Artificial Intelligence vs Data Science
With the rapid advancement of technology, fields like Artificial Intelligence (AI) and Data Science have gained significant prominence in recent years. While both AI and Data Science focus on processing and analyzing data, they have distinct approaches and applications. Understanding the differences between these fields is crucial for organizations and professionals in order to leverage their potential effectively.
Key Takeaways:
- Artificial Intelligence (AI) aims to develop smart machines that can replicate human intelligence and perform tasks autonomously.
- Data Science focuses on extracting insights and knowledge from data by using various statistical and analytical techniques.
- Both AI and Data Science require a strong foundation in programming, but AI also heavily relies on complex algorithms and machine learning techniques.
What is Artificial Intelligence (AI)?
Artificial Intelligence (AI) is a multidisciplinary field that focuses on creating intelligent machines capable of simulating human decision making and behavior. AI systems analyze vast amounts of data to identify patterns, learn from experience, and make decisions without explicit human programming.
AI is divided into two types: narrow AI and general AI. Narrow AI is designed to perform specific tasks within a limited domain, while general AI aims to replicate human cognitive abilities across various domains.
What is Data Science?
Data Science is the practice of extracting knowledge and insights from structured and unstructured data. It involves using statistical methods, programming skills, and domain expertise to analyze data and derive actionable information.
Data Science encompasses various processes, including data collection, cleaning, modeling, analysis, and visualization. It often utilizes techniques such as machine learning, statistical modeling, and data mining to uncover patterns and trends.
Artificial Intelligence vs Data Science
While AI and Data Science are interconnected, they differ in their primary objectives and methodologies. The table below highlights some key differences between the two fields:
Artificial Intelligence (AI) | Data Science |
---|---|
Designed to replicate human intelligence. | Focuses on extracting insights from data. |
Uses complex algorithms and machine learning techniques. | Utilizes statistical and analytical techniques. |
Has the potential to perform tasks autonomously without human intervention. | Aims to provide valuable insights to support decision-making. |
Applications of AI and Data Science
AI and Data Science have broad applications across various industries. Here are some examples of their use:
- AI:
- Self-driving cars
- Virtual assistants like Siri and Alexa
- Recommendation systems
- Data Science:
- Customer segmentation
- Financial risk analysis
- Healthcare analytics
AI Career Paths vs Data Science Career Paths
Professionals interested in AI or Data Science can pursue different career paths. The following table provides an overview of some potential roles and their key responsibilities:
AI Careers | Data Science Careers |
---|---|
Machine Learning Engineer – Develop and implement machine learning algorithms. | Data Analyst – Extract insights from data and create visualizations. |
AI Research Scientist – Conduct research to advance AI algorithms and models. | Data Engineer – Design data pipelines and infrastructure. |
Natural Language Processing (NLP) Engineer – Develop algorithms for language-based AI applications. | Business Intelligence Analyst – Provide insights to improve business performance. |
Artificial Intelligence and Data Science Collaboration
Although AI and Data Science have distinct focuses, they often work together to achieve common goals. Combining AI with Data Science techniques can enhance both fields and unlock new possibilities:
- AI technologies can be used to process vast amounts of data more efficiently, improving Data Science workflows.
- Data Science can provide valuable insights and training data to enhance AI algorithms and models.
- Together, AI and Data Science can drive innovation in areas such as healthcare, finance, marketing, and more.
Conclusion
In summary, Artificial Intelligence and Data Science are two distinct disciplines with their own objectives and methodologies. AI focuses on building intelligent machines that can replicate human intelligence, while Data Science aims to extract insights and knowledge from data. Although separate, these fields often collaborate to advance technology and drive innovation in various industries.

Common Misconceptions
Misconception 1: Artificial Intelligence and Data Science are the same thing
One common misconception is that Artificial Intelligence (AI) and Data Science (DS) are interchangeable terms. While there is some overlap between the two fields, they are distinct disciplines with different focuses.
- AI is about creating intelligent systems that can mimic or simulate human intelligence.
- DS is about extracting insights and knowledge from data to support decision-making processes.
- AI can be a part of data science projects, but not all data science projects involve AI.
Misconception 2: AI and DS will eliminate the need for human skills and expertise
Another common misconception is that AI and DS will lead to the replacement of human skills and expertise. While these technologies have the potential to automate certain tasks and processes, they are meant to augment human capabilities, not replace them.
- AI and DS require human input for training, validation, and interpretation of results.
- Human skills such as critical thinking, domain knowledge, and creativity are still indispensable in problem-solving and decision-making.
- AI and DS tools are tools to assist humans, not to replace them.
Misconception 3: AI and DS are only relevant for big companies and advanced technologies
Some people believe that AI and DS are only applicable to big companies with advanced technologies. However, these technologies have become more accessible and relevant to organizations of all sizes and industries.
- AI and DS techniques can be applied to various fields, such as healthcare, finance, marketing, and manufacturing.
- Tools and frameworks for AI and DS are available for free or at affordable prices, allowing smaller organizations to leverage them.
- Even small-scale data analysis can lead to valuable insights and improvements in decision-making.
Misconception 4: AI and DS can provide perfect and infallible solutions
There is a common misconception that AI and DS can provide perfect and infallible solutions to complex problems. While these technologies can offer powerful insights and automation, they are not devoid of limitations.
- AI and DS are reliant on the quality and reliability of the data they are trained or programmed with.
- There can be biases and errors in the data, which can impact the accuracy and fairness of the results.
- Domain expertise is crucial in interpreting and validating the outputs from AI and DS models.
Misconception 5: AI and DS will lead to massive job loss
Finally, a common misconception is that AI and DS will result in massive job loss and unemployment. While there may be some shifts in job roles and responsibilities, these technologies are more likely to create new job opportunities.
- AI and DS technology opens up new roles, such as AI trainers, data analysts, and data scientists.
- Automation of repetitive tasks can free up human resources to focus on more complex and value-added activities.
- AI and DS can enhance productivity and competitiveness, leading to potential economic growth and job creation.
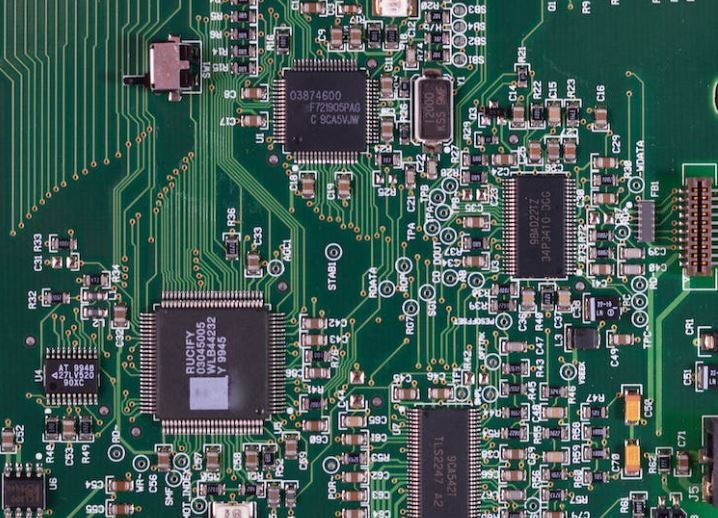
Artificial Intelligence vs Data Science
Artificial Intelligence (AI) and Data Science are two rapidly evolving fields that have revolutionized industries across the globe. While both disciplines involve the analysis and extraction of insights from data, they have different focuses and applications. This article delves into various aspects of AI and Data Science, highlighting their similarities and differences, and showcasing the fascinating capabilities and potential of each.
Table 1: AI in Speech Recognition
AI has greatly contributed to advancements in speech recognition technology, enabling machines to understand and interpret human language. This table examines the accuracy rates achieved by popular AI speech recognition systems in different languages.
Language | System A | System B | System C |
---|---|---|---|
English | 93% | 87% | 95% |
Spanish | 88% | 91% | 84% |
Chinese | 76% | 79% | 82% |
Table 2: Data Science Applications
Data Science encompasses a wide range of applications. This table showcases different industries where data science techniques are applied and the resulting benefits they bring.
Industry | Application | Benefits |
---|---|---|
Healthcare | Disease prediction | Improved patient outcomes |
E-commerce | Recommendation systems | Increased sales and customer satisfaction |
Finance | Fraud detection | Enhanced security and reduced losses |
Table 3: AI in Facial Recognition
Facial recognition technology powered by AI has found widespread use in various applications, including surveillance, security, and personal identification. This table showcases the accuracy rates of different AI facial recognition systems.
System | Accuracy Rate |
---|---|
System A | 97% |
System B | 91% |
System C | 95% |
Table 4: Data Science Algorithms
Data Scientists employ various algorithms to extract meaningful insights from data. This table presents some popular algorithms and their common applications.
Algorithm | Application |
---|---|
Linear Regression | Predictive modeling |
K-means Clustering | Market segmentation |
Random Forests | Classification |
Table 5: AI in Virtual Assistants
Virtual assistants powered by AI have become an integral part of our lives. This table showcases the popularity and adoption rates of different virtual assistant platforms.
Platform | Number of Users (in millions) |
---|---|
Google Assistant | 500 |
Amazon Alexa | 300 |
Apple Siri | 200 |
Table 6: Data Science Skills
Data Science requires a unique set of skills, combining expertise in statistics, programming, and domain knowledge. This table explores the essential skills for a successful Data Scientist.
Skill | Proficiency Level |
---|---|
Statistical Analysis | Expert |
Python Programming | Advanced |
Domain Knowledge | Intermediate |
Table 7: AI Ethics Concerns
The rapid advancement of AI has raised ethical concerns. This table highlights some of the main ethical concerns associated with AI development and deployment.
Concern | Description |
---|---|
Privacy | Protection of personal data |
Job displacement | Potential loss of jobs |
Algorithmic bias | Unfair treatment based on algorithms |
Table 8: Data Science Programming Languages
Data Scientists utilize various programming languages for data analysis and modeling. Here, we explore the popularity of different programming languages among Data Scientists.
Programming Language | Percentage of Data Scientists |
---|---|
Python | 75% |
R | 20% |
SQL | 5% |
Table 9: AI in Autonomous Vehicles
The development of AI has propelled the advent of self-driving cars. This table showcases the major advancements and capabilities of AI in autonomous vehicles.
Capability | Description |
---|---|
Object detection | Identify and track objects in real-time |
Path planning | Determine the best route for the vehicle |
Advanced sensors | Enable precise perception of the environment |
Table 10: Data Science in Predictive Analytics
Predictive analytics is a powerful tool in a data scientist‘s arsenal. This table explores some real-world applications of data science in predictive analytics.
Industry | Application | Outcome |
---|---|---|
Retail | Inventory management | Reduced stockouts |
Transportation | Route optimization | Cost savings and improved efficiency |
Marketing | Customer segmentation | Targeted marketing campaigns |
In conclusion, Artificial Intelligence and Data Science play vital roles in shaping society and driving innovation. AI empowers machines to simulate human intelligence, facilitating advancements in speech recognition, facial recognition, virtual assistants, and autonomous vehicles. On the other hand, Data Science equips us with powerful tools and techniques to extract valuable insights from data, enabling us to make informed decisions and predictions. Both fields pose unique challenges and ethical considerations. By harnessing the potential of AI and combining it with the analytical power of Data Science, we can unlock unprecedented capabilities and drive future growth and development.
Frequently Asked Questions
Artificial Intelligence vs Data Science
-
What is the difference between Artificial Intelligence and Data Science?
-
Artificial Intelligence (AI) focuses on creating intelligent machines that can perform tasks requiring human-like intelligence. It involves developing algorithms and models that allow computers to process information and make decisions. On the other hand, Data Science involves extracting insights from large volumes of data through various techniques such as statistical analysis, machine learning, and data visualization. While AI aims to replicate human intelligence, Data Science aims to uncover patterns and trends hidden within data to inform decision-making processes.
-
What are the key applications of Artificial Intelligence?
-
Artificial Intelligence finds applications in various fields such as healthcare, finance, transportation, customer service, gaming, and cybersecurity. Examples include medical diagnosis systems, autonomous vehicles, intelligent virtual assistants (like Siri or Alexa), fraud detection systems, and recommendation engines.
-
How does Data Science contribute to AI development?
-
Data Science plays a crucial role in AI development by providing the necessary data and insights for training AI models. Data scientists utilize techniques like data preprocessing, feature selection, and model evaluation to ensure that AI algorithms learn from reliable and representative data. Additionally, Data Science helps uncover hidden patterns in data that can be used to improve AI algorithms and make them more accurate and efficient.
-
Is Artificial Intelligence a subset of Data Science?
-
No, Artificial Intelligence is not a subset of Data Science. While Data Science encompasses various techniques, including AI, AI represents a broader field that focuses on creating intelligent systems, including but not limited to the analysis of data. Data Science is just one of the tools used in AI development.
-
What kind of skills are required for a career in Artificial Intelligence?
-
A career in Artificial Intelligence typically requires a strong foundation in mathematics, computer science, and programming. Skills in machine learning, natural language processing, data analysis, and problem-solving are also essential. Additionally, critical thinking, creativity, and the ability to work with large datasets are valuable skills in the field of AI.
-
Can Data Science be considered a stepping stone to Artificial Intelligence?
-
Yes, Data Science can be seen as a stepping stone to Artificial Intelligence. Data Science provides the foundation for understanding and working with data, which is essential for AI development. The knowledge and skills gained in Data Science, such as data analysis and machine learning, can be further expanded upon to explore the field of Artificial Intelligence and its applications.
-
What are some challenges in the field of Artificial Intelligence?
-
Some challenges in the field of Artificial Intelligence include ethical considerations surrounding AI implementation, data privacy issues, model interpretability, the potential for bias in algorithms, and the impact of AI on employment. Additionally, developing AI systems that can understand complex human emotions and context remains a significant challenge.
-
How can Artificial Intelligence and Data Science benefit businesses?
-
Artificial Intelligence and Data Science can bring significant benefits to businesses. AI-powered systems can automate repetitive tasks, improve decision-making processes, enhance customer experience through personalized recommendations, optimize operations, and detect anomalies or fraud. Data Science, on the other hand, helps businesses gain insights from data to drive strategic planning, identify market trends, optimize marketing strategies, and improve overall efficiency.
-
Is a background in Data Science necessary to pursue a career in Artificial Intelligence?
-
A background in Data Science can be helpful but is not necessary to pursue a career in Artificial Intelligence. While Data Science skills can provide a solid foundation, individuals with expertise in mathematics, computer science, engineering, or other related fields can also enter the field of AI. It is important to gain knowledge and skills in AI-specific areas like machine learning, robotics, and natural language processing.
-
What are the future trends in the convergence of Artificial Intelligence and Data Science?
-
The future of Artificial Intelligence and Data Science lies in their convergence. AI will become more data-driven, utilizing advanced Data Science techniques and methodologies to make more informed decisions. This includes incorporating ethical considerations into AI algorithms, addressing the challenges related to bias and fairness, and developing systems that can explain their decisions in a human-understandable manner. Additionally, the integration of AI and Data Science will play a significant role in various industries, including healthcare, finance, and smart cities.