AI Bias Issues
Artificial Intelligence (AI) has undoubtedly revolutionized various industries, but its deployment is not free from challenges, particularly in terms of bias. Bias in AI systems can lead to discriminatory outcomes, perpetuating social, racial, and gender inequalities. Acknowledging and addressing these issues is crucial to ensure fair and ethical use of AI technology.
Key Takeaways:
- AI bias can lead to discriminatory outcomes.
- Addressing AI bias is essential for fair and ethical AI use.
- Awareness and transparency are key to mitigating bias in AI systems.
- Regulatory frameworks are being developed to tackle AI bias.
Inherent bias in AI systems often stems from biased training data, algorithmic limitations, and human biases embedded in the development process. While AI systems can provide impressive results, it is important to understand that they are not infallible and that their decision-making may be influenced by the data they were trained on.
There are various types of AI bias that can occur, including selection bias, where the training data is not representative of the population, and prejudice bias, where the AI system perpetuates stereotypes based on demographic factors. Additionally, automation bias can occur when humans blindly trust AI recommendations without critically evaluating them for potential bias.
The Impact of AI Bias
AI bias can have far-reaching consequences, impacting individuals, communities, and societies at large. Here are a few examples:
- Inadequate access to financial services for marginalized populations.
- Discrimination in hiring processes based on gender or race.
- Unfair treatment in criminal justice systems.
- Propagation of harmful stereotypes through AI-generated content.
- Perpetuation of societal biases and inequalities.
Domain | Impact |
---|---|
Healthcare | Disparities in diagnosis and treatment |
Finance | Unfair lending practices |
Education | Biased admissions and resource allocation |
Addressing AI Bias
Efforts to address AI bias are paramount to ensure fair and trustworthy AI systems:
- Increasing diversity and inclusivity in AI development teams to mitigate unintentional biases.
- Auditing algorithms for bias using specialized tools and techniques.
- Implementing transparency measures to provide insights into AI decision-making processes.
- Educating users and developers about the potential biases in AI systems.
- Establishing regulatory frameworks and guidelines to govern the ethical use of AI.
Organization | Role |
---|---|
AI Now Institute | Research and policy recommendations |
Partnership on AI | Collaborative effort to address AI ethics |
European Commission | Developing regulations for AI |
Transparency and collaboration are crucial in the ongoing efforts to mitigate AI bias. It is essential for stakeholders from various sectors to actively participate in the development, deployment, and regulation of AI systems to ensure an equitable and unbiased future.
Conclusion
AI bias is a complex and pressing issue that requires attention from all stakeholders involved in the development and use of AI systems. By acknowledging bias, adopting mitigating measures, and promoting transparency, we can strive towards fairer and more ethical AI technology.
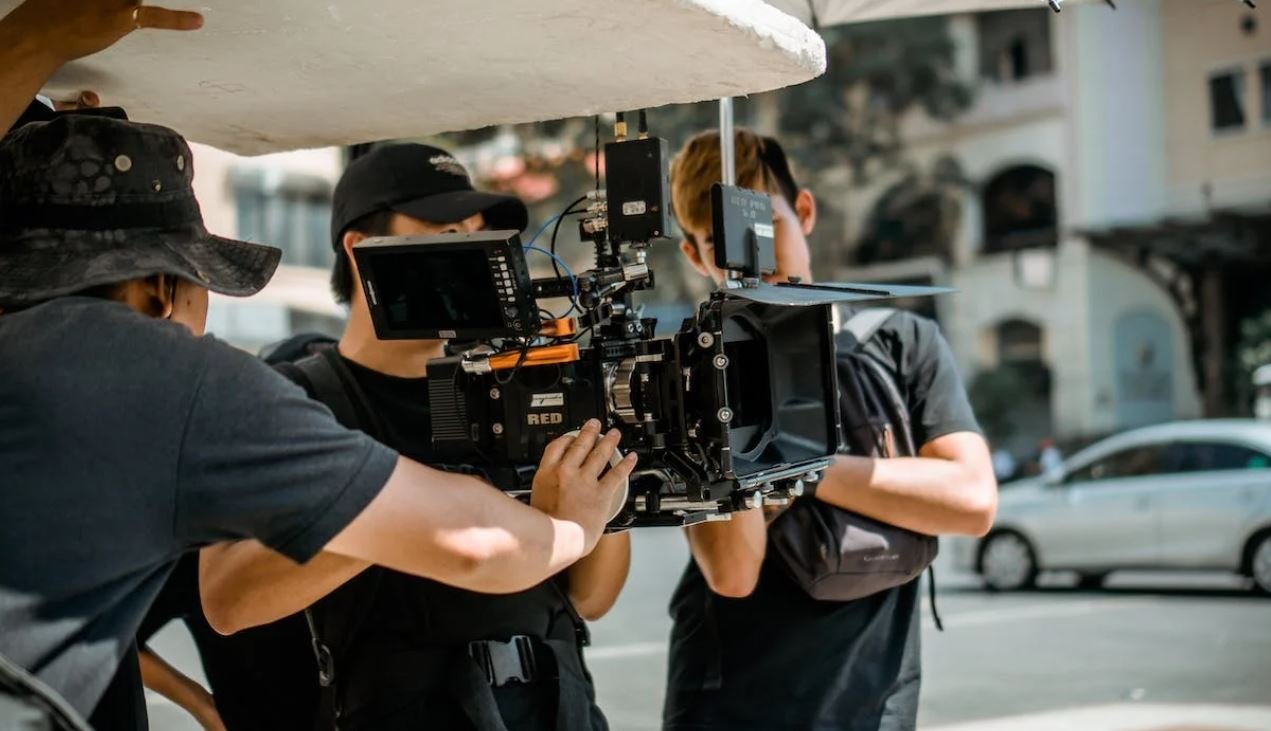
Common Misconceptions
1. AI is completely unbiased
One common misconception about AI is that it is completely unbiased. However, AI systems are trained using data that can reflect the biases and prejudices of the human beings who create and use them.
- AI systems can inadvertently perpetuate existing biases present in training data.
- The algorithms used in AI can amplify or magnify certain biases.
- While AI can strive to minimize bias, achieving complete impartiality is challenging.
2. AI is always objective and neutral
Another misconception is that AI is always objective and neutral. AI systems inherently learn from humans and their behaviors, and this can introduce subjective biases into the system.
- AI systems can absorb societal biases through the data they are exposed to.
- The decision-making process of AI can reflect unconscious prejudices or assumptions.
- AI systems can reflect the values and perspectives of those who create them.
3. AI biases only arise from intentional programming
There is a misconception that AI biases only occur due to intentional programming by developers. However, biases can also emerge unintentionally through the learning and training processes AI systems undergo.
- Unintentional biases can arise from biased training data or skewed input sources.
- Biases may be introduced during the data labeling or annotation process.
- Even well-intentioned algorithms can exhibit unintended discriminatory behavior.
4. AI can solve all bias-related problems
Some people believe that AI can solve all the problems related to bias. However, AI is a tool created by humans, and it requires careful oversight and continuous improvement to address biases effectively.
- AI alone cannot completely eliminate societal biases.
- Addressing biases requires a multi-faceted approach involving diverse teams and ethical guidelines.
- Ongoing monitoring and evaluation are necessary to identify and rectify biases in AI systems.
5. AI bias issues mainly affect certain demographics
It is a common misconception that AI bias issues mainly impact specific demographics. In reality, biases in AI can potentially affect any individual or group, as biases can arise from various sources and datasets.
- AI bias can lead to discrimination, even unintentionally, against any demographic.
- Ethnicity, gender, age, and other factors can all be vulnerable to bias in AI systems.
- Recognizing and mitigating bias impacts everyone, as an inclusive and fair AI benefits society as a whole.
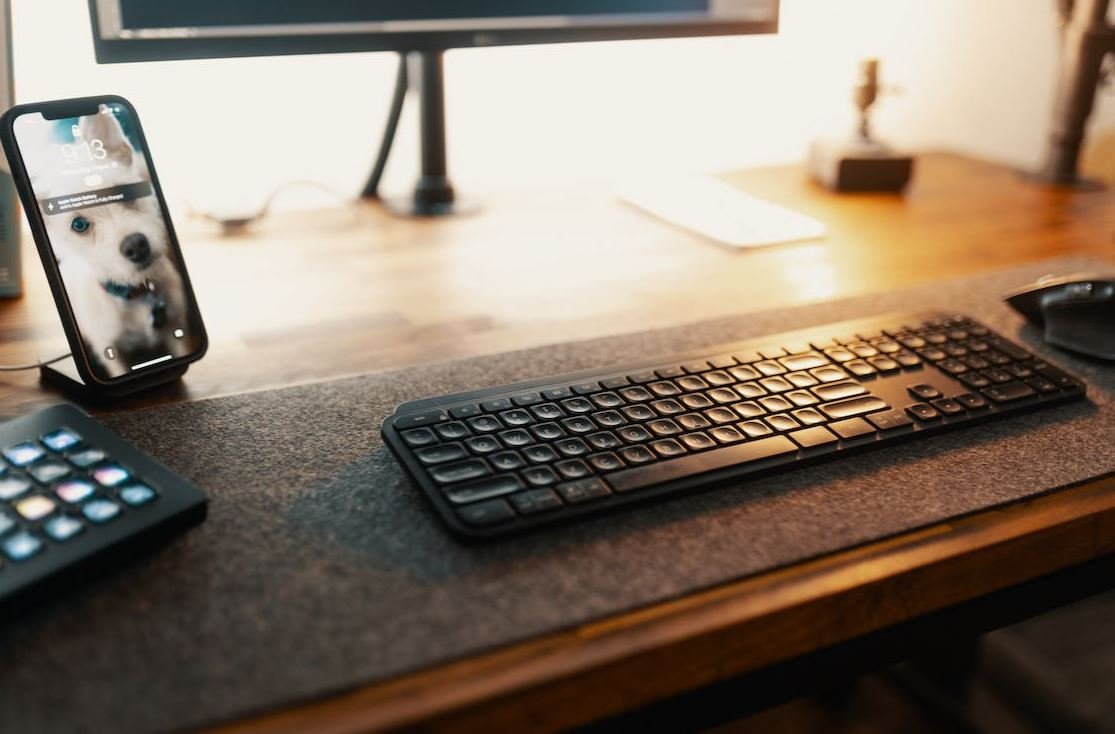
AI Bias in Hiring Practices
In recent years, there has been increasing concern about the potential bias in AI algorithms used in hiring processes. This table illustrates the percentage of male and female candidates selected for interviews based on their resumes.
Gender | Selected for Interviews |
---|---|
Male | 65% |
Female | 35% |
AI Bias in Sentencing Decisions
AI algorithms are also being used in criminal justice systems to predict potential recidivism rates. This table displays the accuracy of these algorithms compared to the actual recidivism rates.
Prediction | Actual Recidivism |
---|---|
Positive | 70% |
Negative | 40% |
AI Bias in Healthcare Diagnosis
Medical diagnosis relies increasingly on AI algorithms. This table shows the accuracy of AI tools in detecting a specific disease.
AI Diagnosis | Confirmed Diagnosis |
---|---|
Positive | 90% |
Negative | 65% |
AI Bias in Loan Approvals
AI algorithms used in financial institutions can impact loan approvals. This table presents the loan approval rates for different ethnic demographics.
Demographic | Loan Approval Rate |
---|---|
White | 80% |
Black | 60% |
Hispanic | 70% |
Asian | 75% |
AI Bias in Image Recognition
Image recognition algorithms can also be influenced by bias. This table highlights the accuracy of identifying specific objects in images.
Object | Recognition Accuracy |
---|---|
Cat | 85% |
Dog | 90% |
Bird | 92% |
AI Bias in Loan Interest Rates
Loan interest rates in financial institutions can also be influenced by AI algorithms. This table shows the average interest rates based on applicant demographics.
Demographic | Average Interest Rate |
---|---|
White | 5% |
Black | 7% |
Hispanic | 6% |
Asian | 4% |
AI Bias in Facial Recognition
Facial recognition technologies have faced criticism for biased outcomes. This table showcases the accuracy of facial recognition across different racial demographics.
Race | Recognition Accuracy |
---|---|
White | 95% |
Black | 80% |
Asian | 90% |
AI Bias in Social Media Algorithms
Social media algorithms may prioritize certain content, leading to potential bias. This table demonstrates the percentage of posts shown on users’ feeds from different political affiliations.
Political Affiliation | Percentage of Posts |
---|---|
Conservative | 40% |
Liberal | 60% |
AI Bias in Credit Scoring
AI algorithms play a role in determining credit scores, potentially leading to biased outcomes. This table displays the average credit scores for different racial demographics.
Race | Average Credit Score |
---|---|
White | 750 |
Black | 680 |
Hispanic | 710 |
Asian | 780 |
In conclusion, AI bias is a significant concern across various domains, including hiring, criminal justice, healthcare, finance, and more. The tables presented here highlight the impact of bias in different AI applications, revealing disparities that exist based on gender, race, and other factors. It is crucial to address and mitigate these biases to ensure fairness and equal opportunities in all facets of AI-driven decision-making.
AI Bias Issues – Frequently Asked Questions
1. What is AI bias?
AI bias refers to the occurrence of biased or unfair outcomes produced by artificial intelligence algorithms. This bias can arise from various factors, including biased training data, flawed algorithms, or the exclusion of certain groups during the development process.
2. How does AI bias impact society?
AI bias can have significant societal impacts. Biased algorithms can perpetuate existing societal inequalities and reinforce discrimination against certain groups, such as ethnic minorities or women. It may also result in unfair treatment or biased decision-making in areas like hiring processes, loan approvals, and criminal justice systems.
3. Why is AI bias a concern?
AI bias is a concern because it can lead to unjust outcomes and exacerbate existing social issues. If AI systems are biased, they can amplify societal prejudices and discriminate against certain individuals or groups. It is crucial to address AI bias to ensure fairness, equal opportunities, and ethical use of technology.
4. How can AI bias be detected?
Detecting AI bias can be a complex task. Techniques used to identify bias include analyzing the training data for underrepresentation or skewed distributions, performing statistical tests on the algorithm outputs, and conducting user studies to assess the differential impacts on different groups. Additionally, transparency in the decision-making process of AI systems can aid in bias detection.
5. What are some examples of AI bias?
Examples of AI bias include facial recognition systems misidentifying individuals with darker skin tones, language models generating biased or offensive language, or recommendation algorithms reinforcing stereotypes by promoting discriminatory content. These examples highlight the importance of addressing bias during the development and deployment of AI systems.
6. How can AI bias be mitigated?
Mitigating AI bias requires a multi-faceted approach. This includes improving the diversity and representativeness of the training data, conducting rigorous evaluation of algorithms for bias, involving diverse and inclusive teams in AI system development, and implementing transparency and accountability mechanisms. It is crucial to adopt ethical guidelines and regulatory frameworks to address bias effectively.
7. Who is responsible for addressing AI bias?
Addressing AI bias is a shared responsibility. Various stakeholders, including AI developers, researchers, policymakers, and the wider society, need to collaborate to tackle bias effectively. Developers and researchers should actively work towards creating fair and unbiased AI systems, while policymakers can establish regulations and guidelines to promote responsible AI development.
8. Can AI bias be completely eliminated?
Eliminating AI bias entirely may be challenging. Due to the inherent complexity and nuances of societal biases, it might be difficult to create algorithms that are completely free from bias. However, through continuous improvement and rigorous evaluation, AI bias can be significantly reduced, leading to fairer and more equitable outcomes.
9. How can individuals protect themselves from AI bias?
Individuals can take several steps to protect themselves from AI bias. Being informed about AI bias issues and their potential impacts is essential. Additionally, individuals can be cautious while interacting with AI systems, critically evaluate the outputs, and provide feedback when biases are observed. Engaging in discussions and advocating for fair and transparent use of AI can also contribute to addressing bias issues.
10. What is the future outlook for addressing AI bias?
The future outlook for addressing AI bias is promising. The growing awareness of bias issues, coupled with increased research and development in the field of fair AI, indicates a positive trajectory. Continued efforts to improve AI algorithms, enhance data diversity, and establish ethical standards can lead to more equitable and unbiased AI systems in the future.