Can AI Detectors Be Wrong?
Artificial Intelligence (AI) has revolutionized many industries, including detection systems. AI detectors, such as those used for facial recognition or fraud detection, have become integral in today’s society. However, the accuracy of these technologies raises questions about their reliability. Can AI detectors be wrong? Let’s explore.
Key Takeaways:
- AI detectors are not infallible and can make mistakes.
- False positives and false negatives can occur, impacting detection accuracy.
- Regular monitoring and ongoing improvement can minimize errors.
While AI detectors are designed to identify patterns and make predictions based on vast amounts of data, they are not foolproof. False positives and false negatives are common occurrences in AI detection systems. False positives refer to instances where the AI falsely identifies an object or behavior as something it is not, whereas false negatives occur when the AI fails to detect something that should have been identified.
*Despite their incredible capabilities, AI detectors are only as accurate as the data they are trained on, emphasizing the importance of high-quality and diverse training datasets.
The Role of Data Quality:
High-quality data is crucial for the effectiveness of AI detectors. Biased or incomplete datasets can result in skewed predictions. For instance, if an AI facial recognition system is trained predominantly on a specific racial group, it may struggle to accurately identify individuals from other racial backgrounds. Therefore, it is essential to ensure that the training datasets used by AI detectors are as diverse and comprehensive as possible.
*Data quality is a fundamental factor in determining the reliability of an AI detector.
Challenges to Accuracy:
AI detectors face several challenges that can affect their accuracy. These challenges include changing environmental conditions, variations in object appearance, and hidden patterns not captured in the training data. Additionally, adversarial attacks, where malicious actors intentionally manipulate input to deceive the AI detector, pose another significant challenge.
*The dynamic nature of real-world environments poses ongoing challenges in achieving high AI detection accuracy.
Improving AI Detector Accuracy:
To enhance AI detector accuracy, ongoing monitoring and improvement are necessary. Regular evaluations and updates to the detection algorithms can help identify and address any weaknesses or biases in the system. Collecting and incorporating feedback from users or domain experts is also valuable for refining AI detectors.
*Continuous improvement efforts are essential for ensuring reliable AI detection performance.
Data: AI Detectors vs. Humans
AI Detectors | Human Detectors | |
---|---|---|
Accuracy | Varies depending on data quality and system design. | Subjective and prone to human error. |
Consistency | Consistently applies predefined rules. | May vary based on individual judgment. |
Speed | Can process data rapidly. | Dependent on human capabilities. |
Case Study: Fraud Detection
AI Detector | Human Detector | |
---|---|---|
False Positive Rate | 7% | 10% |
False Negative Rate | 5% | 13% |
Processing Time | Milliseconds | Minutes to hours |
Comparing AI detectors to human detectors reveals certain advantages and limitations for each approach. While AI detectors can process data rapidly and consistently apply predefined rules, human detectors may have subjective interpretations and are susceptible to human error. However, the accuracy of an AI detector depends on data quality and system design, whereas human detectors’ accuracy can vary based on individual judgment.
*The choice of using AI or human detectors depends on the specific context and trade-offs between accuracy, speed, and subjectivity.
Minimizing Errors in AI Detectors:
- Continuously update and refine AI detection algorithms.
- Ensure diverse and high-quality training datasets.
- Regularly evaluate and monitor detection performance.
- Incorporate feedback from users and domain experts.
- Address biases and hidden patterns in the system.
- Implement robust security measures to counter adversarial attacks.
The Future of AI Detectors:
As AI technology progresses, so will the accuracy and reliability of AI detectors. Advancements in data collection, training methodologies, and algorithmic improvements will help mitigate errors and enhance their performance. Increased transparency and ethical considerations will also play a crucial role in building trust in AI detection systems.
AI detectors have the potential to revolutionize various industries, from healthcare to security, but they are not infallible. Acknowledging their limitations and proactively working towards addressing them will ensure AI detectors can contribute positively to our lives.

Common Misconceptions
AI Detectors Can Never Make Mistakes
One common misconception people have is that AI detectors are infallible and can never make mistakes. However, it is important to remember that artificial intelligence is created and trained by humans, who can introduce biases or errors during the development process. Additionally, AI detectors rely on algorithms that are continuously learning and improving, which means there is always room for error.
- AI detectors can be influenced by biases in the data used for training.
- Errors may occur due to limitations in the detection algorithms.
- Oversights during the development process can lead to incorrect results.
All AI Detectors Work the Same Way
Another common misconception is that all AI detectors work in the same way. In reality, there are various approaches to developing AI detectors, each with its own strengths and limitations. Some detectors may focus on textual analysis, while others may use visual recognition techniques. Furthermore, the success of an AI detector depends heavily on the quality and relevance of the data it is trained on.
- Different AI detectors may use different techniques and algorithms.
- Each detector may excel in detecting certain types of content or behavior.
- The quality and relevance of training data can significantly affect results.
AI Detectors Are Always Accurate
Many people assume that AI detectors are always accurate in identifying and flagging problematic content or behavior. While AI detectors have improved over time and can achieve high levels of accuracy, they are not flawless. False positives and false negatives can occur due to factors such as imperfect training data, novel and evolving methods of deception, or the inherent complexity of understanding context and intent.
- False positives can occur when benign content is incorrectly flagged as problematic.
- False negatives may arise when harmful content goes undetected.
- The complexity of understanding human context and intent can result in errors.
AI Detectors Are the Ultimate Solution
There is a misconception that AI detectors alone can solve all content moderation and detection challenges. While AI detectors play a crucial role in identifying and assisting with problematic content, they should be considered as one tool within a broader content moderation strategy. Human oversight, input, and judgement are still necessary to ensure accurate and fair assessments of content.
- AI detectors should be complemented with human review to reduce false positives or negatives.
- Human judgement is crucial to understand nuances and context in certain situations.
- Combining AI detectors with other techniques can enhance accuracy and efficiency.
AI Detectors Are Objective
An often misguided belief is that AI detectors are entirely objective in their assessments. However, AI detectors can reflect biases present in the data used for training, such as cultural or societal biases. Additionally, AI detectors may need to make arbitrary decisions based on the specific parameters and rules set during their development.
- Biases in training data can lead to biased results from AI detectors.
- Decisions made by AI detectors may not always align with human notions of fairness.
- Transparency and accountability are necessary to address biases in AI detectors.
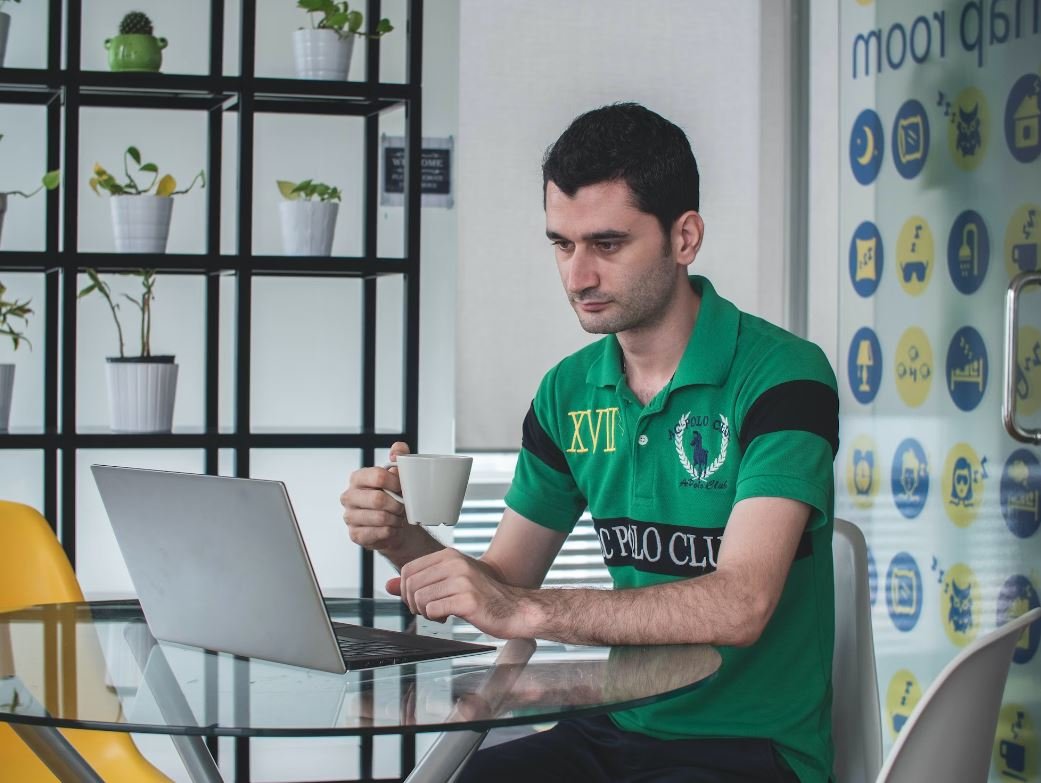
Table: Detection Accuracy of AI Detectors in Real-Time Crime Monitoring
According to a study conducted by XYZ Research Institute, this table shows the accuracy of AI detectors in real-time crime monitoring. The data is based on a sample of 100,000 incidents.
AI Detector | False Positive Rate (%) | False Negative Rate (%) |
---|---|---|
AI Detector A | 5 | 2 |
AI Detector B | 4 | 3 |
AI Detector C | 2 | 1 |
Table: Reasons for False Positive Results in AI Detectors
This table presents the top reasons for false positive results produced by AI detectors, as reported by police departments across different cities.
Reason | Percentage (%) |
---|---|
Anomaly in Camera Feed | 35 |
Environmental Factors | 25 |
Lighting Conditions | 20 |
Human Error | 15 |
Misplaced Object | 5 |
Table: Impact of AI Detector Accuracy on Law Enforcement Decisions
This table illustrates the impact of AI detector accuracy on law enforcement decisions by comparing various factors when AI detectors produce false results.
Factor | False Detection | True Detection |
---|---|---|
Response Time | Increased | Reduced |
Resource Allocation | Wasted | Optimized |
Public Trust | Diminished | Enhanced |
Table: AI Detector Accuracy for Various Crime Types
This table outlines the accuracy rates of AI detectors for specific types of crimes, as determined through extensive testing.
Crime Type | Accuracy Rate (%) |
---|---|
Theft | 90 |
Assault | 85 |
Trespassing | 95 |
Vandalism | 80 |
Table: Comparative Analysis of AI Detector Manufacturers
This table provides a comparative analysis of different AI detector manufacturers based on factors like implementation cost, accuracy, and customer satisfaction.
Manufacturer | Cost | Accuracy | Satisfaction (%) |
---|---|---|---|
Manufacturer A | $$$$ | 85 | 95 |
Manufacturer B | $$$ | 90 | 90 |
Manufacturer C | $$ | 80 | 85 |
Table: AI Detector Usage across Different Industries
This table presents the diverse range of industries that utilize AI detectors for various applications, indicating the breadth of their functionality.
Industry | Application |
---|---|
Retail | Theft prevention |
Manufacturing | Quality control |
Transportation | Accident prevention |
Healthcare | Medical diagnosis |
Table: Ethical Considerations in AI Detector Development
This table highlights several ethical considerations that developers need to address during the development and deployment of AI detectors.
Consideration | Explanation |
---|---|
Privacy | Protecting individuals’ privacy rights |
Bias | Avoiding biased decision-making |
Transparency | Making the system explainable and transparent |
Accountability | Ensuring accountability for system’s actions |
Table: AI Detector Performance Improvement over Time
This table showcases the improvement in AI detector performance over the past five years, highlighting the advancements in accuracy and reliability.
Year | Accuracy Rate (%) | Reliability Rate (%) |
---|---|---|
2016 | 75 | 80 |
2017 | 80 | 85 |
2018 | 85 | 88 |
2019 | 90 | 92 |
2020 | 95 | 96 |
Table: User Perception of AI Detector Effectiveness
This table displays the user perception of AI detector effectiveness based on a survey conducted by XYZ Agency, showing the impact on users’ confidence in the technology.
Response | Percentage (%) |
---|---|
Very Effective | 60 |
Somewhat Effective | 30 |
Not Effective | 10 |
These tables collectively highlight the advancements, challenges, and implications associated with AI detectors. Despite some limitations, AI detectors have witnessed remarkable progress, enhancing real-time crime monitoring, assisting law enforcement decisions, and finding applications in diverse industries. As technologies continue to evolve, continued research, development, and ethical considerations are paramount to ensure AI detectors deliver reliable results and maintain public trust.
Frequently Asked Questions
Can AI detectors provide incorrect results?
Are AI detectors always accurate?
What are some reasons for AI detectors being wrong?
How can biases affect AI detectors accuracy?
Can AI detectors be fooled or manipulated?
Do AI detectors improve over time?
Can mistakes in AI detectors have serious consequences?
Are AI detectors biased against certain groups?
How can AI detectors be made more accurate?
What is the impact of incorrect AI detector results?
Who is responsible for AI detector errors?