AI vs Data Science
Artificial Intelligence (AI) and Data Science are two rapidly evolving fields that have gained significant attention in recent years. While they are often used interchangeably, AI and Data Science are distinct disciplines with different focuses and applications.
- AI and Data Science are distinct fields with different focuses.
- AI aims to create intelligent machines that can mimic human behavior and perform tasks without explicit instructions.
- Data Science is concerned with extracting actionable insights from vast amounts of structured and unstructured data.
- The two fields overlap in certain areas, with AI utilizing data science techniques to enhance its capabilities.
- Both AI and Data Science have a wide range of applications across various industries.
AI and Its Applications
AI refers to the simulation of human intelligence in machines that are programmed to think and learn like humans. It encompasses a range of techniques and technologies, including machine learning, natural language processing, and computer vision, among others. AI is employed in various ways and has numerous applications across different sectors, such as:
1. Robotics: AI plays a crucial role in the development of advanced robots that can perform complex tasks autonomously.
2. Virtual Assistants: AI powers virtual assistants like Siri and Alexa, which can understand and respond to natural language queries.
3. Healthcare: AI is used for medical imaging analysis, drug discovery, personalized treatment plans, and disease diagnosis.
AI has the potential to revolutionize industries by automating mundane tasks and augmenting human capabilities.
Data Science and Its Significance
Data Science is an interdisciplinary field that combines statistical analysis, machine learning, and domain knowledge to extract meaningful insights and make data-driven decisions. It encompasses various stages, including data cleaning, exploration, modeling, and interpretation. Some key applications of Data Science include:
1. Predictive Analytics: Data Science enables organizations to forecast future outcomes and make informed decisions based on historical data.
2. Fraud Detection: Data Science techniques are utilized to identify patterns and anomalies in large datasets to detect fraudulent activities.
3. Recommendation Systems: Companies employ Data Science algorithms to provide personalized recommendations to users based on their preferences and behavior.
Data Science is transforming businesses by uncovering hidden patterns, optimizing processes, and driving innovation.
A Comparison: AI vs. Data Science
AI | Data Science | |
---|---|---|
Focus | Building intelligent machines that mimic human behavior. | Extracting actionable insights from data to drive decision-making. |
Techniques | Machine learning, natural language processing, computer vision. | Statistical analysis, machine learning, data cleaning. |
Applications | Robotics, virtual assistants, healthcare. | Predictive analytics, fraud detection, recommendation systems. |
AI | Data Science | |
---|---|---|
Overlap | AI techniques utilize Data Science methodologies to enhance capabilities. | Data Science techniques can be applied within AI models for data analysis. |
Industry Impact | Automation, augmented human capabilities, improved customer experiences. | Data-driven decision-making, efficiency improvements, innovation. |
Skills | Proficiency in machine learning, natural language processing, and algorithm development. | Strong statistical analysis, programming, and domain knowledge. |
A better understanding of both AI and Data Science helps organizations leverage their capabilities effectively.
Wrapping Up
AI and Data Science are complementary disciplines that have the potential to revolutionize industries and drive innovation. While AI focuses on building intelligent machines that mimic human behavior, Data Science extracts valuable insights from vast amounts of data. The overlap between the two fields enhances their capabilities, and organizations can leverage both to gain a competitive edge in today’s data-driven world.
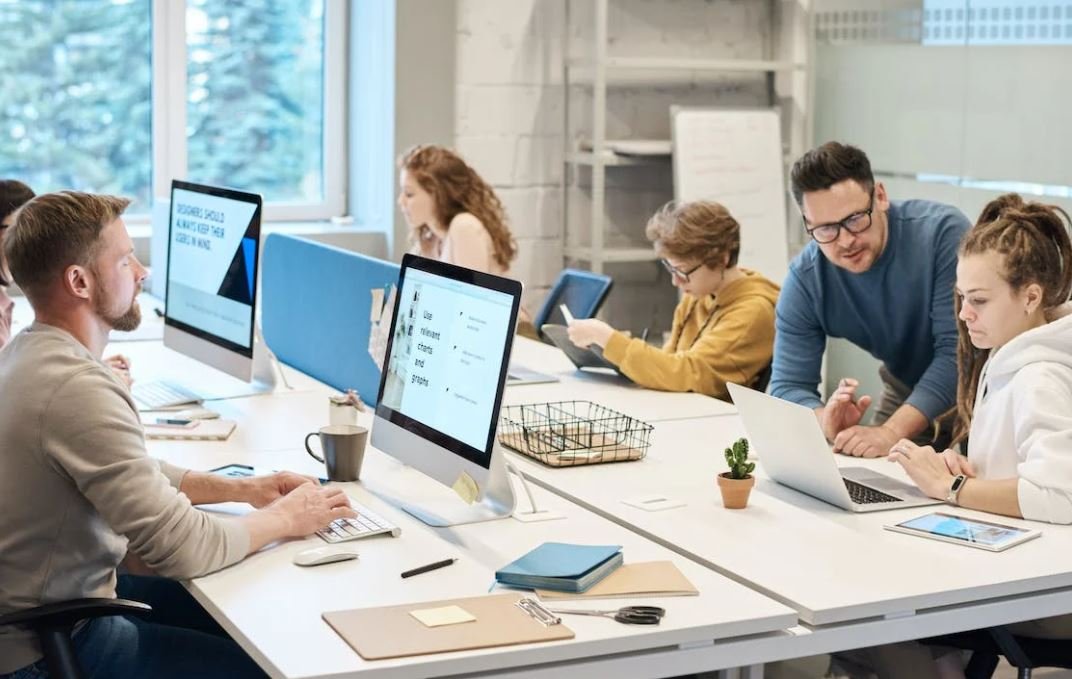
Common Misconceptions
Misconception 1: AI and data science are the same thing
One of the most common misconceptions is that AI (Artificial Intelligence) and data science are interchangeable terms and refer to the same thing. However, this is not true. While both fields are related, they are distinct disciplines with different focuses.
- AI aims to create intelligent machines that can simulate human behavior and perform tasks requiring human-like intelligence.
- Data science, on the other hand, focuses on extracting insights and knowledge from data through various techniques and algorithms.
- AI relies on data science to collect and analyze data for making intelligent decisions.
Misconception 2: AI will replace humans in all jobs
Another common misconception is that AI will ultimately replace humans in all jobs, leading to widespread unemployment. While it’s true that AI has the potential to automate certain tasks and jobs, it is unlikely to completely replace human workers in most industries.
- Many jobs require complex decision-making, creativity, and emotional intelligence, which AI currently lacks.
- AI is a tool that can enhance human capabilities and productivity, rather than replacing humans entirely.
- Instead of replacing jobs, AI is more likely to transform job roles and create new opportunities in areas such as AI development and data science.
Misconception 3: AI is infallible and free from biases
AI systems are often seen as infallible and unbiased decision-makers, but this is not the case. AI models are trained using data, and if the training data contains biases or errors, the AI system can inherit those biases as well.
- AI systems can inadvertently perpetuate existing biases and discrimination present in the data it is trained on.
- It is important to ensure that AI systems are trained on diverse and representative datasets to minimize biases.
- Regular monitoring and auditing of AI systems are necessary to identify and address biases that may arise over time.
Misconception 4: AI is only applicable in high-tech industries
Many people believe that AI is only relevant in industries such as technology and robotics. However, AI has the potential to impact a wide range of industries, including healthcare, finance, transportation, and agriculture.
- In healthcare, AI can analyze medical data to improve diagnoses and treatment plans.
- In finance, AI algorithms can detect fraudulent transactions and make more accurate predictions for investment portfolios.
- In transportation, AI can optimize routes and manage traffic flow to reduce congestion.
Misconception 5: AI will become self-aware and take over the world
One of the most pervasive misconceptions is the idea that AI will become self-aware and take control over humanity, as depicted in science fiction movies. However, this fear of a future AI “takeover” is largely unfounded.
- AI is designed to perform specific tasks and lacks consciousness or self-awareness.
- Intelligent machines are programmed and controlled by humans, and their actions depend on their programming and data inputs.
- Ensuring ethical guidelines and responsible AI development can help mitigate any potential risks associated with AI.
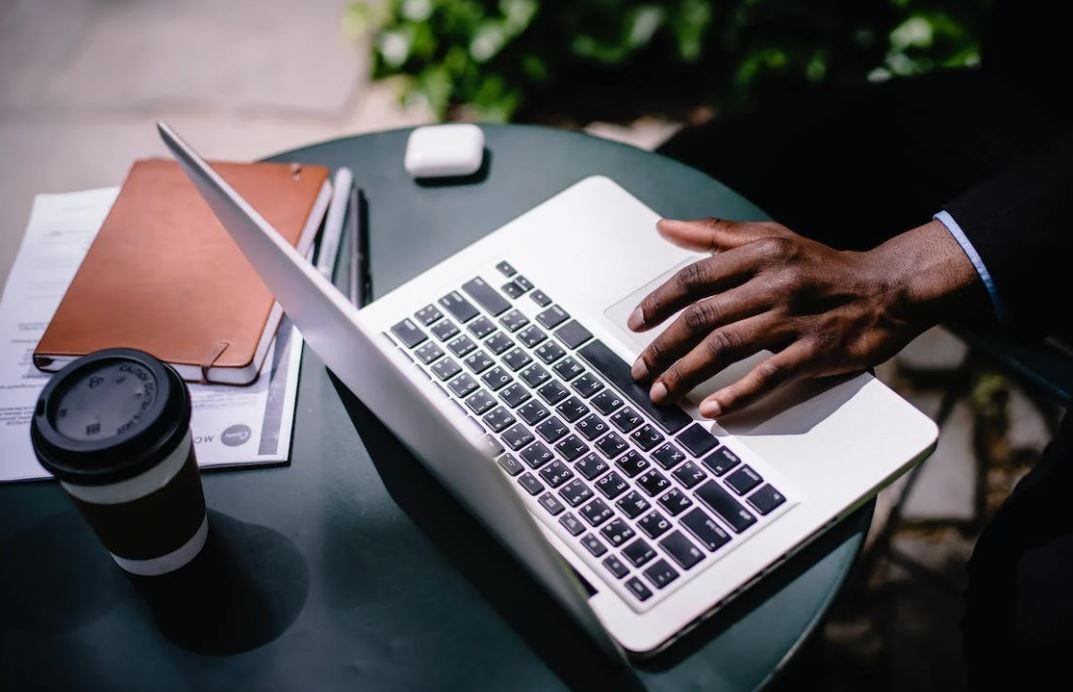
AI: The Future of Technology
Artificial Intelligence (AI) and Data Science are two rapidly growing fields in the world of technology. Both are driving innovation and reshaping industries across the globe. In this article, we explore various aspects of AI and Data Science, comparing their key features, applications, and impacts. Let’s dive into the fascinating world of AI versus Data Science!
AI Applications
The table below showcases some remarkable real-life applications of Artificial Intelligence:
|[Application](#)|[Description](#)|[Impact](#)|[Example](#)|
|—|—|—|—|
|Image Recognition|AI algorithms can accurately identify and label objects, enabling applications like self-driving cars and facial recognition.|Reduces human error and enhances security.|Facebook’s automatic photo tagging.|
|Chatbots|Virtual assistants powered by AI that can interact and answer questions, providing customer support or personalized recommendations.|Improves efficiency and customer satisfaction.|Apple’s Siri or Amazon’s Alexa.|
|Predictive Analytics|AI algorithms analyze historical data to make predictions and forecasts, aiding businesses in making informed decisions.|Boosts productivity and profitability.|Netflix’s recommendation system.|
|Medical Diagnosis|AI models can analyze medical data to assist doctors in diagnosing diseases and recommending treatments.|Improves accuracy and saves time.|IBM’s Watson in cancer diagnosis.|
|Financial Fraud Detection|AI algorithms can detect patterns and anomalies in financial transactions, identifying potential fraud attempts.|Safeguards economies and reduces financial losses.|Mastercard’s fraud detection system.|
Data Science Techniques
Data Science employs various techniques to extract insights and drive decision-making processes. The following table highlights some key techniques:
|[Technique](#)|[Description](#)|[Application](#)|[Example](#)|
|—|—|—|—|
|Machine Learning|Utilizes algorithms to train models and let them learn from data, enabling predictions and classifications.|Personalized recommendations and predictive analysis.|Google’s search algorithm.|
|Data Visualization|Represents data visually through charts, graphs, and maps, aiding in the understanding and communication of complex information.|Presenting trends and patterns for better decision-making.|Tableau’s interactive dashboards.|
|Statistical Analysis|Applies statistical methods to uncover relationships and dependencies within data, allowing for hypothesis testing and inference.|Identifying significant factors and determining statistical significance.|A/B testing in marketing campaigns.|
|Text Mining|Extracts information and insights from unstructured textual data, analyzing trends, and sentiments.|Sentiment analysis and topic modeling.|Google Translate’s language processing.|
|Big Data Management|Handles large and complex datasets, ensuring efficient storage, processing, and retrieval for analytics purposes.|Scalable data storage and real-time analytics.|Apache Hadoop’s distributed file system.|
AI Ethics and Challenges
The ethical implications and challenges associated with AI are crucial aspects to consider. The following table highlights some significant concerns:
|[Ethical Concern](#)|[Description](#)|[Impact](#)|[Example](#)|
|—|—|—|—|
|Job Displacement|Automation of tasks through AI may lead to job losses and economic disparity.|Unemployment rates increase, requiring workforce adaptation.|Automation in the manufacturing industry.|
|Bias and Fairness|AI systems can inherit biases from the data used to train them, resulting in discriminatory outcomes.|Unequal treatment based on race, gender, or other factors.|Racial profiling in facial recognition.|
|Privacy and Security|AI systems handle vast amounts of personal data, raising concerns about privacy breaches and data leaks.|Violation of individuals’ privacy rights and personal information exposure.|Cambridge Analytica’s Facebook data scandal.|
|Lack of Transparency|Complex AI models can be opaque and difficult to interpret, making it challenging to understand decision-making processes.|Reduced accountability and difficulty in identifying errors or biases.|Black box trading algorithms in finance.|
|Autonomous Weapons|The development of AI-powered weaponry raises ethical debates around decision-making and accountability.|Increased risks of indiscriminate, lethal autonomous systems.|DARPA’s development of autonomous drones.|
Data Science Salaries
Data Scientists are in high demand, and their salaries reflect the significance of their skills and expertise. The table below shows the average salaries for Data Scientists in different countries:
|[Country](#)|[Average Salary](#)|
|—|—|
|United States| $120,000 – $150,000 |
|United Kingdom| £50,000 – £90,000 |
|Canada| CAD 75,000 – CAD 100,000 |
|Germany| €65,000 – €100,000 |
|Australia| AUD 100,000 – AUD 150,000 |
AI and Data Science: Job Market
The demand for professionals in AI and Data Science continues to grow rapidly. The following table provides an overview of job postings:
|[Job Market](#)|[Number of Postings](#)|[Top Industries](#)|
|—|—|—|
|United States| 250,000+ | Technology, Finance, Healthcare |
|United Kingdom| 50,000+ | Finance, Retail, Consulting |
|India| 100,000+ | IT Services, E-commerce, Healthcare|
|Germany| 40,000+ | Automotive, Manufacturing, Technology |
|Australia| 30,000+ | Finance, IT, Telecommunications |
AI and Data Science in Academia
Academic institutions play a vital role in research and education in AI and Data Science. The table below lists some notable universities offering relevant programs and research:
|[University](#)|[Location](#)|[Ranking](#)|[Research Areas](#)|
|—|—|—|—|
|Massachusetts Institute of Technology (MIT)|Cambridge, USA|1|Machine Learning, Natural Language Processing|
|Stanford University|Stanford, USA|2|Computer Vision, Reinforcement Learning|
|Harvard University|Cambridge, USA|3|Data Privacy, Computational Genomics|
|University of Oxford|Oxford, UK|4|Data Ethics, Deep Learning|
|ETH Zurich|Zurich, Switzerland|5|Artificial Intelligence, Robotics|
AI and Data Science Funding
Investment in AI and Data Science is crucial for technological advancement. The following table showcases some prominent funding initiatives:
|[Funding Initiative](#)|[Description](#)|[Investment Amount](#)|
|—|—|—|
|National Artificial Intelligence Research Institutes|Funded by the National Science Foundation (NSF) to accelerate AI innovations in various sectors.|$100 million (approx.)|
|European Union Horizon 2020|Funding program supporting research and innovation in Europe, with a focus on AI and Data Science projects.|€80 billion (approx.)|
|AI Development Fund|Established by the Chinese government to fuel AI development and establish the country as a global AI leader.|¥10 billion (approx.)|
|AI for Earth|An initiative by Microsoft, offering grants to organizations that leverage AI for environmental conservation and sustainability.|$50 million (approx.)|
The Future of AI and Data Science
The potential of AI and Data Science is immense, and their integration is likely to shape the future of technology. As AI becomes more advanced and Data Science further revolutionizes businesses and industries, we can expect remarkable advancements in healthcare, automation, optimization, and personalized experiences. Embracing the exciting possibilities of AI and Data Science will undoubtedly lead us towards a technologically empowered future.
AI vs Data Science – Frequently Asked Questions
Q: What is the difference between AI and Data Science?
A: AI (Artificial Intelligence) refers to the development of machines or software that can mimic human intelligence
and perform tasks that would typically require human intelligence. Data Science, on the other hand, focuses on
extracting insights and knowledge from data using various statistical and machine learning techniques.
Q: How are AI and Data Science related?
A: Data Science plays a crucial role in the development and implementation of AI systems. AI relies heavily on
large amounts of data, and Data Science provides the necessary tools and techniques to analyze, clean, and
interpret the data for training AI models.
Q: What are some typical AI applications?
A: AI is widely used across various industries and applications. Some common AI applications include natural
language processing, image recognition, autonomous vehicles, recommendation systems, and fraud detection.
Q: Can AI be considered a part of Data Science?
A: Yes, AI can be considered a subset of Data Science. Data Science covers a broad range of techniques and
methodologies for data analysis, while AI specifically focuses on the development of intelligent systems that
can learn and make intelligent decisions.
Q: How does Data Science support AI development?
A: Data Science enables AI development by providing the necessary tools and techniques for data collection,
preprocessing, feature engineering, model training, and evaluation. It helps in extracting meaningful insights
from data and optimizing AI models.
Q: Are AI and Data Science career paths similar?
A: While there may be overlap, AI and Data Science career paths are distinct. AI professionals generally focus on
developing and implementing AI systems, while Data Scientists focus on analyzing and extracting insights from
data.
Q: What skills are required for a career in AI?
A: A career in AI often requires a strong background in mathematics, programming, statistics, and machine
learning. Proficiency in programming languages like Python and knowledge of AI frameworks is also beneficial.
Q: Are AI and Data Science used together in practice?
A: Yes, AI and Data Science are often used together in practice. Data Science provides the foundation for AI
models by analyzing and preparing the data, while AI enhances Data Science by enabling intelligent decision
making and automation based on the insights derived from data.
Q: What are the future prospects of AI and Data Science?
A: The future prospects for both AI and Data Science are promising. As data continues to grow exponentially, the
demand for skilled professionals in AI and Data Science is expected to increase, with new applications and
advancements to be made in these fields.
Q: How are AI and Data Science transforming industries?
A: AI and Data Science have the potential to revolutionize industries by automating processes, improving
decision-making, enhancing efficiency, and enabling the development of innovative products and services. They
are driving advancements in sectors such as healthcare, finance, manufacturing, and transportation.