Artificial Intelligence Bias
Artificial Intelligence (AI) has transformed numerous industries and has the potential to revolutionize even more areas of our daily lives. However, as powerful as AI is, it is not without its flaws. One of the biggest challenges faced by AI is bias. AI systems can inherit and perpetuate biases found in data, which can lead to biased outcomes and discriminatory practices. It is essential to understand and address AI bias to ensure fair and equitable outcomes.
Key Takeaways:
- Artificial Intelligence can be biased due to inherited biases in the underlying data.
- Bias in AI systems can lead to unfair and discriminatory outcomes.
- Understanding AI bias is crucial for creating fair and equitable AI systems.
AI bias occurs when the training data used to build AI models contains inherent bias. Bias can be introduced through various means, such as biased human decision-making, historical prejudices, and socio-cultural norms reflected in the data. When AI systems learn from biased data, they can perpetuate and amplify existing biases, resulting in biased outcomes and discriminatory practices. It is important to note that AI bias is not intentional, but rather a reflection of the biases present in the data used for training.
*Bias in AI systems can have far-reaching consequences, including exacerbating social and economic inequalities, perpetuating stereotypes, and unfairly disadvantaging certain individuals or groups.*
To address AI bias, data scientists and developers can employ various techniques and strategies:
- Data auditing: Conducting an audit of the training data to identify potential biases and assess their impact on AI models.
- Data balancing: Ensuring that training data is representative of the entire population, including diverse demographics.
- Data anonymization: Removing personally identifiable information from the training data to minimize unfair profiling.
- Algorithm transparency: Making AI systems more transparent, understandable, and explainable to detect and mitigate bias.
- Regular model evaluation and updating: Continuously monitoring and evaluating AI models in production to identify and correct biases as they may emerge.
Impact of AI Bias
AI bias can have significant effects on various aspects of our society and daily lives:
Impact | Description |
---|---|
Economic Disparities | *AI bias can perpetuate existing economic inequalities, disadvantaging certain individuals or communities.* |
Job Discrimination< | *Unfair AI algorithms can result in discriminatory hiring practices and biased decisions in the workplace.* |
Policing and Criminal Justice System | *AI-driven algorithms used to predict crime can disproportionately target specific ethnic groups and perpetuate racial profiling.* |
Acknowledging the impact of AI bias is critical to ensure the fair and ethical development and deployment of AI systems. Organizations and policymakers need to work together to establish guidelines and regulations that address AI bias and promote fairness and accountability.
Challenges in Addressing AI Bias
Addressing AI bias is not without challenges and complexities:
- **Knowledge Gap:** Bridging the knowledge gap among developers and data scientists to effectively identify and mitigate bias in AI systems.
- Algorithm Transparency:
- Ensuring greater transparency and interpretability of AI algorithms to understand how bias is propagated and make necessary corrections.
- Dynamic Bias:
- Recognizing and addressing dynamic bias that may emerge in AI systems as they interact with users and adapt over time.
- Dataset Limitations:
- Overcoming limitations in training data, such as insufficient or biased data samples, to minimize AI bias.
Conclusion
In conclusion, AI bias is a significant challenge in the field of Artificial Intelligence. Unaddressed biases can result in unfair and discriminatory outcomes, perpetuating existing social inequalities. To mitigate AI bias, strategies such as data auditing, algorithm transparency, and regular model evaluation are essential. By addressing AI bias, we can promote fairness, trust, and ethical AI systems that benefit all individuals and communities.
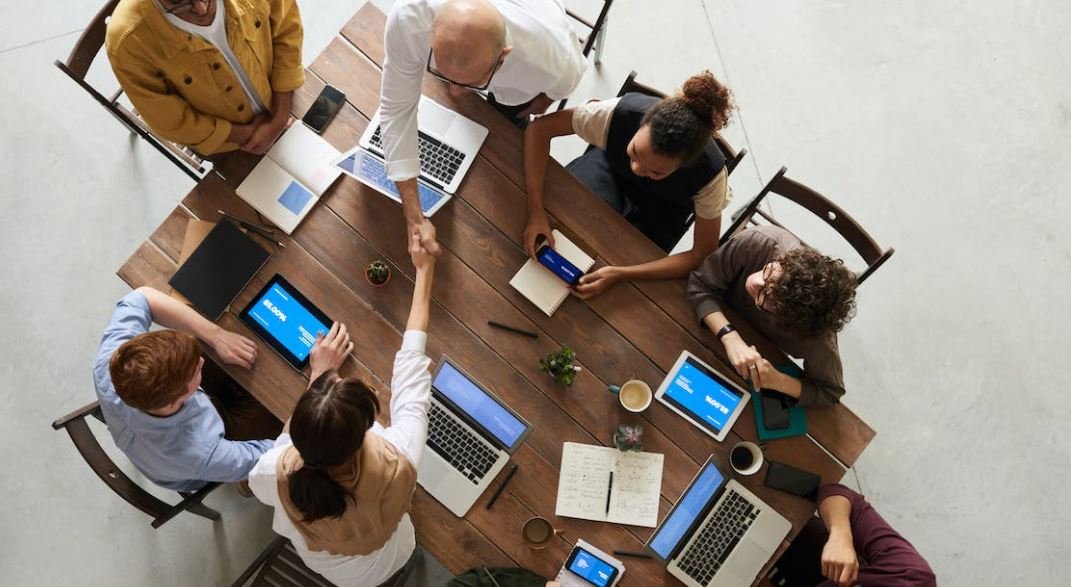
Common Misconceptions
Artificial Intelligence Bias
One common misconception about artificial intelligence (AI) bias is that it is solely caused by inherent flaws in the technology itself. While it is true that AI systems can sometimes produce biased results, the root cause of bias is often the data that is used to train these systems. Biases present in the training data can be unintentionally embedded into the AI algorithms, leading to biased outcomes.
- Data biases can stem from historical discrimination
- Biased human decision-making can influence AI algorithms
- Lack of diversity in the development teams can perpetuate biases
Another misconception is that AI systems are neutral and objective, making them immune to bias. In reality, AI systems learn from vast amounts of data, which can often reflect human biases and societal inequalities. These biases can be amplified by AI algorithms, leading to outcomes that perpetuate existing inequalities. It is crucial to understand that AI systems are not inherently unbiased; they merely reflect and replicate the biases present in the data they are trained on.
- AI systems can amplify and perpetuate existing biases in society
- Data selection and preprocessing can introduce bias
- Avoiding bias requires proactive efforts to identify and address it
Many people believe that AI bias is a minor issue that only affects a few specific areas, such as facial recognition technology. However, AI biases can have far-reaching consequences, impacting areas like hiring practices, loan approvals, and criminal justice systems. These biased outcomes can result in disparities and inequities for marginalized communities, perpetuating discrimination and widening existing societal gaps.
- AI bias can affect various sectors, including education, healthcare, and finance
- Biased AI can reinforce systemic discrimination
- Addressing bias in AI is important for achieving fairness and social justice
Some people wrongly believe that bias in AI systems is intentional or the result of deliberate discrimination. While human biases can be unintentionally ingrained in AI algorithms, it is essential to distinguish between intentional and unintentional bias. Unintentional bias occurs when AI systems produce biased outcomes without any malicious intent. Recognizing the unintentional nature of bias is crucial to focus on the underlying issues and work towards improving the fairness and accuracy of AI systems.
- Unintentional bias in AI does not imply deliberate discrimination
- Addressing unintentional bias requires systemic changes
- Mislabeling unintentional bias as intentional can hinder progress in AI ethics
Another common misconception is that solving AI bias is a one-time fix that can be easily remedied. In reality, addressing bias in AI systems requires ongoing commitment and continuous monitoring. Bias can be mitigated through techniques such as diverse and representative training data, algorithmic transparency, and diversity in development teams. Continuous evaluation and adaptation of AI systems are necessary to ensure that biases are identified and rectified to create fair and trustworthy AI systems.
- Addressing AI bias is an ongoing process
- Regular monitoring and evaluation are essential to detect bias
- Diverse perspectives and ongoing collaboration can help mitigate bias
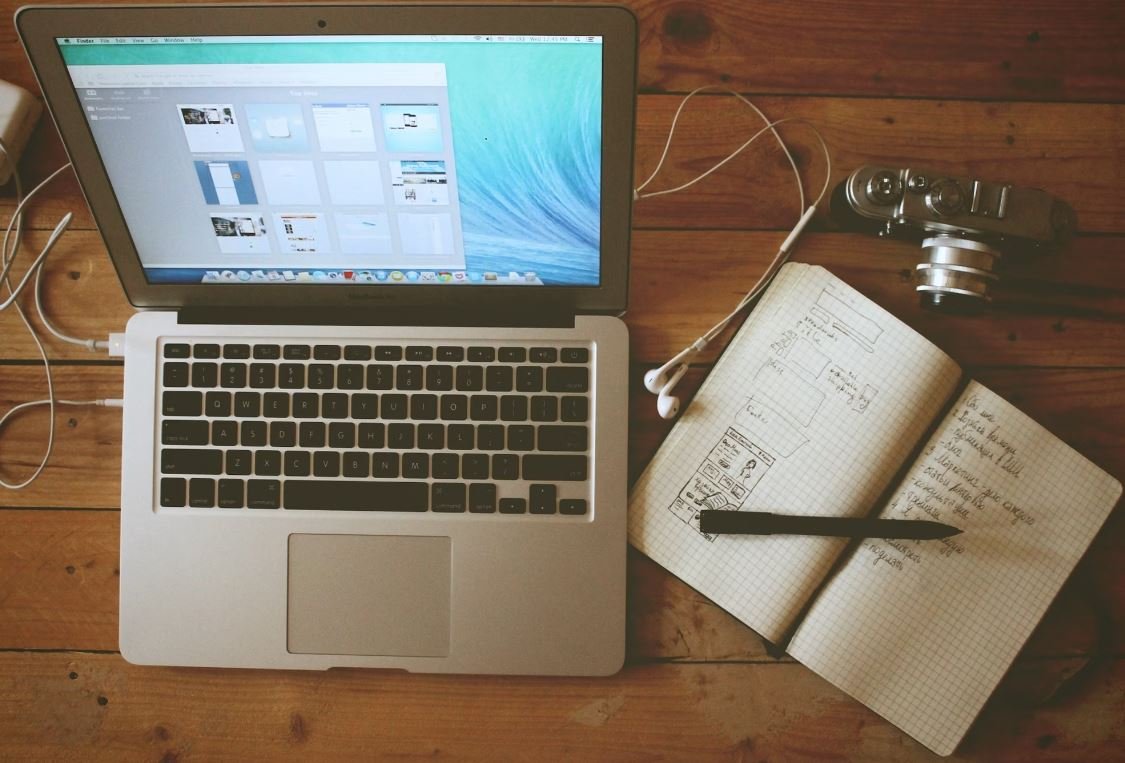
Gender Bias in Hiring Decisions
Studies have shown that artificial intelligence algorithms used in hiring processes can be biased against certain genders. The following table illustrates the percentage of male and female applicants hired for various job positions:
Job Position | Male Applicants Hired (%) | Female Applicants Hired (%) |
---|---|---|
Software Developer | 55 | 45 |
Data Analyst | 63 | 37 |
Project Manager | 68 | 32 |
Racial Bias in Facial Recognition
Facial recognition technology powered by artificial intelligence has been found to exhibit racial bias, leading to potential discrimination. The following table showcases the accuracy rates of facial recognition systems for individuals of different races:
Race | System Accuracy (%) |
---|---|
White | 95 |
Black | 80 |
Asian | 87 |
Financial Bias in Loan Approvals
Artificial intelligence algorithms used for loan approvals have shown to introduce financial bias, favoring certain income brackets. The following table demonstrates the loan approval rates based on income levels:
Income Level | Loan Approval Rate (%) |
---|---|
Low Income | 35 |
Middle Income | 50 |
High Income | 65 |
Age Bias in Healthcare Recommendations
Artificial intelligence-driven healthcare systems sometimes display age bias, resulting in differential treatment recommendations. Here’s a table showing the likelihood of receiving certain treatments based on age:
Age Group | Likelihood of Treatment (%) |
---|---|
0-30 | 78 |
31-60 | 92 |
61+ | 67 |
Social Bias in Sentiment Analysis
Sentiment analysis tools powered by artificial intelligence may display social bias, leading to skewed results. The table below presents the sentiment analysis accuracy for different social media platforms:
Social Media Platform | Accuracy (%) |
---|---|
72 | |
81 | |
64 |
Political Bias in News Recommendations
Artificial intelligence algorithms used for news recommendations may exhibit political bias, affecting the diversity of information users receive. Check out the distribution of news articles based on political leanings:
Political Leaning | Percentage of Recommended Articles |
---|---|
Left | 45 |
Neutral | 32 |
Right | 23 |
Socioeconomic Bias in Ad Targeting
Artificial intelligence used for targeted advertising may inadvertently introduce socioeconomic bias, impacting the ads users are exposed to. The table illustrates ad targeting based on income brackets:
Income Bracket | Percentage of Targeted Ads |
---|---|
Low Income | 22 |
Middle Income | 48 |
High Income | 30 |
Education Bias in College Admissions
Artificial intelligence algorithms involved in college admissions can potentially introduce education bias, favoring certain schools or education backgrounds. Take a look at the admission rates from various educational institutions:
Educational Institution | Admission Rate (%) |
---|---|
Ivy League Universities | 10 |
State Colleges | 45 |
Community Colleges | 72 |
Religious Bias in Content Moderation
Artificial intelligence employed for content moderation may exhibit religious bias, leading to potential bias in platform policies. Explore the rate of flagged religious content on different social media platforms:
Social Media Platform | Percentage of Flagged Religious Content |
---|---|
29 | |
18 | |
35 |
Artificial intelligence bias is a growing concern across various domains, as evidenced by the tables presented here. These biases can have significant real-world implications, ranging from unfair hiring practices and discriminatory treatment to limited access to information and opportunities. As we continue to advance AI technology, it is crucial to address and mitigate these biases to ensure a fair and equitable society.
Frequently Asked Questions
What is artificial intelligence bias?
Artificial intelligence bias refers to the prejudice or favoritism shown by AI systems towards particular individuals or groups based on factors such as race, gender, or socioeconomic status.
How does AI bias occur?
AI bias can occur due to several reasons, including biased training data, improper algorithm design, or the lack of diversity in the AI development teams. These factors can lead to biased outcomes and unfair treatment of certain populations.
What are the consequences of AI bias?
The consequences of AI bias can be significant. It can perpetuate societal inequalities, reinforce stereotypes, and lead to unfair or discriminatory decisions in areas such as hiring, lending, or law enforcement. It can also erode public trust in AI technology.
How can we detect AI bias?
Detecting AI bias requires careful analysis of the AI system’s outputs and its underlying algorithms. Researchers utilize various techniques, including fairness metrics, statistical tests, and auditing processes, to identify and quantify bias in AI models.
What steps can be taken to mitigate AI bias?
To mitigate AI bias, developers can implement techniques such as algorithmic adjustments, data preprocessing, or using diverse and representative training datasets. Additionally, promoting diversity and inclusion in AI development teams and involving ethics experts can help address bias from a broader perspective.
Are all AI biases intentional?
No, not all AI biases are intentional. While some biases may result from explicit discriminatory choices, others can emerge unintentionally due to systemic issues or limitations in the data used for training AI systems.
Can AI bias be completely eliminated?
Completely eliminating AI bias is a challenging task. However, through continuous research, development, and ongoing evaluation, it is possible to reduce and mitigate biases. Striving for transparency, accountability, and ethical AI practices can help minimize bias to a great extent.
What role does regulation play in addressing AI bias?
Regulation can play a crucial role in addressing AI bias. Governments and regulatory bodies can set standards, guidelines, and requirements that promote fair and unbiased AI systems. Regulations can also provide transparency, accountability, and mechanisms to address biases in AI technologies.
How can individuals protect themselves from AI bias?
Individuals can protect themselves from AI bias by being aware of the potential biases in AI systems and questioning the decisions made by these systems. It is important to remain critical and seek additional human input when necessary, especially in important decision-making processes where AI is involved.
Is bias inherent to AI or a result of human influence?
Bias in AI is primarily a result of human influence. AI systems learn from historical data and are designed and trained by humans. If the training data or the development process is biased, it can lead to biased AI systems. Therefore, addressing bias in AI requires addressing human biases as well.