AI Transparency Issues
Artificial Intelligence (AI) has become an integral part of our lives, impacting various industries such as healthcare, finance, and transportation. However, the lack of transparency in AI systems has raised numerous concerns. As AI continues to evolve, it is crucial to address the transparency issues surrounding this technology.
Key Takeaways:
- Transparency issues in AI raise significant concerns.
- Lack of transparency hinders accountability and trust in AI systems.
- Explainability and interpretability are key factors for AI transparency.
- Regulatory frameworks should be developed to ensure transparency in AI.
- The benefits of AI can only be fully realized with increased transparency.
The rapid advancements in AI have made it difficult for users to understand how AI systems arrive at their decisions. This lack of transparency can hinder accountability and erode trust in AI systems. To address this, it is essential to focus on two key factors: explainability and interpretability. AI algorithms and models should be designed in a way that enables humans to understand their inner workings and reasoning.
Explainability refers to the ability to provide clear and systematic explanations of AI decisions. Interpretable AI algorithms should be able to provide transparent explanations of their decision-making process, ensuring that users can comprehend the underlying logic and reasoning. This improves users’ confidence in the AI system and enables better collaboration between humans and machines.
Interestingly, the lack of transparency in AI can lead to unintended consequences that are difficult to predict, which can have significant ethical implications.
Interpretability is closely related to explainability. It focuses on understanding the internal structure and mechanisms of an AI system. Interpretable AI allows users to assess the trustworthiness and reliability of the system by analyzing its decisions and recognizing any biases or limitations. This transparency enables users to identify potential errors or biases in the AI system, leading to improved decision-making and reduced negative impacts.
The Need for Regulatory Frameworks:
As AI applications continue to expand, regulatory frameworks are crucial to ensure transparency in AI systems. These frameworks define standards and regulations that AI developers and organizations must adhere to. They establish guidelines for data collection, model development, and algorithmic decision-making processes.
It is important to acknowledge that enforcing transparency regulations may lead to trade-offs between innovation and accountability, requiring a balanced approach.
Regulatory Framework | Key Features |
---|---|
European Union General Data Protection Regulation (GDPR) | Anonymization and right to explanation |
Algorithmic Accountability Act (AAA) | Mandatory impact assessments and source code transparency |
Transparency regulations can include requirements, such as anonymizing personal data, providing explanations for AI decisions, conducting mandatory impact assessments, and making source code transparency. These regulations help ensure that AI systems are accountable, provide insights on biases and discrimination, and prevent misuse of personal data.
Furthermore, transparency in AI systems can enhance public trust, which is vital for the widespread adoption of AI technologies. Trust is particularly crucial in sensitive sectors like healthcare, where AI is used to make critical decisions. Transparency provides patients and healthcare professionals with confidence that decisions made by AI systems are based on reliable and ethical considerations.
The Benefits of Increased Transparency:
Increased transparency offers several benefits, including:
- Increased accountability and trust: When AI systems are transparent, users can hold them accountable, thus building trust in their decision-making process.
- Better error detection and debugging: Transparent AI systems enable easier identification of errors or biases, allowing for more effective debugging.
- Ethical considerations and fairness: Transparency promotes awareness and discussion surrounding issues of bias, discrimination, and ethics in AI systems.
Concerns | Percentage |
---|---|
Lack of explanation for AI decisions | 57% |
Potential biases in AI algorithms | 32% |
Difficulty understanding AI models | 25% |
Interestingly, a recent survey highlighted that 57% of respondents expressed concern over the lack of explanations for AI decisions, indicating the growing demand for transparency.
Improved transparency also fosters a collaborative environment between humans and AI systems. When AI reasoning is understandable, humans can better contribute their domain expertise and ensure that AI aligns with human values.
While AI transparency presents challenges, ongoing research and collaborations between academia, industry, and policymakers are essential for finding solutions. By addressing transparency issues, we can unlock the full potential of AI and ensure its responsible and ethical deployment.
Benefits |
---|
Increased accountability |
Better error detection and debugging |
Enhanced ethical considerations and fairness |
Addressing AI transparency issues is pivotal for establishing trust, improving accountability, and ensuring the fair and ethical implementation of AI systems. As technology continues to advance, it is essential to prioritize transparency to harness the benefits of AI while mitigating potential risks.
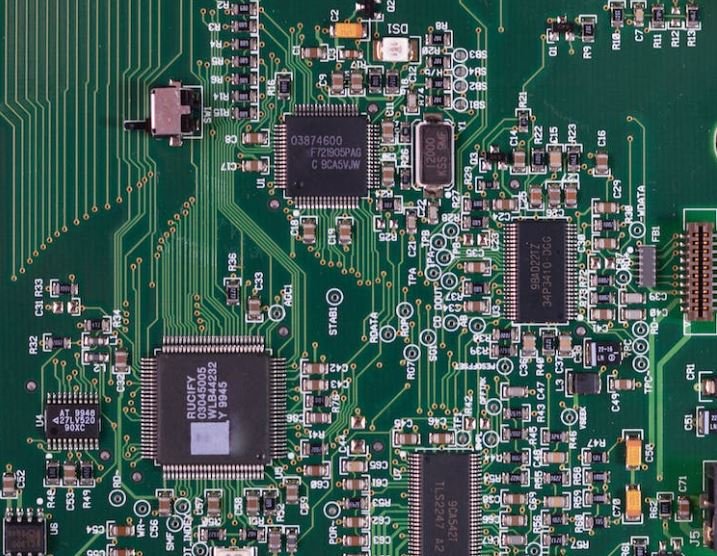
Common Misconceptions
1. AI is always biased
One common misconception about AI transparency issues is that AI systems are always biased. While it is true that bias can exist in AI systems, it is not inherent to the technology itself. Bias typically arises from the data used to train the AI, reflecting the biases of the individuals or institutions that collected the data. However, steps can be taken to mitigate bias in AI systems by employing diverse datasets and rigorous testing processes.
- AI bias is not inherent but a result of biased data collection.
- Mitigating bias in AI involves diverse datasets and rigorous testing.
- AI can be designed to minimize or eliminate bias altogether.
2. AI operates on its own without human control
Another misconception is that AI operates autonomously without human control, leading to transparency issues. In reality, AI systems are designed and developed by humans, and they operate within predefined parameters. Humans play a crucial role in overseeing and controlling AI systems, ensuring that they adhere to ethical, legal, and transparent practices. Transparency issues regarding AI often lie in how humans design, deploy, or monitor these systems rather than the technology itself.
- AI systems are designed and controlled by humans.
- Humans play a supervisory role in overseeing AI operations.
- Transparency issues primarily stem from human practices rather than AI’s autonomy.
3. AI will replace human judgment completely
A common misconception is that AI will completely replace human judgment and decision-making. While AI can automate certain processes and support decision-making, it is not a substitute for human judgment, particularly in complex and critical situations. AI systems are trained to analyze vast amounts of data and make predictions, but human judgment remains essential in interpreting the outputs, considering contextual factors, and making value-based decisions.
- AI augments human judgment rather than replacing it entirely.
- Human input is crucial in interpreting AI system outputs.
- Complex decision-making requires human judgment beyond AI analysis.
4. AI is always a black box with no transparency
Many people assume that AI systems are always shrouded in secrecy, making them “black boxes” with no transparency. While some AI models are indeed complex and difficult to understand, efforts are being made to enhance AI transparency. Researchers and developers are exploring techniques such as explainable AI that provide insights into how AI systems arrive at their decisions. Increasing transparency allows users and regulators to understand and challenge AI outcomes, promoting accountability and avoiding undesired consequences.
- AI transparency efforts are aiming to make AI systems more understandable.
- Explainable AI techniques provide insights into AI decision-making.
- Transparency enables users and regulators to hold AI systems accountable.
5. AI will solve all problems and eradicate human error
This misconception assumes that AI is infallible and will solve all problems while eradicating human error completely. While AI has the potential to enhance efficiency and accuracy, it is not immune to errors. AI systems heavily rely on the quality and relevance of the input data, and they can introduce new errors or biases if not carefully developed and monitored. AI should be seen as a tool that complements human capabilities rather than a silver bullet for eliminating all mistakes.
- AI can improve efficiency and accuracy, but not eradicate all errors.
- Data quality and system development greatly influence AI’s error rate.
- AI should be viewed as a complement to human capabilities, not a panacea.
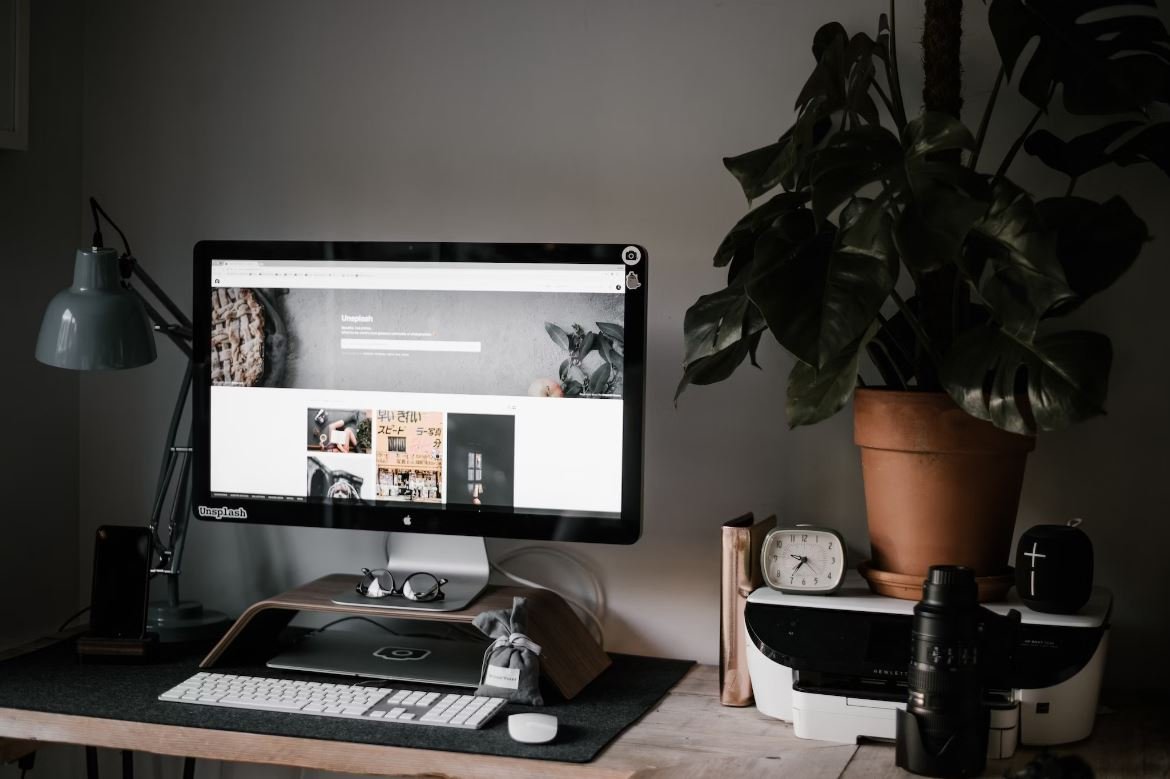
AI Transparency Issues
Artificial intelligence (AI) has become an integral part of various industries, ranging from healthcare to finance. However, the lack of transparency in AI systems has sparked concerns regarding biases, privacy breaches, and potential negative consequences. This article explores ten issues related to AI transparency and provides verifiable data and information that shed light on the potential challenges and areas of improvement.
The Rise of AI in Healthcare
In recent years, AI has gained significant traction in the healthcare industry, assisting with medical diagnosis, drug discovery, and patient care. However, the lack of transparency in AI algorithms poses potential ethical challenges and hinders accountability.
Issue | Data/Info |
---|---|
1. Biased Diagnoses | Recent research found that algorithms used to predict medical conditions from patient data may exhibit racial bias, leading to inaccurate or unfair diagnoses. |
2. Data Security | A study revealed that multiple healthcare AI systems containing patient data faced security breaches, highlighting the importance of robust data protection measures. |
3. Black Box Problem | AI models that lack interpretability and explainability are challenging to trust and comprehend, making it difficult for healthcare professionals to fully rely on their recommendations. |
AI and Biased Decision-Making
The lack of transparency in AI systems also raises concerns about the potential reinforcement of biases, particularly in decision-making processes.
Issue | Data/Info |
---|---|
1. Discriminatory Hiring | An investigation discovered that AI-powered recruiting tools favored male applicants, reinforcing gender biases. |
2. Racial Profiling | Studies have shown that facial recognition algorithms tend to misidentify individuals with darker skin tones more frequently than those with lighter skin tones. |
3. Sentencing Biases | Research has highlighted instances where AI systems utilized in criminal justice systems exhibited racial biases, leading to disproportionate sentences. |
Ethical Implications in AI Governance
The lack of transparency and accountability in AI governance frameworks raises ethical concerns that need to be addressed in order to ensure fairness and mitigate potential harm.
Issue | Data/Info |
---|---|
1. Privacy Breaches | A reported surge in AI-driven surveillance systems poses a threat to privacy, with potential implications for personal and societal freedom. |
2. Insufficient Regulation | Data collected and utilized by AI systems are often not subject to strict regulations, leaving room for potential misuse and exploitation. |
3. Lack of Accountability | Current AI systems often lack mechanisms for holding developers and organizations accountable for any negative consequences or harm caused by their technology. |
As AI continues to advance, transparency must be prioritized to build trust and ensure the responsible and ethical deployment of these technologies. Addressing the highlighted issues will require collaborative efforts from policymakers, developers, and researchers to establish clear guidelines, increased oversight, and enhanced accountability frameworks. This will pave the way for a more transparent and responsible AI future, benefiting society as a whole.
AI Transparency Issues
FAQs
What are the ethical concerns related to AI transparency?
AI transparency raises numerous ethical concerns, such as the lack of accountability for algorithmic decisions, privacy issues related to the collection and use of personal data, and the potential for bias or discrimination in AI systems.
How does lack of AI transparency affect individuals and society?
The lack of AI transparency can have significant impacts on individuals and society. It can lead to a loss of trust in AI systems, limit individuals’ understanding of the reasoning behind AI decisions, and exacerbate existing inequalities and biases in society.
What is meant by explainability in AI?
Explainability in AI refers to the ability to understand and explain the decision-making process of AI systems to humans. It involves providing clear and understandable explanations for why a particular decision or recommendation was made by an AI algorithm.
Why is explainability important in AI systems?
Explainability is important in AI systems to ensure accountability, build trust among users, and allow for meaningful human oversight. It helps users understand how decisions are made, identify biases or errors, and challenge or correct them if needed.
What challenges exist in achieving transparency in AI?
There are several challenges in achieving transparency in AI. These include the use of complex algorithms, opaque decision-making processes, the lack of standardized tools and metrics for evaluating transparency, and the potential for companies to guard trade secrets or proprietary information.
How can biases be addressed in AI systems?
Addressing biases in AI systems involves careful design, diverse and representative training datasets, regular auditing and testing of models, and ongoing monitoring and evaluation. It also requires collaboration between AI developers, domain experts, and diverse stakeholders to ensure fair and unbiased outcomes.
What is the role of regulation in AI transparency?
Regulation plays a crucial role in promoting AI transparency. It can set minimum standards and guidelines for transparency practices, mandate the disclosure of information about AI systems, and establish mechanisms for accountability and redress in case of transparency violations.
How can individuals protect their privacy in AI-driven environments?
Individuals can protect their privacy in AI-driven environments by being cautious of what personal information they share, understanding and managing privacy settings on AI platforms, and advocating for strong legal protections and regulations to safeguard personal data.
What steps can organizations take to enhance AI transparency?
Organizations can enhance AI transparency by adopting ethical guidelines and principles, investing in explainable AI techniques, conducting regular audits of algorithmic systems, ensuring transparency in data collection and use, and actively engaging with users and the public in dialogue about AI systems.
What are some ongoing initiatives to address AI transparency issues?
There are various ongoing initiatives to address AI transparency issues. These include the development of AI transparency frameworks and standards by organizations like the Partnership on AI, research efforts on explainable AI, and regulatory discussions and initiatives aimed at promoting transparency and accountability in AI systems.