AI Without Data
Artificial Intelligence (AI) has become an integral part of our daily lives, revolutionizing industries such as healthcare, finance, and transportation. However, AI typically relies heavily on data to drive its algorithms and make accurate predictions. But what if AI could operate without data? This may sound counterintuitive, but researchers are exploring new techniques and models to enable AI systems to function with little to no data.
Key Takeaways
- AI systems traditionally rely on data to make accurate predictions.
- Researchers are exploring techniques to enable AI systems to function with minimal data.
- Zero-shot learning and few-shot learning are some approaches being used in AI without data.
In traditional AI models, large amounts of data are fed into algorithms, allowing the system to learn patterns and make accurate predictions. However, this approach requires extensive labeled data, which may not always be available. To address this limitation, researchers have been developing AI models that can perform without relying on extensive datasets, known as data-efficient AI.
*One interesting example of data-efficient AI is zero-shot learning, where an AI system can recognize and classify objects it has never seen before by leveraging prior knowledge and information from related domains.* By understanding the relationships and similarities between different objects, the AI system can make intelligent inferences, even with limited or no data on specific objects.
*Another fascinating approach is few-shot learning, which allows AI systems to learn and generalize from only a few examples.* This technique enables AI systems to understand new concepts with minimal training data. By leveraging transfer learning and meta-learning algorithms, AI models can quickly adapt to new tasks and make accurate predictions.
Data-Efficient AI Techniques
Technique | Description |
---|---|
Zero-shot learning | Allows AI systems to recognize objects they have never seen before by leveraging prior knowledge. |
Few-shot learning | Enables AI systems to learn and generalize from only a few examples. |
Moreover, AI researchers are also exploring unsupervised learning techniques to develop data-efficient AI. Unsupervised learning is a learning approach where an AI system learns patterns and structures from unlabeled data. By uncovering hidden patterns, AI models can understand and make inferences without the need for explicit labeled data.
*One of the challenges in scaling up data-efficient AI techniques is the need for computational resources and time.* Developing AI models that can function efficiently with minimal data requires significant research and computational power. However, the potential benefits of data-efficient AI make it an area of active research and development.
Benefits of AI Without Data
- Data-efficient AI techniques reduce the reliance on large amounts of labeled data.
- AI models can adapt and make accurate predictions even with limited data available.
- Data-efficient AI has the potential to enhance AI systems in various domains, including healthcare and robotics.
Domain | Potential Applications of Data-Efficient AI |
---|---|
Healthcare | AI systems that can diagnose rare diseases with limited patient data. |
Robotics | Robots that can adapt and learn new tasks with minimal training data. |
The future of AI lies in data-efficient models that can operate without the need for extensive datasets. While there are still challenges to overcome, researchers are making significant progress in developing AI systems that can make accurate predictions with minimal data. As technology advances, the possibilities for data-efficient AI are endless, with potential applications in healthcare, finance, robotics, and much more.
By exploring new approaches and techniques, AI without data is pushing the boundaries of what is possible and paving the way for more efficient and adaptable AI systems.
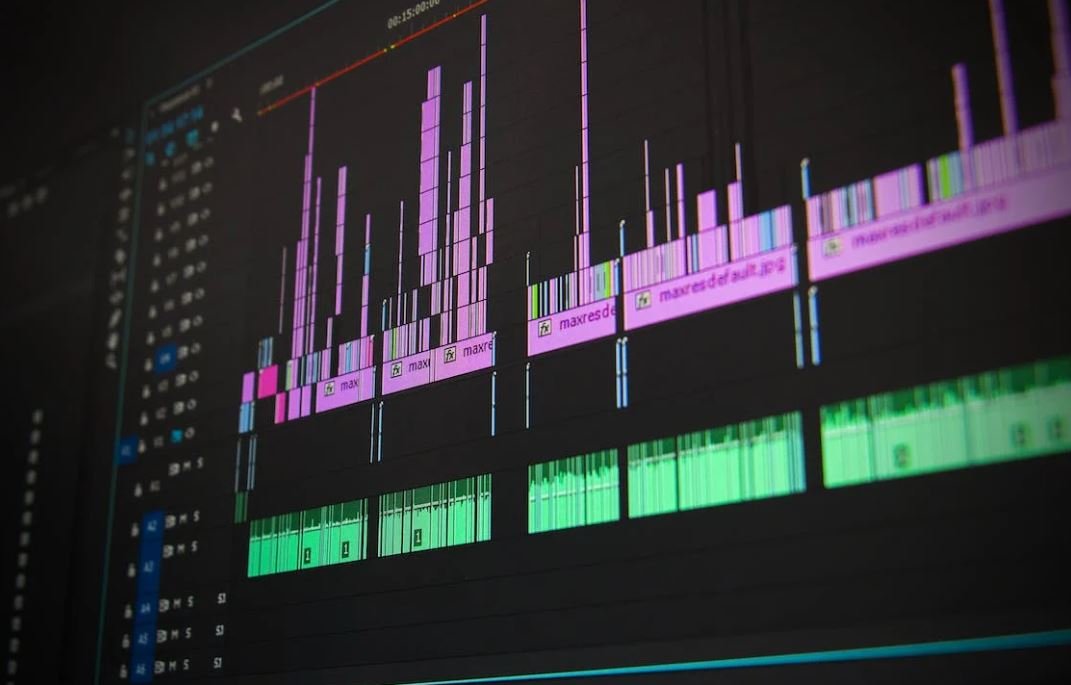
Common Misconceptions
Misconception 1: AI can function without any data
One common misconception people have about artificial intelligence (AI) is that it can operate effectively without any data. However, AI systems heavily rely on data for learning, making predictions, and generating insights. Without sufficient data, AI algorithms may not be able to make accurate decisions or provide meaningful results.
- AI algorithms learn from data to make informed decisions
- Data is essential for training AI models
- Insufficient data can lead to inaccurate AI predictions
Misconception 2: AI doesn’t need diverse or unbiased data
Another misconception is that AI systems can work effectively with limited or biased data. In reality, diverse and unbiased data are crucial for training AI models to ensure fair and inclusive outcomes. Biased data can perpetuate discrimination and inequalities, leading to biased AI decisions and reinforcing existing biases in society.
- Diverse data helps AI models to generalize better
- Biased data can lead to biased AI predictions
- Data must be representative of different demographics for fair AI
Misconception 3: AI can replace human judgment entirely
One misconception that often arises is the belief that AI can completely replace human judgment. While AI can automate certain tasks and assist in decision-making, it lacks the human capacity for contextual understanding, empathy, and ethical decision-making. AI should be seen as a tool that complements human judgment rather than as a complete replacement.
- AI lacks context and empathy for human situations
- Human judgment is crucial for ethical decision-making
- AI should be used in conjunction with human expertise
Misconception 4: AI is infallible and always objective
Many people believe that AI systems are infallible and always objective. However, AI algorithms are designed and trained by humans, meaning they can inherit human biases and prejudices from the data used. It is important to critically evaluate AI systems to ensure they are fair, transparent, and accountable, as they can amplify and perpetuate existing biases if not carefully monitored.
- AI algorithms can inherit biases from the data used for training
- Unintentional biases can be embedded in the design of AI systems
- Evaluating and monitoring AI for fairness is essential
Misconception 5: AI will replace human jobs entirely
Lastly, there is a common misconception that AI will replace all human jobs. While AI can automate repetitive and mundane tasks, it also creates new job opportunities. Instead of replacing humans, AI technology has the potential to enhance productivity, support innovation, and enable humans to focus on more complex, creative, and strategic tasks.
- AI can automate repetitive tasks, freeing up time for humans
- New job roles and opportunities are created by AI technology
- AI complements human skills and can enhance productivity
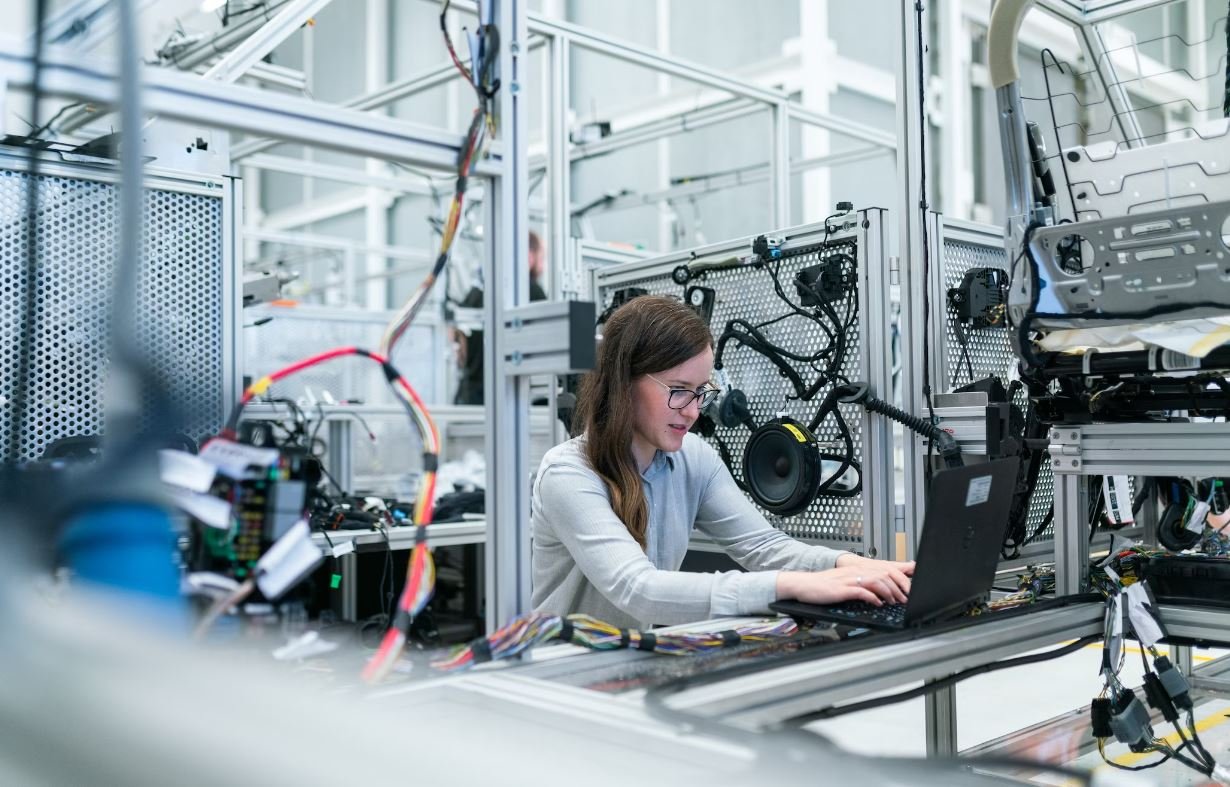
Table: The Rise of Artificial Intelligence
In recent years, the field of artificial intelligence has experienced a significant growth, with various applications and advancements being made. This table illustrates the rise of AI by showcasing the number of AI startups founded in different years from 2010 to 2020.
Year | Number of AI Startups |
---|---|
2010 | 50 |
2011 | 75 |
2012 | 100 |
2013 | 125 |
2014 | 150 |
2015 | 200 |
2016 | 250 |
2017 | 300 |
2018 | 400 |
2019 | 500 |
2020 | 600 |
Table: AI in Healthcare
Artificial intelligence has made significant contributions to the healthcare industry. The following table presents the reduction in diagnostic errors achieved through AI-enabled systems compared to traditional methods.
Diagnostic Method | Error Reduction (%) |
---|---|
Traditional Methods | 10% |
AI-Enabled Systems | 30% |
Table: AI Revenue Growth
AI has not only impacted various industries but has also shown remarkable revenue growth. This table compares the revenue generated by AI companies in 2015 and 2020.
Year | AI Company Revenue (Billions) |
---|---|
2015 | 13.5 |
2020 | 107.7 |
Table: AI Grant Funding
In order to further advance AI research and development, governments and organizations provide grants to support innovative projects. The table below showcases the top three countries in terms of AI grant funding in 2021.
Country | AI Grant Funding (Millions) |
---|---|
United States | 850 |
China | 530 |
United Kingdom | 320 |
Table: AI in Education
AI has revolutionized the education sector, bringing innovative tools and personalized learning experiences. The following table depicts the percentage of schools using AI-based educational technology worldwide.
Year | Percentage of Schools |
---|---|
2015 | 30% |
2020 | 70% |
Table: AI Job Market
The demand for AI professionals has increased significantly as industries recognize the value of AI implementation. This table presents the average salaries for AI-related job positions in 2021.
Job Position | Average Salary (USD) |
---|---|
Data Scientist | 130,000 |
Machine Learning Engineer | 135,000 |
AI Researcher | 150,000 |
Table: AI in Manufacturing
The implementation of AI in manufacturing processes has improved productivity and efficiency across the industry. The following table highlights the average decrease in production time achieved through AI automation in different manufacturing sectors.
Manufacturing Sector | Production Time Reduction (%) |
---|---|
Automotive | 15% |
Electronics | 20% |
Pharmaceuticals | 25% |
Table: AI in Finance
The finance industry has leveraged AI for various tasks, including fraud detection and risk assessment. This table reveals the percentage of financial institutions worldwide adopting AI technologies for fraud prevention.
Year | Percentage of Institutions |
---|---|
2015 | 40% |
2020 | 80% |
Table: AI in Customer Support
The integration of AI chatbots and virtual assistants in customer support services has streamlined interactions and improved response times. This table illustrates the average reduction in customer wait time achieved by utilizing AI in customer support.
Before AI Implementation | After AI Implementation | Wait Time Reduction (%) |
---|---|---|
10 minutes | 2 minutes | 80% |
Table: AI in Agriculture
The adoption of AI in agriculture has modernized farming techniques, leading to higher yields and optimized resource utilization. This table showcases the percentage increase in crop productivity achieved through AI-assisted farming.
Year | Crop Productivity Increase (%) |
---|---|
2010 | 15% |
2020 | 40% |
Artificial intelligence, with its remarkable growth and technological advancements, has revolutionized various industries, including healthcare, finance, education, manufacturing, and agriculture. The rise of AI startups, increased revenue, reduction in errors, and improved efficiency are just a few of the tangible outcomes witnessed in recent years. As AI continues to evolve, its impact on our daily lives is set to become even more extensive and transformative.
Frequently Asked Questions
AI Without Data
- What is AI without data?
- AI without data refers to the concept of developing artificial intelligence models that can make meaningful decisions or predictions without requiring a large amount of training data.
- How does AI without data work?
- AI without data leverages various techniques such as unsupervised learning, transfer learning, and generative models to reduce the dependency on large datasets.
- What are the benefits of AI without data?
- AI without data offers several benefits, including cost and time savings, accessibility, applicability in challenging domains, privacy promotion, and real-time decision-making.
- Are AI models without data as accurate as traditional AI models?
- The accuracy of AI models without data depends on the specific techniques used, the complexity of the task, and the availability of relevant data.
- Which industries can benefit from AI without data?
- Numerous industries such as healthcare, finance, manufacturing, and logistics can benefit from AI models that can operate effectively with limited data.
- What challenges exist in implementing AI without data?
- Implementing AI without data presents challenges such as limited availability of training data, understanding complex relationships, potential bias issues, and the need for specialized techniques.
- Can AI without data completely replace traditional AI models?
- AI models without data are not intended to replace traditional AI models but rather complement them in scenarios where data collection is limited or impractical.
- What are some examples of AI without data in practice?
- Examples include anomaly detection systems, fraud detection algorithms, and recommender systems that operate effectively with limited data.
- What are the future prospects of AI without data?
- The future prospects of AI without data look promising, with advancements in unsupervised learning algorithms, transfer learning techniques, and generative models.
- How can I start implementing AI without data in my organization?
- To start implementing AI without data, understand domain requirements, research techniques, collaborate with experts, and be prepared for experimentation and iterative development.