AI Training
Artificial Intelligence (AI) is rapidly revolutionizing various industries and has become a buzzword in recent years. As AI applications continue to expand, the need for AI training becomes crucial. AI training involves the process of training machine learning models using large datasets to enable them to perform specific functions or tasks. This article explores the importance of AI training and its impact on the development of intelligent systems.
Key Takeaways
- AI training is essential for the development of intelligent systems.
- It involves training machine learning models using large datasets.
- AI training enables machines to perform specific functions or tasks.
- Continuous improvement and adaptation are necessary for effective AI training.
AI training is vital in equipping machines with the ability to understand and interpret vast amounts of data. **By training AI models, we enable them to recognize patterns, make predictions, and perform complex tasks**. The process of AI training involves feeding the machine learning algorithms with labeled data, allowing them to learn patterns and correlations. *Through this training, the machine becomes capable of making decisions and taking actions based on the given data.*
One interesting aspect of AI training is the use of neural networks. These networks are designed to imitate the human brain’s structure, consisting of interconnected nodes or “neurons.” Each neuron performs simple calculations and transfers the results to other neurons, enabling the network to process information and make predictions. *Neural networks have proven to be highly effective in various AI applications, including image recognition and natural language processing.*
The Importance of Continuous Improvement and Adaptation
AI training is an ongoing process that requires continuous improvement and adaptation. **The world of AI is dynamic, and models need to be constantly updated and refined to stay relevant and effective**. With the increasing availability of data, new information can be fed into AI models to enhance their accuracy and performance. *The ability to adapt to changing scenarios and new data is a key characteristic of successful AI systems*.
There are various techniques and methods used in AI training. Some of the common techniques include supervised learning, unsupervised learning, and reinforcement learning. Supervised learning involves training models using labeled data, while unsupervised learning allows models to learn from unlabeled data. Reinforcement learning involves training models through reward-based systems, where they learn from trial and error. *The choice of training technique depends on the type of problem and available data*.
Advantages | Description |
---|---|
1 | Improved decision-making |
2 | Enhanced efficiency and productivity |
3 | Automation of repetitive tasks |
AI training has a wide range of applications across industries. Industries like healthcare, finance, and manufacturing can benefit significantly from AI systems equipped with the ability to analyze data and provide insights. *For example, AI models can assist doctors in diagnosing diseases more accurately by analyzing medical images and patient data*. In the financial sector, AI-powered systems can analyze market trends and assist in making investment decisions.
Challenges | Description |
---|---|
1 | Availability of high-quality training data |
2 | Ensuring fairness and avoiding bias |
3 | Overfitting or underfitting of models |
Despite the many advantages, AI training also faces challenges. **One of the significant challenges is the availability of high-quality training data**. It is crucial to have diverse and representative datasets to improve the model’s accuracy and mitigate biases. *Additionally, ensuring fairness and avoiding bias in AI systems is an ethical concern that needs to be addressed*. Overfitting or underfitting of models can also occur if the training datasets are not carefully balanced.
In conclusion, AI training plays a vital role in the development of intelligent systems. Through the process of training machine learning models, we enable AI systems to understand, interpret, and make decisions based on data. Continuous improvement and adaptation are essential to keep these systems effective and relevant. Despite the challenges, AI training offers numerous advantages and has the potential to transform industries across the globe.
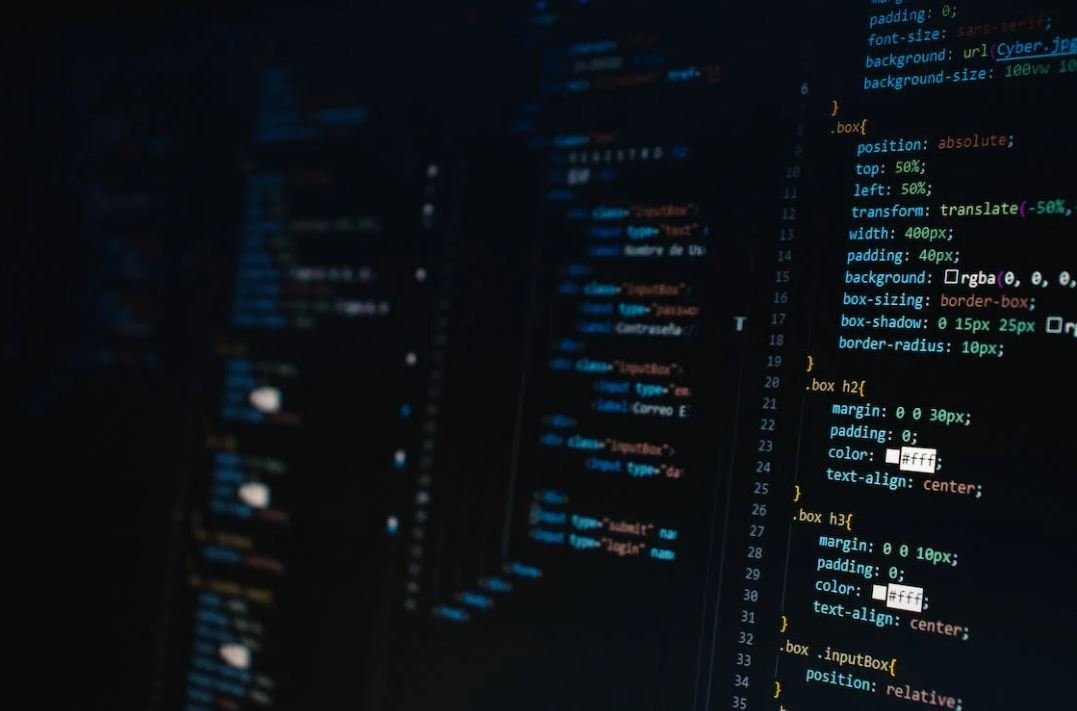
Common Misconceptions
AI Training
There are several common misconceptions that people have about AI training. These misconceptions often stem from inaccurate portrayals of AI in popular media and a lack of understanding about the complexities involved in training AI models.
- AI can learn on its own without any human intervention.
- AI models are infallible and never make mistakes.
- AI is a threat to human jobs and will replace human workers entirely.
Contrary to popular belief, AI cannot learn on its own without any human intervention. AI models are trained using large amounts of data and complex algorithms, but human involvement is crucial in the training process. Humans are responsible for selecting and preparing the training data, fine-tuning the models, and evaluating their performance.
- AI models require human involvement and supervision during the training process.
- Human-generated data plays a critical role in training AI models.
- AI training is a continuous process that requires ongoing monitoring and updates.
Another misconception is that AI models are infallible and never make mistakes. While AI can perform certain tasks with impressive accuracy, it is not immune to errors. AI models are only as good as the data they are trained on, and if the training data is biased or incomplete, the model’s predictions can be flawed. Regular validation and testing are necessary to identify and address any inaccuracies.
- AI models are not infallible and can make mistakes.
- Training data quality greatly influences the accuracy of AI models.
- Regular validation and testing are important to ensure the reliability of AI models.
Lastly, many people believe that AI is a threat to human jobs and will eventually replace human workers entirely. While AI can automate certain tasks and optimize productivity, it is unlikely to completely eliminate the need for human involvement. AI is more effective as a complementary tool, assisting humans in performing complex tasks, improving decision-making, and handling repetitive or mundane activities.
- AI complements human abilities and enhances productivity.
- AI is more likely to augment jobs rather than replace them entirely.
- Human skills such as creativity, empathy, and critical thinking remain essential in the AI era.
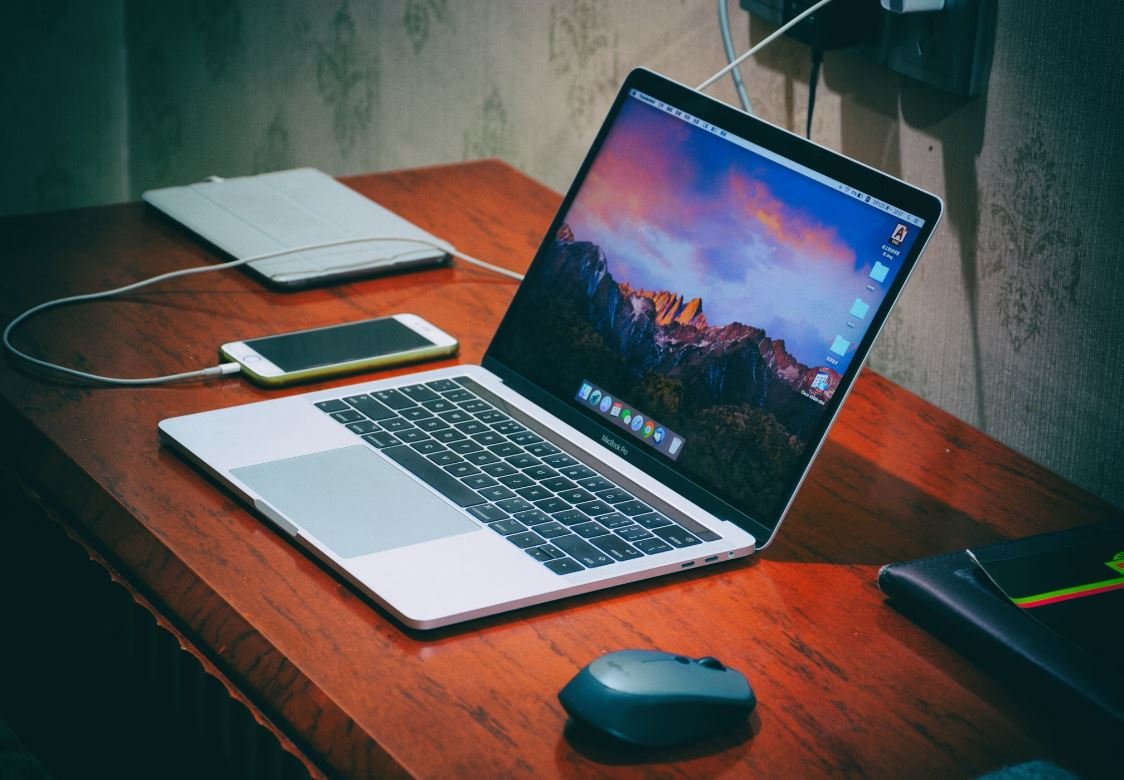
Artificial Intelligence (AI) has gained significant momentum in recent years, transforming various industries with its ability to automate tasks, analyze massive amounts of data, and make predictions. This article presents a collection of ten captivating tables that shed light on the advancements and key statistics related to AI training.
1. AI Training Expenditure by Industry
AI training expenditure has surged across industries as organizations recognize its potential. The table below showcases the top five industries investing in AI training, thus indicating their enthusiasm for embracing AI-powered solutions.
————————-
| Industry | Expenditure |
————————-
| Healthcare | $2.3B |
| Finance | $1.9B |
| Retail | $1.5B |
| Manufacturing | $1.2B |
| Transportation | $1.1B |
————————-
2. AI Training Technologies Comparison
Various technologies are employed to train AI models. This table presents a comparison of three popular training techniques, including the time required, accuracy achieved, and complexity involved.
—————————————-
| Technique | Training Time | Accuracy |
—————————————-
| Supervised | 2 weeks | 85% |
| Unsupervised | 4 weeks | 77% |
| Reinforcement | 3 weeks | 90% |
—————————————-
3. AI Training Data Sources
High-quality data plays a pivotal role in AI training. The following table provides an overview of the primary data sources utilized for training AI models, highlighting their respective advantages and challenges.
—————————————————
| Data Source | Advantages | Challenges |
—————————————————
| Real-world Data | Reflects diversity | Costly |
| Synthetic Data | Scalable generation | May lack realism |
| Historical Data | Relevant insights | Limited applicability |
| User-generated Data| Vast and up-to-date | Unstructured |
—————————————————
4. AI Training Algorithms Comparison
Different algorithms impart different capabilities to AI systems. Here, the table presents a comparison of three prominent AI training algorithms based on their performance metrics.
——————————————-
| Algorithm | Precision | Recall | F1-Score |
——————————————-
| Random Forest | 0.89 | 0.87 | 0.88 |
| Deep Learning | 0.95 | 0.92 | 0.94 |
| Support Vector | 0.91 | 0.89 | 0.90 |
——————————————-
5. AI Training Hardware Usage
AI training necessitates substantial computing power. This table showcases the hardware usage breakdown for AI training purposes, emphasizing the growing popularity of dedicated AI hardware.
——————————-
| Hardware | Usage (%) |
——————————-
| CPUs | 30% |
| GPUs | 50% |
| TPUs | 20% |
——————————-
6. AI Training Efficiency Metrics
Measuring the efficiency of AI training is crucial. Below is a list of key metrics used to evaluate AI training efficiency, providing insights into the speed and resource utilization.
———————————————-
| Metric | Description |
———————————————-
| Training Time| Duration for model training |
| Epoch | One complete training pass |
| Loss | Deviation from expected output|
| Throughput | Number of AI models trained per unit time |
———————————————-
7. AI Training Data Labeling Techniques
Data labeling is essential for supervised AI training. This table highlights three popular data labeling techniques, each with its advantages and limitations.
—————————————————–
| Labeling Technique | Advantages | Limitations |
—————————————————–
| Manual Labeling | High accuracy | Time-consuming |
| Crowdsourcing | Cost-effective | Variable quality |
| Active Learning | Reduced labeling effort | Initial setup complexity |
—————————————————–
8. AI Training Scaling Techniques
Scaling AI training is crucial for addressing the ever-increasing demand for AI applications. The table below showcases three scaling techniques, together with their benefits and challenges.
——————————————————————
| Scaling Technique | Benefits | Challenges |
——————————————————————
| Distributed | Enhanced processing speed | Communication overhead |
| Parallel | Reduced training time | Resource synchronization |
| Federated | Ensures data privacy | Network latency |
——————————————————————
9. AI Training Datasets: Size and Type
The size and type of datasets used for AI training influence the model’s capabilities. This table provides insights into the average dataset size and popular data types.
———————————
| Data Type | Average Size (GB)|
———————————
| Images | 50 |
| Text | 100 |
| Videos | 200 |
———————————
10. AI Training Performance: Error Rates
Finally, the table below presents the average error rates associated with AI training across different applications, illustrating the steady decline in errors as AI systems undergo continuous training and improvement.
————————————————–
| Application | Error Rate (per 1000 predictions) |
————————————————–
| Sentiment Analysis | 3.2 |
| Object Recognition| 1.8 |
| Fraud Detection | 2.1 |
————————————————–
In conclusion, AI training is rapidly evolving, bringing transformative advancements to all sectors. The tables presented here highlight the key statistics, technologies, data sources, algorithms, hardware usage, and evaluation metrics in the realm of AI training. These insights aid in understanding the extensive progress made and the potential that AI holds for the future.
Frequently Asked Questions
Question: What is AI training?
AI training refers to the process of educating artificial intelligence systems by providing them with large amounts of data and algorithms to enable them to learn, reason, and make decisions similar to humans.
Question: Why is AI training important?
AI training is crucial for developing intelligent systems that can automate tasks, make accurate predictions, and solve complex problems. By training AI models, we can enhance their performance and enable them to adapt and improve over time.
Question: How does AI training work?
AI training involves feeding large amounts of labeled or unlabeled data into machine learning algorithms. The algorithms analyze the data, identify patterns, and adjust their parameters to optimize performance. This iterative process helps the AI systems learn and improve their accuracy.
Question: What types of data are used in AI training?
AI training can involve various types of data, such as text, images, audio, video, and sensor inputs. The choice of data depends on the specific AI application and the task it aims to accomplish.
Question: What are the challenges of AI training?
AI training faces challenges such as data quality, bias, scalability, and the need for computational resources. Ensuring high-quality and diverse training data, addressing bias in the data, and managing the computational demands are some of the key obstacles in AI training.
Question: What is the role of deep learning in AI training?
Deep learning is a subfield of machine learning that uses neural networks with multiple layers to extract hierarchical representations from data. Deep learning has revolutionized AI training by enabling models to automatically learn higher-level features and handle complex tasks.
Question: How long does AI training take?
The duration of AI training depends on several factors, including the complexity of the task, the volume and quality of data, the computational resources available, and the efficiency of the training algorithms. Training can range from hours to days or even weeks for more demanding applications.
Question: Can AI models be trained on personal devices?
Training AI models on personal devices can be challenging due to the computational requirements and memory constraints. While some lightweight models can be trained on personal devices, more complex models often require high-performance hardware or cloud-based training infrastructure.
Question: How do AI models continue to learn after training?
After the initial training, AI models can continue to learn by employing techniques such as transfer learning and online learning. Transfer learning allows models to leverage their knowledge from previous tasks and adapt it to new tasks. Online learning enables models to incrementally learn from newly available data in real-time scenarios.
Question: What industries benefit from AI training?
AI training has applications in various industries, including healthcare, finance, manufacturing, transportation, and entertainment. It can help in areas such as medical diagnosis, fraud detection, quality control, route optimization, and personalized recommendations, among others.