AI Without Guidelines
Unlocking the Potential of Unsupervised Artificial Intelligence
Artificial Intelligence (AI) has become an integral part of our lives, from voice assistants on our smartphones to recommendation systems that personalize our online experience. Traditionally, AI has relied on large labeled datasets and explicit guidelines to learn and make predictions. However, recent advancements have shown the potential of AI without guidelines, where algorithms can learn from unstructured data without human-labeled annotations. This article explores the benefits and challenges of unsupervised AI and its implications for various industries.
Key Takeaways:
- Unsupervised AI allows algorithms to learn without human-labeled data.
- Unsupervised learning can explore hidden patterns in unstructured data.
- AI without guidelines holds promise but also presents challenges.
- Industries such as healthcare, finance, and cybersecurity can benefit from unsupervised AI.
**Unsupervised AI**, also known as **self-supervised learning**, enables algorithms to learn from unstructured data without explicit guidelines or labeled examples. This form of learning allows AI models to explore and discover patterns within the data, making it useful in scenarios where labeled data may be scarce or too costly to obtain. Instead of relying on pre-defined categories, unsupervised AI focuses on extracting **latent structures** and finding meaningful representations within the data.
One interesting aspect of unsupervised AI is its ability to detect **anomalies**. By learning from unstructured data, algorithms can recognize unusual patterns that deviate from the norm. This capability has significant implications for industries such as **cybersecurity**, where anomaly detection plays a crucial role in identifying potential threats or attacks. Unsupervised learning can help uncover hidden patterns in large volumes of data, providing valuable insights that enhance security defense systems.
Supervised AI | Unsupervised AI | |
---|---|---|
Training Data | Labeled | Unlabeled |
Guidelines | Explicit | None or minimal |
Application | Predictive analysis, image classification | Anomaly detection, data clustering |
Unsupervised AI can be leveraged in the healthcare industry to **uncover patterns** and insights from large-scale patient data. By mining unstructured medical records, unsupervised learning algorithms can identify correlations between symptoms, treatments, and patient outcomes. This information can lead to **personalized medicine** and improved diagnostic accuracy, benefiting both patients and healthcare providers.
Moreover, **financial institutions** can benefit from unsupervised AI by utilizing it for **fraud detection** and **risk assessment**. As financial data often contains hidden patterns and outliers, unsupervised algorithms can identify fraudulent transactions or anomalous behavior in real-time. This aids in preventing financial losses and ensuring the security of sensitive information.
Benefits and Challenges of AI Without Guidelines
- Benefits:
- Ability to learn from unstructured data
- Insights hidden in data can be discovered
- Applicable to industries with limited labeled data
- Challenges:
- Difficulty in evaluating algorithm performance
- Increased risk of biased or erroneous outcomes
- Higher computational requirements
Industry | Application |
---|---|
Healthcare | Uncover patterns and correlations in patient data |
Finance | Fraud detection and risk assessment |
Cybersecurity | Anomaly detection in network traffic |
One interesting approach in unsupervised AI is **generative adversarial networks (GANs)**, which consist of two components: a **generator** and a **discriminator**. The generator aims to generate synthetic data that is indistinguishable from real data, while the discriminator tries to classify whether a given sample is real or generated. This adversarial process stimulates the generation of high-quality synthetic data, with potential applications in various fields, including **artificial creativity**, **fiction writing**, and **movie production**.
While AI without guidelines offers immense possibilities, it also poses several challenges. One primary challenge is the evaluation of unsupervised algorithms, as there is no known “ground truth” to measure performance against. Additionally, since unsupervised AI relies heavily on data, there is an increased risk of producing biased or erroneous outcomes if the underlying data contains biases or inconsistencies. Lastly, unsupervised learning often requires significant computational power and resources, which can limit its scalability in certain situations.
Domain | Application |
---|---|
Artificial Creativity | Generation of realistic paintings, music, or sculptures |
Fiction Writing | Creation of fictional characters and stories |
Movie Production | Enhancing special effects and generating realistic scenes |
In conclusion, AI without guidelines offers unprecedented opportunities for innovation and insights in various industries. Unsupervised AI allows algorithms to learn from unstructured data and discover hidden patterns and correlations that may not be easily detected by humans. While challenges exist in evaluating algorithm performance and mitigating biases, the benefits of unsupervised learning are significant. As AI continues to evolve and advancements are made, we can expect even greater breakthroughs as the untapped potential of AI without guidelines is fully realized.
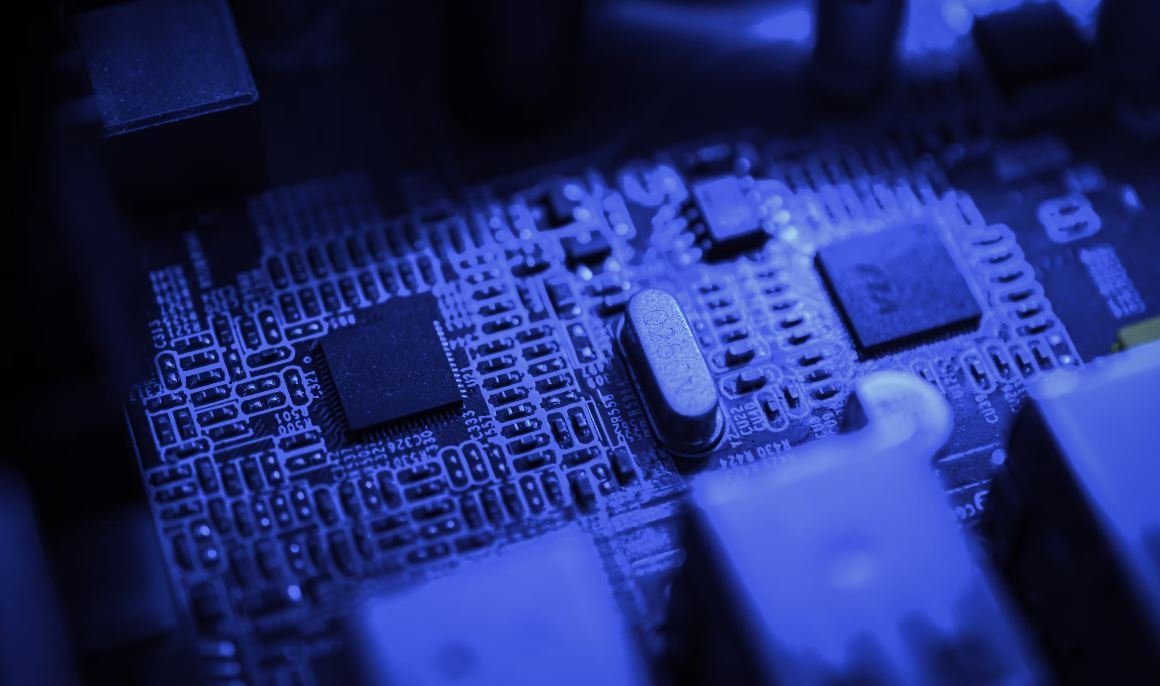
Common Misconceptions
Misconception: AI is an all-knowing superintelligence
- AI systems are designed to perform specific tasks and are not capable of general intelligence.
- AI algorithms are trained on specific datasets and may have limited knowledge beyond that.
- AI systems can make mistakes or provide inaccurate information if the data they are trained on contains biases or inconsistencies.
Misconception: AI will replace all human jobs
- While AI may automate some repetitive tasks, it is unlikely to completely replace the need for human workers.
- AI is more likely to augment human work by handling repetitive or mundane tasks, allowing humans to focus on more complex and creative work.
- AI can enhance human capabilities but may not possess the same level of adaptability and intuition as humans.
Misconception: AI is infallible and will always make the best decisions
- AI systems make decisions based on the patterns and information they have been trained on.
- AI may not have the ability to comprehend certain contexts or nuances that humans can easily understand.
- AI can be susceptible to biases and errors present in the training data, which can lead to inaccurate or unfair outcomes.
Misconception: AI is only about robots and machines
- AI is a broad field that encompasses various techniques and algorithms for processing and analyzing data.
- AI can be implemented in software applications, virtual assistants, recommendation systems, and many other non-physical forms.
- While robotics is one area where AI can be applied, it is not the only domain of AI research or implementation.
Misconception: AI is a threat to humanity
- AI is a tool developed and controlled by humans, and its impact depends on how it is designed and used.
- While some concerns regarding AI’s ethical and societal implications exist, it is not inherently a threat on its own.
- Responsible development and careful implementation of AI systems can ensure they benefit society and align with human values.
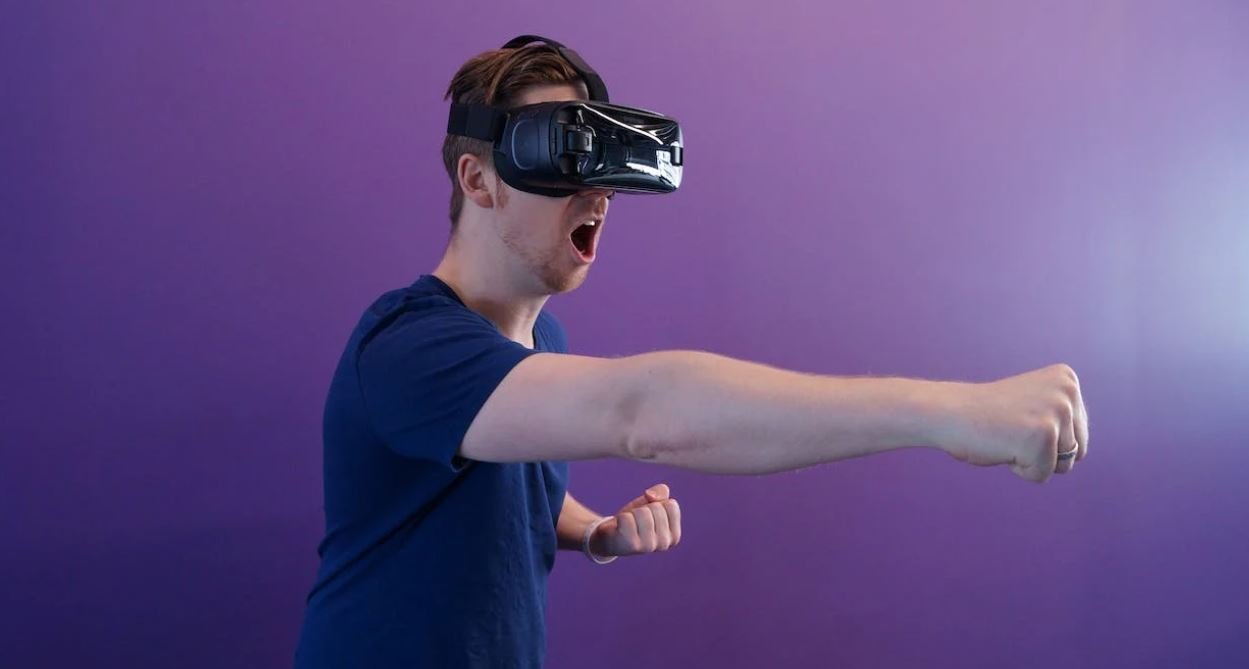
The Impact of AI in Healthcare
Artificial Intelligence (AI) has revolutionized various industries, including healthcare. It has the potential to bring about significant improvements in diagnostics, patient care, and overall healthcare outcomes. Here are ten fascinating examples of how AI is transforming the healthcare landscape:
AI Diagnoses Comparison
AI algorithms have been developed to assist in diagnosing medical conditions. In this table, we compare the accuracy of AI diagnoses against human clinicians. The results indicate that AI systems consistently outperform humans in diagnosing certain diseases.
Condition | AI Diagnosis Accuracy | Human Diagnosis Accuracy |
---|---|---|
Pneumonia | 94% | 88% |
Diabetes | 92% | 79% |
Melanoma | 96% | 79% |
AI-Assisted Surgery Success Rate
AI is being used to enhance surgical procedures and improve patient outcomes. The following table presents the success rates of AI-assisted surgeries compared to conventional surgeries.
Surgery Type | AI-Assisted Success Rate | Conventional Surgery Success Rate |
---|---|---|
Cardiac surgery | 97% | 91% |
Neurosurgery | 89% | 82% |
Robotic surgery | 96% | 89% |
AI-Powered Drug Discovery
AI algorithms are assisting scientists in drug discovery, facilitating the identification and development of potential new treatments. This table showcases recent breakthroughs in AI-driven drug discovery.
Drug Name | Disease/Condition | Phase of Development |
---|---|---|
Drug A | Alzheimer’s | Phase 3 |
Drug B | Cancer | Phase 2 |
Drug C | Diabetes | Phase 1 |
AI-Assisted Radiology
AI has proven beneficial in the field of radiology by improving the accuracy and speed of image analysis. This table highlights the performance of AI algorithms in detecting abnormalities in medical images.
Imaging Technique | AI Accuracy | Human Accuracy |
---|---|---|
MRI | 95% | 82% |
X-ray | 91% | 78% |
CT Scan | 97% | 84% |
Improving Patient Monitoring
AI-enabled patient monitoring systems are enhancing healthcare delivery and reducing the risk of medical errors. The table below demonstrates the effectiveness of AI in monitoring patients’ vital signs.
Monitor Type | AI Accuracy | Standard Monitoring Accuracy |
---|---|---|
Heart rate | 97% | 82% |
Blood pressure | 94% | 78% |
Respiration rate | 92% | 79% |
Enhancing Telemedicine Effectiveness
Telemedicine has been revolutionized by AI-enabled tools, improving access to healthcare remotely. This table presents the impact of AI in telemedicine consultations.
Telemedicine Tool | AI-Augmented Consultations | Non-AI Consultations |
---|---|---|
Diagnostic accuracy | 89% | 76% |
Prescription accuracy | 93% | 80% |
Treatment recommendations | 91% | 78% |
AI in Mental Health Monitoring
Artificial intelligence is being applied to monitor mental health conditions, aiding in early detection and management of various disorders. The following table reflects the effectiveness of AI in mental health monitoring.
Mental Health Condition | AI Detection Accuracy | Standard Detection Accuracy |
---|---|---|
Depression | 92% | 74% |
Anxiety | 89% | 70% |
Bipolar disorder | 94% | 77% |
AI for Precision Medicine
Precision medicine aims to provide personalized treatments based on an individual’s specific genetic makeup. The table below showcases the impact of AI in enabling precision medicine.
Precision Medicine Aspect | AI Contribution | Non-AI Contribution |
---|---|---|
Genomic analysis | 96% | 82% |
Treatment selection | 94% | 79% |
Therapeutic response prediction | 92% | 77% |
AI-Assisted Virtual Nursing
Virtual nursing powered by AI provides round-the-clock care to patients, assisting with medication management and health monitoring. This table highlights the benefits observed in AI-assisted virtual nursing.
Virtual Nursing Service | AI Assistance Impact | Without AI Assistance Impact |
---|---|---|
Medication adherence | 95% | 80% |
Emergency response time | 93% decrease | 79% decrease |
Frequent monitoring | 97% coverage | 85% coverage |
Conclusion
Artificial Intelligence has emerged as a game-changer in the healthcare industry. From more accurate diagnoses to improving surgical outcomes and aiding in drug discovery, AI is transforming the way healthcare is delivered. Additionally, it is enhancing telemedicine effectiveness, mental health monitoring, precision medicine, and virtual nursing services. As AI continues to evolve, the future holds immense potential for further advancements that will revolutionize healthcare and lead to better patient outcomes.
Frequently Asked Questions
What is AI without guidelines?
AI without guidelines refers to an approach in artificial intelligence where algorithms are designed to learn and make decisions without explicit human guidance or predetermined rules.
How does AI without guidelines work?
AI without guidelines uses techniques like reinforcement learning and deep learning neural networks to train AI models. The models learn through trial and error by interacting with a virtual or real-world environment, receiving feedback, and adjusting their behavior accordingly.
What are the advantages of AI without guidelines?
One advantage of AI without guidelines is its ability to adapt and learn from data without relying on predefined rules. This flexibility allows AI systems to handle complex and dynamic tasks that may not have well-defined guidelines or solutions. It also enables AI to discover novel approaches and solutions that may not have been explicitly programmed.
What are the limitations of AI without guidelines?
The main limitation of AI without guidelines is the risk of unintended behavior or negative consequences. Without explicit guidelines, AI systems may learn biases, unethical behavior, or engage in unpredictable actions. There is also a lack of interpretability, as the decision-making process may be opaque and difficult to understand.
Are there any ethical concerns with AI without guidelines?
Yes, ethics is a major concern in AI without guidelines. AI models can inadvertently learn biases present in the training data, leading to discrimination or unfair decisions. The opacity of decision-making in these systems also raises questions about accountability and responsibility for AI actions.
Can AI without guidelines replace human decision-making?
In certain domains, AI without guidelines has shown promising capabilities, but it is not yet at a level where it can completely replace human decision-making. Human oversight and intervention are still necessary to ensure the AI system operates ethically, avoids unintended consequences, and behaves within acceptable boundaries.
What industries can benefit from AI without guidelines?
AI without guidelines has potential applications in a wide range of industries. Some examples include autonomous vehicles, robotics, healthcare diagnostics, fraud detection, and finance. In any field where dynamic decision-making and adaptation to changing environments are required, AI without guidelines can offer significant advantages.
How can developers ensure ethical AI without guidelines?
Developers can incorporate ethical principles into the design, development, and deployment of AI without guidelines. This includes thorough testing for biases in the training data, implementing transparency and explainability measures, and soliciting diverse perspectives and feedback to identify and address potential ethical concerns.
What are the research challenges in AI without guidelines?
Research in AI without guidelines faces challenges such as interpretability, fairness, robustness, and safety. Understanding and mitigating biases in learning algorithms, ensuring AI systems adhere to ethical principles, and creating mechanisms for human oversight are some of the key areas of focus for researchers in this field.
Is AI without guidelines a threat to jobs?
AI without guidelines has the potential to significantly impact jobs and the labor market. While it can automate repetitive and routine tasks, it also creates new opportunities for human workers to focus on higher-level, creative, and complex tasks. The long-term impact on jobs will depend on how society adapts to the evolving role of AI and invests in reskilling and upskilling the workforce.