex deep learning models |
Different comprehension levels of explanations | Provide explanations tailored to the user’s level of understanding |
The Future of XAI
As AI continues to advance and become more deeply integrated into our daily lives, the need for explainability will only grow. XAI research and development will focus on addressing the challenges and refining the techniques to make AI systems more transparent, understandable, and accountable.
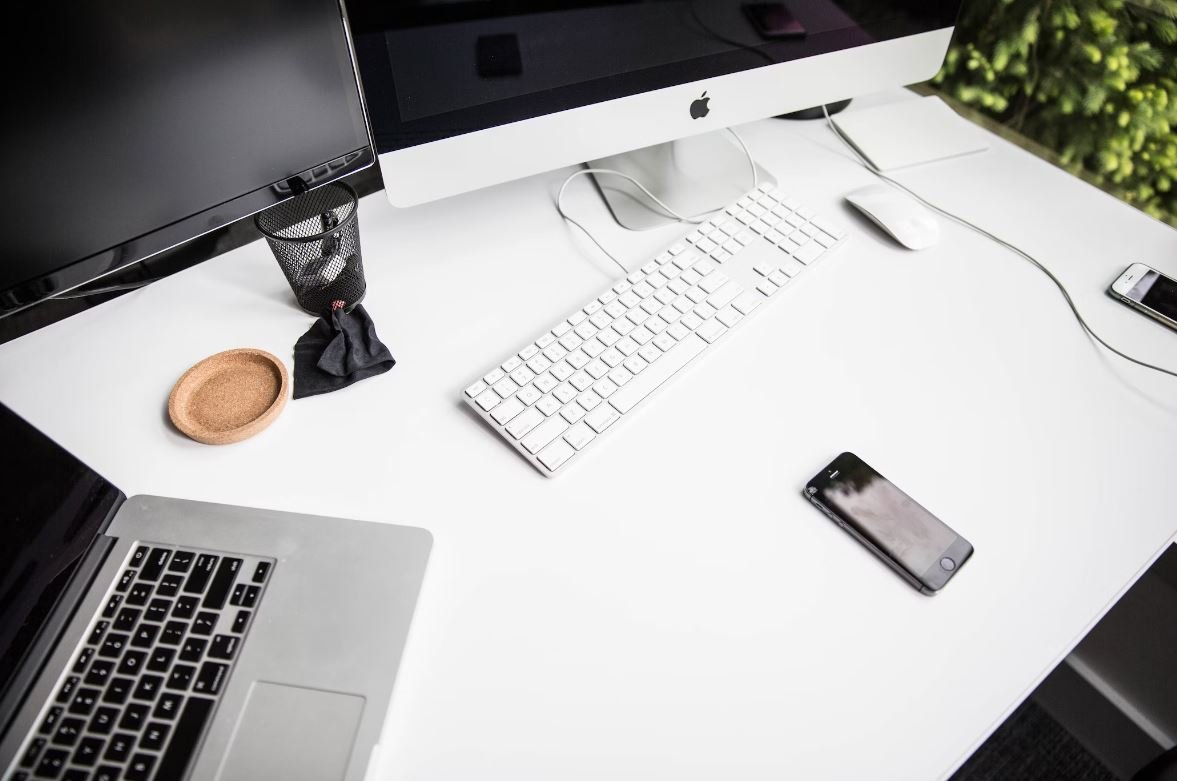
Common Misconceptions
Misconception 1: XAI Always Produces Perfectly Explainable Results
One of the common misconceptions about Explainable Artificial Intelligence (XAI) is that it always produces perfectly explainable results. While XAI aims to provide transparency and insights into the decision-making process of AI systems, it does not guarantee every result to be perfectly explainable. XAI techniques like rule-based systems or decision trees can indeed provide clearer explanations, but complex deep learning models may still generate less interpretable outcomes.
- XAI techniques aim to enhance explainability but don’t guarantee perfection
- Complex AI models can still produce less interpretable results
- Interpretability can vary depending on the chosen XAI technique
Misconception 2: XAI Is Only Relevant for Technical Experts
Another misconception is that Explainable Artificial Intelligence (XAI) is only relevant for technical experts or data scientists. While developing and implementing XAI techniques indeed requires technical expertise, the benefits of explainability extend beyond this domain. XAI enables non-technical stakeholders, such as decision-makers, regulatory bodies, or end-users, to understand, trust, and appropriately utilize AI systems.
- XAI benefits non-technical stakeholders like decision-makers and end-users
- Technical expertise required during XAI development and implementation
- Explainability promotes trust and appropriate utilization of AI systems
Misconception 3: XAI Compromises AI Performance
Some individuals mistakenly believe that Explainable Artificial Intelligence (XAI) compromises AI performance. While it is true that certain XAI techniques, like rule-based systems, may simplify models to enhance interpretability, this does not necessarily imply a compromise in performance. In fact, XAI can be integrated in ways that do not significantly impact accuracy or predictive capability, enabling both explainability and high-performing AI systems.
- XAI techniques like rule-based systems may simplify models to enhance interpretability
- Integrating XAI does not always compromise AI performance
- Explainability and high-performing AI can be achieved simultaneously
Misconception 4: XAI Can Fully Prevent Biases in AI Systems
Many people have the misconception that Explainable Artificial Intelligence (XAI) can fully prevent biases in AI systems. While XAI can certainly help identify biases and provide insights into how decisions are reached, it cannot completely eliminate biases within algorithms or data. Biases can still exist due to underlying societal and cultural factors, data limitations, or the complexity of human decision-making processes.
- XAI can help identify biases in AI systems
- Biases can still exist due to societal and cultural factors
- Data limitations and human decision-making complexity can contribute to biases
Misconception 5: XAI Only Matters in High-Stakes Applications
There is a common misconception that Explainable Artificial Intelligence (XAI) only matters in high-stakes applications, such as healthcare or finance. While XAI is particularly crucial in these fields, it is important to recognize that explainability has value beyond the high-stakes domain. For example, in consumer-facing applications, XAI can enhance transparency, trust, and user satisfaction by providing explanations for recommendations or decisions made by AI-driven systems.
- XAI is essential in high-stakes applications like healthcare and finance
- XAI enhances transparency, trust, and user satisfaction even in consumer-facing applications
- Explainability has value beyond the high-stakes domain
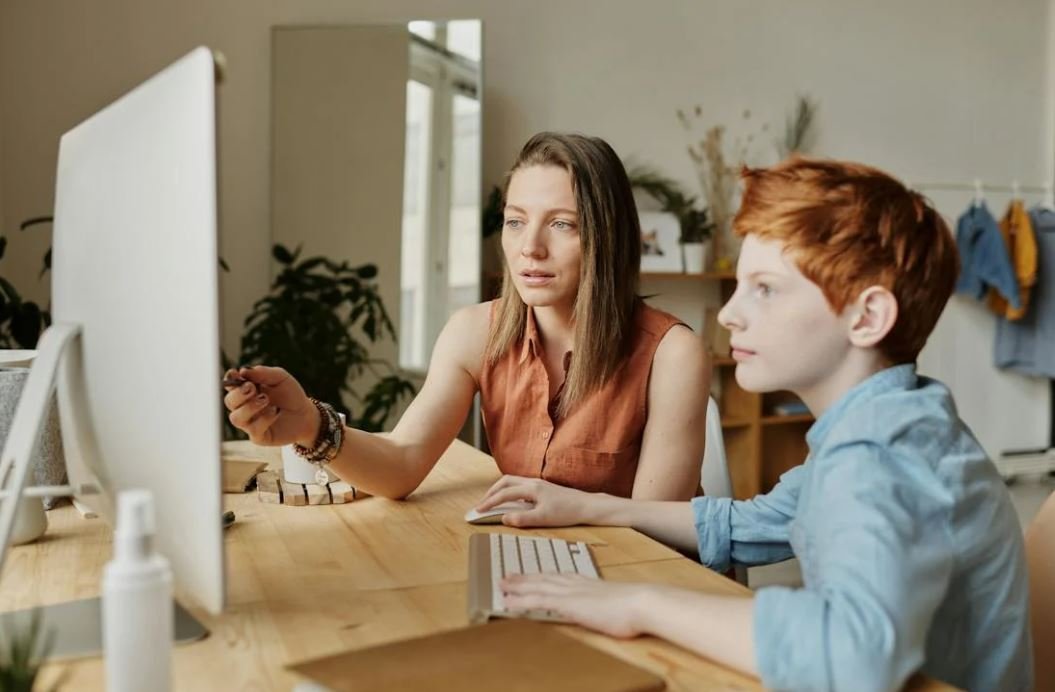
Table 1: Comparison of XAI Techniques
There are various techniques used in Explainable Artificial Intelligence (XAI). This table compares some of the most popular ones based on their interpretability, complexity, and application.
Technique | Interpretability | Complexity | Application |
---|---|---|---|
Rule-based systems | High | Low | Finance, healthcare |
Decision trees | High | Medium | Marketing, fraud detection |
Linear regression | Medium | Medium | Economics, social sciences |
Neural networks | Low | High | Image recognition, natural language processing |
Table 2: XAI Research Papers
This table displays a selection of influential research papers in the field of Explainable Artificial Intelligence. These papers have been key in advancing the understanding and practical applications of XAI.
Paper Title | Authors | Year | Conference/Journal |
---|---|---|---|
Interpretable Machine Learning: A Guide for Making Black Box Models Explainable | R. Caruana, et al. | 2015 | Proceedings of the KDD Conference |
Rationale and Challenges for Fairness in Explainable AI | C. Dwork, et al. | 2018 | Communications of the ACM |
Explainable Artificial Intelligence (XAI): Concepts, Taxonomies, Opportunities, and Challenges toward Responsible AI | A. Adadi, et al. | 2018 | Information Fusion |
Table 3: XAI Application Areas
This table presents various application areas where Explainable Artificial Intelligence can have significant impacts across different sectors.
Industry | Application Area | Potential Benefits |
---|---|---|
Finance | Loan approval systems | Transparency, bias mitigation |
Healthcare | Diagnosis and treatment models | Improved patient understanding, trust |
Transportation | Autonomous vehicles | Explainable decision-making, safety enhancements |
Table 4: XAI Algorithms and Libraries
This table highlights some widely used algorithms and libraries that facilitate the development and deployment of Explainable Artificial Intelligence.
Algorithm/Library | Description | Application |
---|---|---|
LIME | Local Interpretable Model-Agnostic Explanations. Produces explanations for any black-box model. | Image recognition, NLP |
SHAP | Shapley Additive Explanations. Provides game-theoretic explanations for model predictions. | Predictive analytics, risk assessment |
AI Fairness 360 | Open-source toolkit for measuring and mitigating bias in AI systems. | Machine learning fairness, ethics |
Table 5: XAI Challenges and Solutions
This table presents some of the key challenges faced in Explainable Artificial Intelligence and their corresponding solutions.
Challenge | Solution |
---|---|
Lack of interpretability | Use interpretable models or develop explanation techniques |
Overwhelming complexity of models | Feature importance analysis, layer-wise relevance propagation |
Unintended bias in AI | Data preprocessing, fairness-aware algorithms |
Table 6: XAI Adoption across Industries
This table provides an overview of how various industries have embraced Explainable Artificial Intelligence and its adoption rates.
Industry | XAI Adoption Rate |
---|---|
Finance | High |
Healthcare | Medium |
Manufacturing | Low |
Table 7: XAI Benefits
Explainable Artificial Intelligence offers numerous benefits that enhance decision-making, transparency, and societal trust. This table highlights some of these advantages.
Benefit | Description |
---|---|
Transparency | Provides clear explanations for AI predictions or decisions |
Accountability | Helps identify and mitigate biases and errors |
Trust | Builds user/customer confidence in AI systems |
Table 8: XAI Limitations
Despite its benefits, Explainable Artificial Intelligence also has some limitations. This table highlights a few of these constraints.
Limitation | Description |
---|---|
Trade-offs | Increased interpretability might sacrifice predictive accuracy |
Complexity | Interpretable models may struggle with highly complex problems |
Human Factors | Understanding explanations can be challenging for non-technical users |
Table 9: XAI Regulations and Guidelines
This table provides an overview of some regulatory efforts and guidelines proposed or implemented to ensure responsible use of Explainable Artificial Intelligence.
Regulation/Guideline | Organization | Description |
---|---|---|
General Data Protection Regulation (GDPR) | European Union | Protects individuals’ data rights and demands explainability for automated decisions |
Algorithmic Accountability Act | United States Congress | Proposed legislation aiming to enhance transparency and accountability in AI systems |
Singapore Model AI Governance Framework | Infocomm Media Development Authority (IMDA) | Guidelines and suggestions for organizations to deploy trustworthy AI systems |
Table 10: XAI Future Trends
This table highlights some of the anticipated future trends in the field of Explainable Artificial Intelligence.
Trend | Description |
---|---|
Hybrid Approaches | Combining interpretability techniques from different models to achieve better performance |
Human-Interactive XAI | Enabling users to interactively explore and influence AI explanations |
Regulatory Standards | Development of stricter regulations and industry standards for explainable AI |
In conclusion, Explainable Artificial Intelligence (XAI) plays a crucial role in making AI systems more transparent, trustworthy, and accountable. Through the use of various techniques, algorithms, and libraries, XAI enables stakeholders to understand and interpret AI models and their decision-making processes. While XAI offers benefits such as transparency, accountability, and reduced bias, it also faces challenges, including maintaining accuracy and addressing human factors in interpretation. Regulatory efforts and guidelines are emerging to ensure the responsible deployment of XAI. As the field evolves, we can anticipate hybrid approaches, human-interactive XAI, and the establishment of stricter regulatory standards to shape the future of explainability in AI systems.
Frequently Asked Questions
Explainable Artificial Intelligence (XAI)
What is Explainable Artificial Intelligence (XAI)?
Explainable Artificial Intelligence (XAI) refers to the development of AI systems that can provide users with clear explanations of their decision-making process. XAI aims to increase transparency and enable humans to understand, trust, and effectively use AI technologies.
Why is explainability important in AI?
Explainability in AI is crucial for various reasons. It helps users, such as data scientists, regulators, and end-users, understand how and why AI systems make certain decisions. Explainability also allows for easier identification of biases and ethical concerns and increases trust in AI technology.
What are the benefits of Explainable AI (XAI)?
The benefits of XAI include improved transparency, accountability, and trust in AI systems. XAI also facilitates error diagnosis, enables faster model improvement, enhances human-AI collaboration, aids in compliance with regulations, and provides insights into the decision-making process.
How does XAI differ from traditional AI?
Traditional AI focuses on developing highly accurate AI models without emphasizing transparency or interpretability. In contrast, XAI places an emphasis on making AI more explainable and human-understandable without compromising accuracy.
What methods are employed in achieving explainability in AI?
Methods used for achieving explainability in AI include rule-based systems, decision trees, interpretable machine learning approaches, and model-agnostic techniques like LIME and SHAP. Each method aims to provide insights into the decision-making process based on different levels of interpretability.
Are there any downsides to XAI?
Although XAI offers numerous benefits, there are some potential downsides. Achieving explainability may require sacrificing some level of accuracy in AI models. Additionally, explainability methods can sometimes be complex, computationally expensive, and may require domain expertise to interpret and understand.
How is explainability being regulated in AI systems?
As AI grows in prominence, regulations surrounding explainability are emerging. For instance, the European Union‘s General Data Protection Regulation (GDPR) includes provisions for individuals’ right to explanation. Other regulatory bodies are also exploring guidelines to address the challenges and risks associated with non-explainable AI systems.
Can XAI be applied to all AI techniques?
While XAI can be applied to a variety of AI techniques and algorithms, the level of interpretability achieved may vary. Some AI models, such as deep neural networks, might be inherently less interpretable than others. However, efforts are being made to develop XAI methods applicable to a wide range of AI techniques.
Is XAI only important in high-stakes applications?
While explainability in AI is crucial in high-stakes applications like healthcare and finance, it is becoming increasingly important across various domains. Explainable AI can lead to better decision-making, identify bias, improve accountability, and enhance transparency in a wide range of AI applications.
What is the future of XAI?
The future of XAI holds immense potential. As AI technologies continue to advance, so will the methods and techniques for achieving explainability. XAI is expected to become an integral part of deploying AI systems, paving the way for responsible and trustworthy AI adoption across different industries.